ConFEDE: Contrastive Feature Decomposition for Multimodal Sentiment Analysis.
Annual Meeting of the Association for Computational Linguistics (ACL)(2023)CCF A
Abstract
Multimodal Sentiment Analysis aims to predict the sentiment of video content. Recent research suggests that multimodal sentiment analysis critically depends on learning a good representation of multimodal information, which should contain both modality-invariant representations that are consistent across modalities as well as modality-specific representations. In this paper, we propose ConFEDE, a unified learning framework that jointly performs contrastive representation learning and contrastive feature decomposition to enhance representation of multimodal information. It decomposes each of the three modalities of a video sample, including text, video frames, and audio, into a similarity feature and a dissimilarity feature, which are learned by a contrastive relation centered around text. We conducted extensive experiments on CH-SIMS, MOSI and MOSEI to evaluate various state-of-the-art multimodal sentiment analysis methods. Experimental results show that ConFEDE outperforms all baselines on these datasets on a range of metrics.
MoreTranslated text
Key words
Emotion Recognition,Aspect-based Sentiment Analysis,Feature Extraction,Sentiment Analysis
PDF
View via Publisher
AI Read Science
Video&Figures
Log in,to view the remaining content
论文作者介绍
The authors of this paper include Jiuding Yang, Yakun Yu, and Di Niu from the University of Alberta, as well as Weidong Guo and Xu Yu from Tencent's Platform and Content Group. Their research areas cover content retrieval, dataset construction, image annotation, video summarization, Chinese nested named entity recognition, recommendation systems, embedding dimension search, representation learning, neural architecture search, topic models, information retrieval, language models, meta-learning, document understanding, concept mining, as well as generation and diffusion.
文献大纲
ConFEDE: Contrastive Feature Decomposition for Multimodal Sentiment Analysis
1. Introduction
- Background and challenges of Multimodal Sentiment Analysis (MSA)
- The importance of MSA
- Multimodal fusion and modality decomposition
- The proposal of ConFEDE
2. Related Work
- Multimodal Sentiment Analysis
- Contrastive representation learning
3. Method
- ConFEDE Model Architecture
- Feature Extraction
- Feature Decomposition
- Multi-task learning objective function
- Contrastive Feature Decomposition
- Contrastive loss function
- Data sampling algorithm
4. Experiments
- Datasets
- Evaluation Metrics
- Results
- CH-SIMS Dataset
- MOSI Dataset
- MOSEI Dataset
- Ablation Study
5. Conclusion
- Advantages of ConFEDE
- Limitations
关键问题
Q: What specific research methods were used in the paper?
1. Contrastive Feature Decomposition (Contrastive Feature Decomposition)
- Decompose the three modalities of video samples (text, video frames, audio) into similarity features and difference features.
- Use text similarity features as anchors to establish the contrastive relationship for all decomposed features.
2. Contrastive Learning (Contrastive Learning)
- Perform contrastive learning within and between samples to enhance the representation of multimodal information.
- Use the NT-Xent contrastive loss framework for learning.
3. Multi-task Learning (Multi-task Learning)
- Introduce multi-task prediction loss to enable the model to learn from multimodal prediction and unimodal prediction.
4. Data Sampling (Data Sampling)
- Design data samplers to retrieve similar samples based on multimodal features and labels for inter-sample contrastive learning.
Q: What are the main research findings and achievements?
1. The ConFEDE framework effectively improves the performance of multimodal sentiment analysis
- On the CH-SIMS dataset, ConFEDE outperforms all baseline methods on multiple metrics.
- On the MOSI and MOSEI datasets, ConFEDE also achieves performance superior to baseline methods.
2. Contrastive feature decomposition can effectively learn the similarity and difference between modalities
- ConFEDE can bring similar features closer together and push different features apart, thereby extracting clearer feature representations.
3. Multi-task learning can further enhance model performance
- ConFEDE can learn from multimodal prediction and unimodal prediction, further improving model performance.
Q: What are the current limitations of this research?
1. Modality Missing Issue
- ConFEDE is applicable to multimodal sentiment analysis that includes three modalities (visual, audio, text).
- When one modality is missing, the performance of the model may be affected.
2. Data Sampling Efficiency
- As the number of training samples increases, the data sampler may require more time to retrieve similar samples.
3. Model Complexity
- The ConFEDE model is relatively complex and requires more computational resources for training.
