Predicting Protein-Peptide Interactions: Benchmarking Deep Learning Techniques and a Comparison with Focused Docking
Journal of chemical information and modeling(2023)
摘要
The accurate prediction of proteinstructures achieved by deeplearning (DL) methods is a significant milestone and has deeply impactedstructural biology. Shortly after its release, AlphaFold2 has beenevaluated for predicting protein-peptide interactions and shownto significantly outperform RoseTTAfold as well as a conventionalblind docking method: PIPER-FlexPepDock. Since then, new AlphaFold2models, trained specifically to predict multimeric assemblies, havebeen released and a new ab initio folding model OmegaFold has becomeavailable. Here, we assess docking success rates for these new DLfolding models and compare their performance with our state-of-the-art,focused peptide-docking software AutoDock CrankPep (ADCP). The evaluationis done using the same dataset and performance metric for all methods.We show that, for a set of 99 nonredundant protein-peptidecomplexes, the new AlphaFold2 model outperforms other Deep Learningapproaches and achieves remarkable docking success rates for peptides.While the docking success rate of ADCP is more modest when consideringthe top-ranking solution only, it samples correct solutions for around62% of the complexes. Interestingly, different methods succeed ondifferent complexes, and we describe a consensus docking approachusing ADCP and AlphaFold2, which achieves a remarkable 60% for thetop-ranking results and 66% for the top 5 results for this set of99 protein-peptide complexes.
更多查看译文
AI 理解论文
溯源树
样例
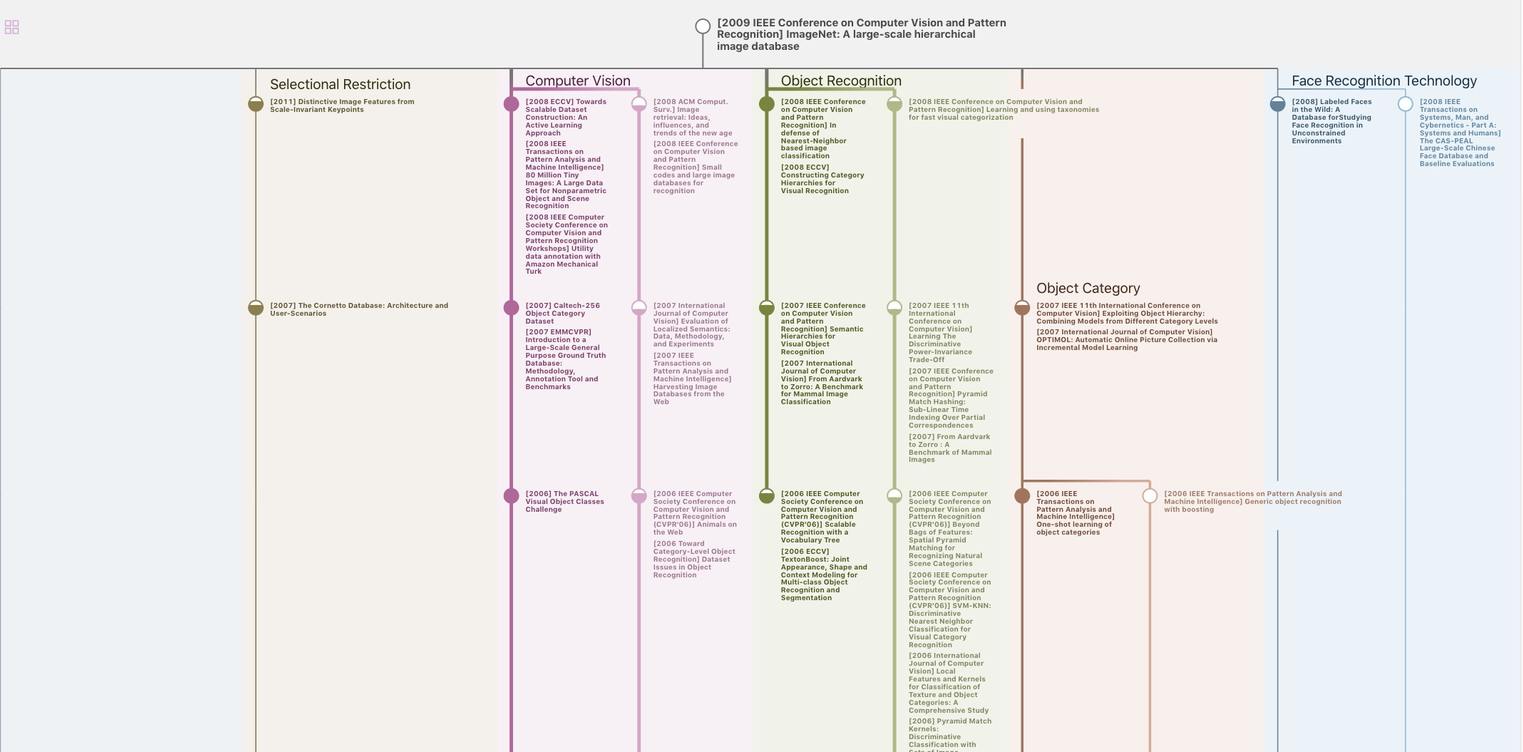
生成溯源树,研究论文发展脉络
Chat Paper
正在生成论文摘要