An Improved Deep Learning Network Based Defect Detection Algorithm for Lithium-ion Battery Pole Chip
2023 8th International Conference on Cloud Computing and Big Data Analytics (ICCCBDA)(2023)
摘要
In order to meet the needs of the detection accuracy and speed of lithium-ion battery chip defects, this article proposes an improved algorithm based on deep learning YOLO5. First, introduce the coordinate attention mechanism (CA) to strengthen the study of the characteristics of the image area of the lithium-ion polar tablet so that the model focuses on the extraction of the characteristics of the polar defects and weakens the effects of complex background on the test results. Further, improve the accuracy of polar defect detection, and use the CIoU loss function to replace the GIoU function, so that the regression process focuses more on high-quality anchor frames and improves the convergence speed, regression accuracy, and robustness of the model. Based on the above experimental results, the two improvements were jointly added to the YOLOv5 model, the mAP was increased by 1.48%, and the detection speed FPS was increased by 2 f/s
-1
.The YOLOv5-CC algorithm proposed in this article is a lithium-ion with both detection accuracy and practicality. Battery pole defect detection algorithm has high application value.
更多查看译文
关键词
lithium-ion battery,defect detection,attention mechanism,loss function,yolo5
AI 理解论文
溯源树
样例
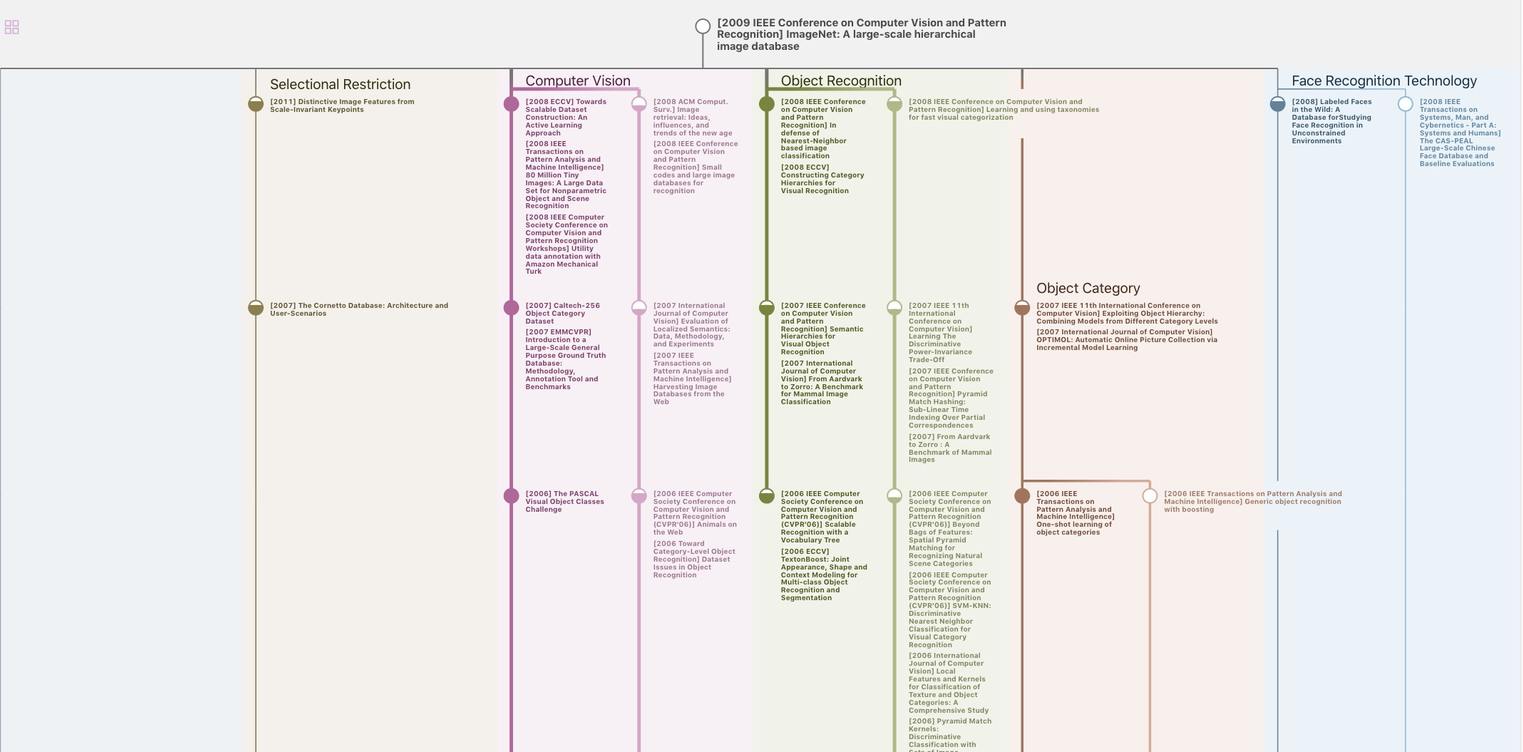
生成溯源树,研究论文发展脉络
Chat Paper
正在生成论文摘要