The curse and opportunity of heterogeneity in the pursuit of psychiatric biomarkers.
World psychiatry : official journal of the World Psychiatric Association (WPA)(2023)
摘要
Abi-Dargham et al1 provide a comprehensive overview of the state of biomarker research in psychiatry. Over the past two decades the field has witnessed a remarkable increase in studies aiming to identify biomarkers for mental disorders, facilitated by large-scale funding initiatives (e.g., by the US National Institute of Mental Health) and advancements in acquisition technologies and computational approaches. However, a clinically actionable biomarker is yet to be identified for any mental disorder. This is a timely opportunity to reflect on some of the barriers to and future directions for developing clinically useful biomarkers in psychiatry. The authors define different stages of development of biomarkers: biomarker identification, internal validation, external validation, and demonstrating clinical utility1. Most of the identified biomarkers have not moved beyond the second stage, with few biomarkers externally validated in independent datasets. The few studies that have looked at predictive performance of biomarkers in external validation samples have often used small sample sizes, which may lead to systematically inflated predictive performance estimates2. The sparsity of studies with external validation and sufficiently large sample sizes reflects the pilot-study stage of research in this area, where results can be interpreted as promising but still in their infancy. In line with the authors’ conclusions, I believe that the current approach to identifying diagnostic biomarkers (mostly case-control studies within a single mental disorder) is unlikely to lead to a biomarker-based or -assisted diagnostic framework for mental disorders. This judgment is based on the observation that most individual neuroimaging, genetic and peripheral markers have relatively small effect sizes, explaining an insufficient amount of variance of the disease phenotypes. Even when, for instance, multimodal neuroimaging measures are combined with the hope to obtain larger effects, diagnostic accuracy often remains limited3. The small effects and limited diagnostic ability of identified biological abnormalities can be explained by the fact that mental disorders are highly multi-factorial in nature, with a broad set of psychological and environmental factors contributing to vulnerability. An alternative, or perhaps complementary, explanation can be found in the imprecise measurement of our disease phenotypes. Unlike other medical fields such as oncology, that relies on histopathology for diagnosis, psychiatry is plagued with highly complex and heterogeneous presentations within and across diagnostic categories. Current diagnostic classifications incorrectly assume the existence of syndromes with a fixed set of symptoms identical for all patients. However, persons with different symptom profiles within a psychiatric diagnostic category likely differ from each other with respect to their underlying biology, severity or functioning. For example, recent large-scale studies (N>150,000) have identified a specific symptom profile of major depressive disorder (MDD), characterized by atypical energy-related symptoms. This profile is linked to a unique biological signature – most noticeably a dysregulation of the immune system4. Indeed, larger effect sizes for associations of immune and metabolic dysregulations with this specific depression symptom profile are observed than when comparing all patients with an MDD diagnosis to healthy individuals5. The common practice of aggregating diverse or even opposite symptom profiles has hampered the development of clinically use- ful diagnostic biomarkers of mental disorders. Furthermore, most identified genetic and (neuro)biological abnormalities are not specific to a single mental disorder6, making them unsuitable for differential diagnosis, which is arguably a more meaningful objective than distinguishing people with a mental disorder from those who are healthy. For example, the above-mentioned atypical energy-related symptom profile of depression linked to immunometabolic dysregulations is likely not unique to MDD, since similar symptoms as well as immune and metabolic disturbances are observed in other disorders (e.g., bipolar disorder). Therefore, incorporating transdiagnostic symptom or behavioural dimensions as the phenotype of interest has the potential to significantly advance biomarker development, albeit not necessarily facilitating a more accurate diagnosis. It is important to remind ourselves that the value of identifying biological markers of mental disorders is not restricted to whether or not they can represent useful tools for diagnostic purposes; they may also provide important clues for optimization of existing treatments or development of new interventions. For example, abnormal functional connectivity between the dorsal prefrontal cortex and subgenual anterior cingulate cortex has been suggested to be a core functional deficit in MDD. The effect size of this deficit is too small for it to serve as a diagnostic biomarker. Nonetheless, it has proven to be an important target site for repetitive transcranial magnetic stimulation (rTMS). Recent preliminary work suggests that rTMS can be optimized and individualized by targeting dorsolateral prefrontal cortex sites that display stronger negative functional connectivity with the subgenual cingulate cortex7, thereby reducing heterogeneity of rTMS treatment outcomes. Despite suggested strategies to improve diagnostic biomarker identification, I agree with the authors that a shift from a focus on diagnostic biomarkers to prognostic or predictive biomarkers would be appropriate. Identifying prognostic biomarkers of relapse/recurrence or treatment response may be a more fruitful endeavour, as they correspond more clearly to processes involved in the outcome in question (e.g., a biological treatment). Indeed, studies have shown that therapeutic outcomes are often related to pre-treatment brain differences, and that the brain changes as a result of the treatment. Moreover, grouping people based on their response to a treatment may result in more homogeneous samples than those based on a DSM diagnosis, further enhancing the likelihood of deriving clinically actionable biomarkers. Some studies are beginning to show promising candidate treatment biomarkers that have been internally and/or externally validated, including, for instance, EEG biomarkers of response to selective serotonin reuptake inhibitors in patients with MDD8, 9. Nonetheless, heterogeneity may still exist within groups of treatment responders or those who experience recurrent episodes of mental ill-health, since different underlying mechanisms may lead to the same outcome in different people (most treatments have no clear single mechanism of action). Unfortunately, most clinical trials have not been adequately powered to disentangle this within-group heterogeneity, and future larger-scale clinical trials (e.g., through clinical trial networks), data pooling initiatives of existing trial data, or more flexible trial designs are required. Another important consideration for future development of prognostic and predictive biomarkers is the extension of predictive models with time-varying predictors, facilitated by recent advancements in machine learning (e.g., recurrent neural networks). Including time-varying biomarkers could enhance predictive accuracy, given that treatment response or recurrence of mental ill-health is often not a static but a dynamic process. Very few studies to date have exploited the time-varying nature of predictor variables, although initial studies are starting to show increased prediction accuracy for treatment response when adding early changes in biomarkers to baseline predictors8. In conclusion, even though a clinically actionable biomarker is yet to be discovered, several developments – including larger-scale studies and collaborations (enabling independent validation), advances in statistical methods, and more precise phenotyping – have the potential to accelerate progress in psychiatric biomarker research in the coming years. Harnessing heterogeneity within and across our current diagnostic classifications, and within groups of treatment responders, will be key to future success of biomarkers in optimizing care for the next generation of people with mental disorders.
