P-38 Using a machine learning approach to study host-microbiome interactions in early-onset colorectal adenocarcinoma
Annals of Oncology(2023)
摘要
Early-onset colorectal cancer (eoCRC) is increasing in incidence. Given that the metabolome captures both the host and microbiome metabolic correlations with cancer, we hypothesize that classifiers based on the microbiome and metabolome features could highlight unique host-microbiome associations in eoCRC and average-onset CRC (aoCRC). Patients with stage I-IV CRC were identified from prospective biobanks and categorized based on age 60 years (aoCRC) at diagnosis. Untargeted plasma metabolomic profiling was performed using GC-TOF mass spectrometry. Microbiome analysis using 16S rRNA gene amplicon sequencing was performed on fresh frozen tissue from the primary tumor. Microbiome and untargeted metabolomics data from eoCRC vs aoCRC cohorts were used to construct a robust machine-learning classifier model using DIABLO (Data Integration Analysis for Biomarker discovery using Latent variable approaches for Omics studies). The number of components was selected using Mahalanobis distance. The final model was tuned to determine optimal parameters using leave-one-out cross-validation scores. Feature analysis was performed using the Block Rank algorithm. Features were ranked based on the stability metric, defined as a fraction indicating the number of models in the performance test that selected this feature when being fitted. The study population comprised of 64 CRC patients (20 eoCRC and 44 aoCRC). The majority were male - 60% for eoCRC vs 59% aoCRC, and White - 100% vs 91%. The distribution of comorbidities (eoCRC vs aoCRC) was as follows - hyperlipidemia (25% vs 27%), obesity (25% vs 7%), and diabetes (5% vs 30%). Tumors were mostly left-sided (85% eoCRC vs. 68% aoCRC) and rectal primary 65% vs. 39%. Stage IV disease comprised 45% eoCRC vs 20% aoCRC. Patient characteristics between the two groups were not significantly different (p>0.05). Block Rank algorithm-based ranking revealed several unique metabolites and microbiome features contributing to the model. These included 25 metabolites and 10 microbial taxa. Circular correlation technique analysis highlighted several key associations (positive and negative) between key metabolites and microbial genera. Faecalibacterium and Eubacterium positively correlated with glutamine and hydroxybutyric acid abundance patterns, respectively. Additionally, Lactobacillus showed a negative correlation with glycerol alpha phosphate. Using an amplified sequence variant (ASV) matrix in the final model, we could further corroborate these associations at the strain level resolution. When comparing the differential features that would classify the groups, metabolomics classifier achieved an Area under the curve (AUC) of 98.2% vs. AUC 60.5% for microbiome-based vs. AUC 83% for microbiome plus metabolome-based classifiers. This study demonstrates that metabolomics and microbiome-based multi-omics analysis can distinguish host-microbiome interactions between eoCRC vs aoCRC. The model remained robust in multiple tests. The features driving the metabolomic differences require further investigation. Future steps include external validation of the classifier, comparison with age-matched controls, and mechanistic studies to establish the robustness of the model.
更多查看译文
关键词
adenocarcinoma,machine learning approach,machine learning,host-microbiome,early-onset
AI 理解论文
溯源树
样例
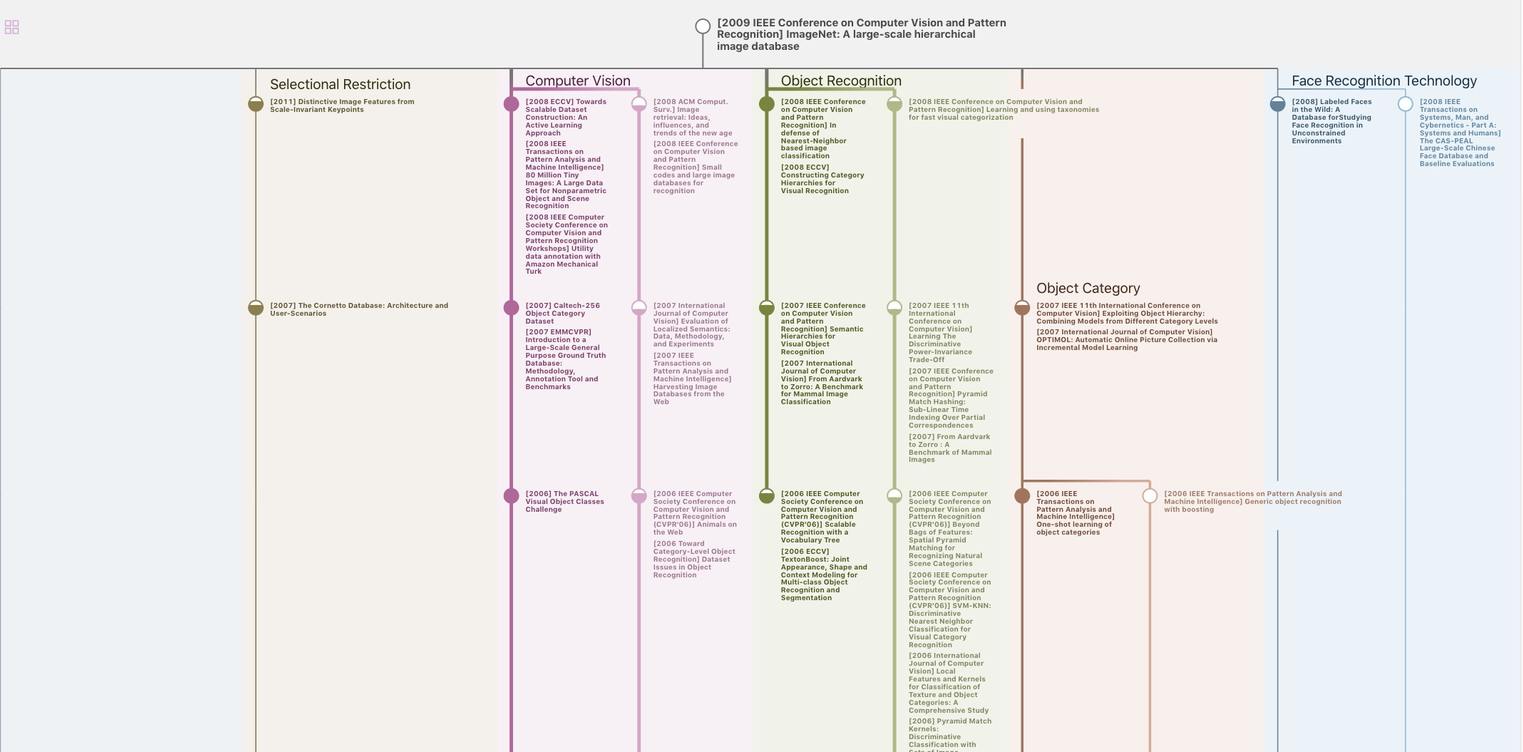
生成溯源树,研究论文发展脉络
Chat Paper
正在生成论文摘要