Must-Reading Tree
Example
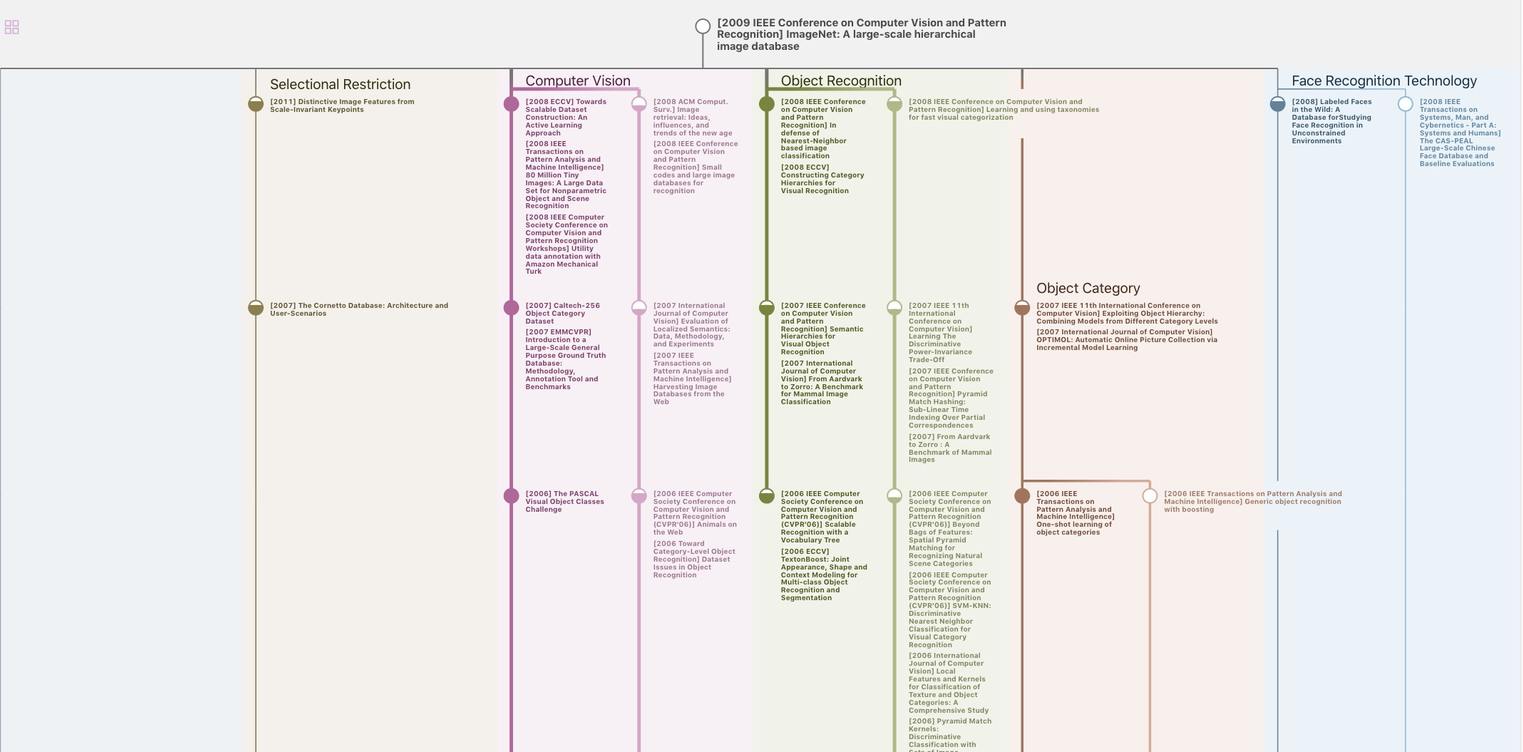
Generate MRT to find the research sequence of this paper
Related Papers
2016
被引用269 | 浏览
2018
被引用1225 | 浏览
2019
被引用69 | 浏览
2020
被引用626 | 浏览
Data Disclaimer
The page data are from open Internet sources, cooperative publishers and automatic analysis results through AI technology. We do not make any commitments and guarantees for the validity, accuracy, correctness, reliability, completeness and timeliness of the page data. If you have any questions, please contact us by email: report@aminer.cn
Chat Paper
去 AI 文献库 对话