更多查看译文
关键词
psychiatric biomarkers,heterogeneity
AI 理解论文
溯源树
样例
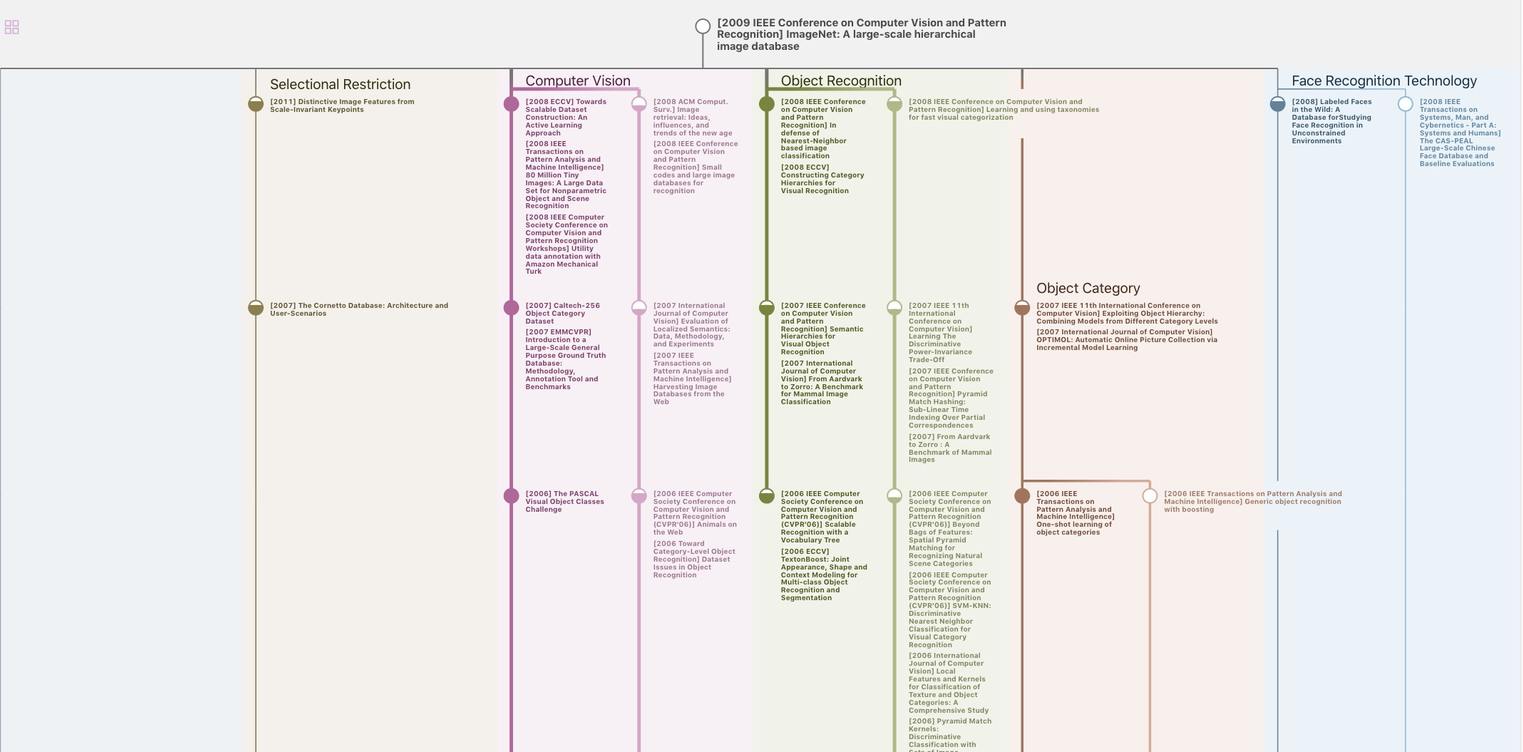
生成溯源树,研究论文发展脉络
Chat Paper
正在生成论文摘要