On the influence of the quality of pseudo-labels on the self-supervised speaker verification task: a thorough analysis
2023 11th International Workshop on Biometrics and Forensics (IWBF)(2023)
摘要
One of the most widely used self-supervised (SS) speaker verification (SV) system training methods is to optimize the speaker embedding network in a discriminative fashion using clustering algorithm (CA)-driven Pseudo-Labels (PLs). Although the PL-based SS training scheme showed impressive performance, recent studies have shown that label noise can significantly impact performance. In this paper, we have explored various PLs driven by different CAs and conducted a fine-grained analysis of the relationship between the quality of the PLs and the SV performance. Experimentally, we shed light on several previously overlooked aspects of the PLs that can impact SV performance. Moreover, we could observe that the SS-SV performance is heavily dependent on multiple qualitative aspects of the CA used to generate the PLs. Furthermore, we show that SV performance can be severely degraded from overfitting the noisy PLs and that the mixup strategy can mitigate the memorization effects of label noise.
更多查看译文
关键词
Speaker verification, clustering, self-supervised speaker verification, pseudo-labels, label noise
AI 理解论文
溯源树
样例
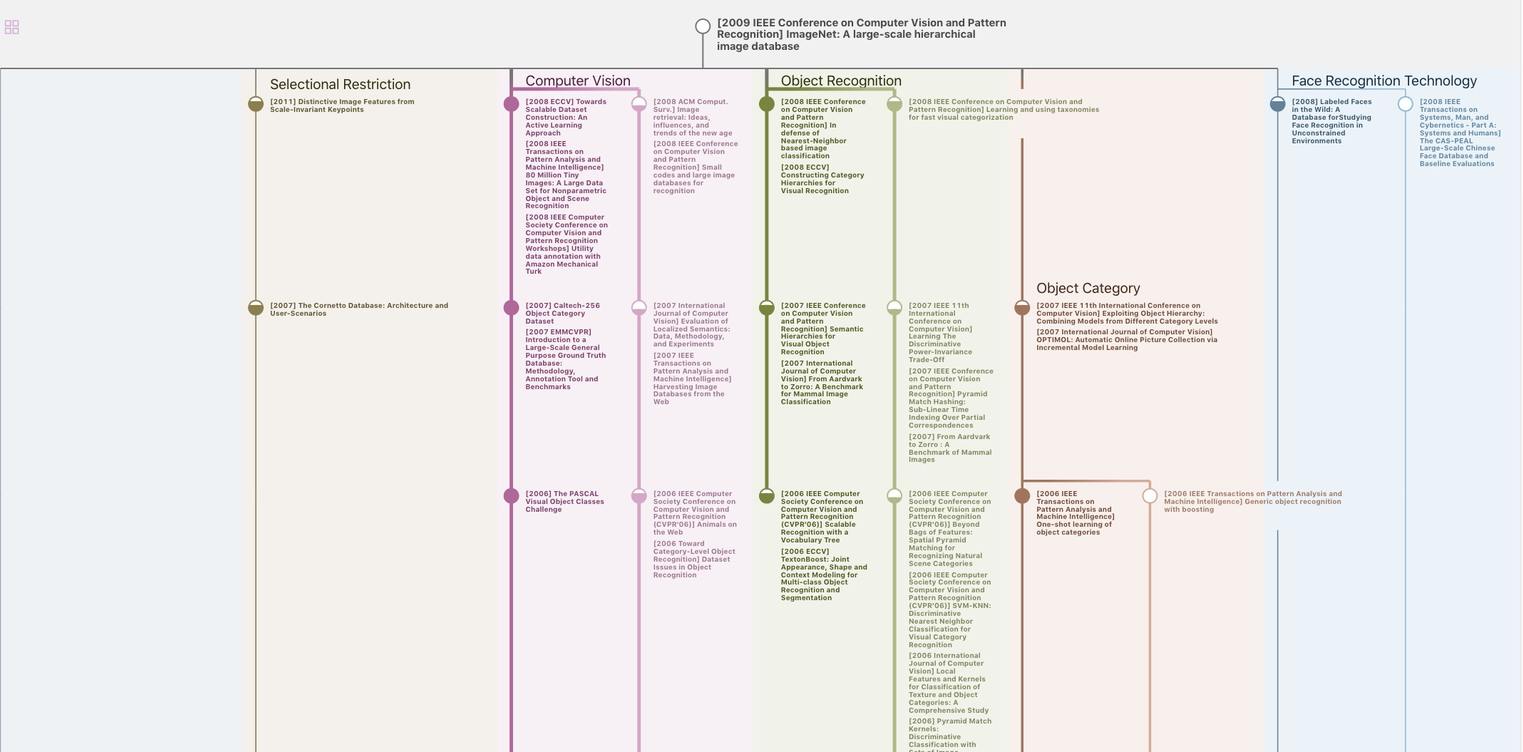
生成溯源树,研究论文发展脉络
Chat Paper
正在生成论文摘要