Multi-step prediction of carbon emissions based on a secondary decomposition framework coupled with stacking ensemble strategy
Environmental science and pollution research international(2023)
摘要
Accurate prediction of carbon emissions is vital to achieving carbon neutrality, which is one of the major goals of the global effort to protect the ecological environment. However, due to the high complexity and volatility of carbon emission time series, it is hard to forecast carbon emissions effectively. This research offers a novel decomposition-ensemble framework for multi-step prediction of short-term carbon emissions. The proposed framework involves three main steps: (i) data decomposition. A secondary decomposition method, which is a combination of empirical wavelet transform (EWT) and variational modal decomposition (VMD), is used to process the original data. (ii) Prediction and selection: ten models are used to forecast the processed data. Then, neighborhood mutual information (NMI) is used to select suitable sub-models from candidate models. (iii) Stacking ensemble: the stacking ensemble learning method is innovatively introduced to integrate the selected sub-models and output the final prediction results. For illustration and verification, the carbon emissions of three representative EU countries are used as our sample data. The empirical results show that the proposed framework is superior to other benchmark models in predictions 1, 15, and 30 steps ahead, with the mean absolute percentage error (MAPE) of the proposed framework being as low as 5.4475% in Italy dataset, 7.3159% in France dataset, and 8.6821% in Germany dataset.
更多查看译文
关键词
Carbon emission prediction,Multi-step prediction,Stacking ensemble learning,Empirical wavelet transform,Variational mode decomposition,Neighborhood mutual information
AI 理解论文
溯源树
样例
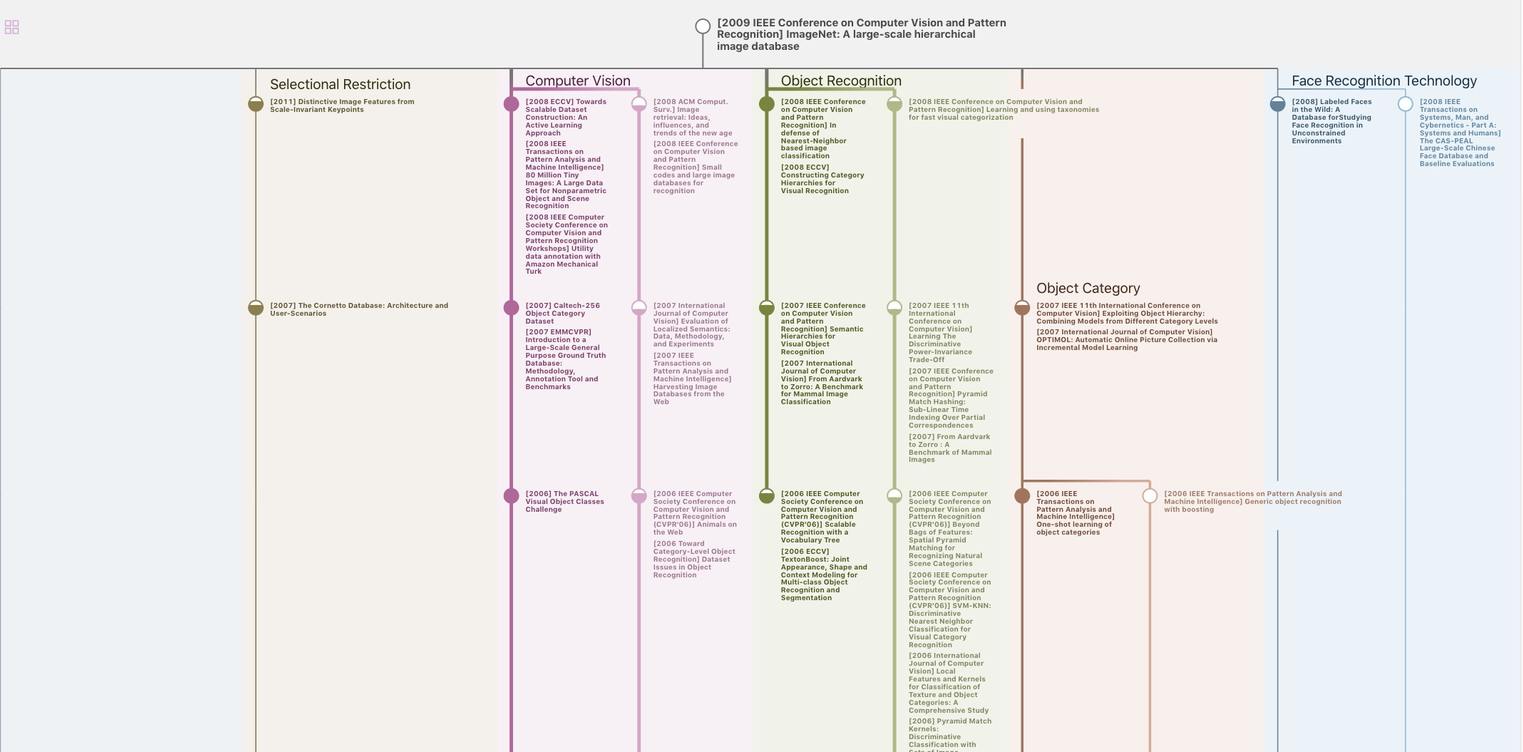
生成溯源树,研究论文发展脉络
Chat Paper
正在生成论文摘要