A multi-view co-training network for semi-supervised medical image-based prognostic prediction.
Neural networks : the official journal of the International Neural Network Society(2023)
摘要
Prognostic prediction has long been a hotspot in disease analysis and management, and the development of image-based prognostic prediction models has significant clinical implications for current personalized treatment strategies. The main challenge in prognostic prediction is to model a regression problem based on censored observations, and semi-supervised learning has the potential to play an important role in improving the utilization efficiency of censored data. However, there are yet few effective semi-supervised paradigms to be applied. In this paper, we propose a semi-supervised co-training deep neural network incorporating a support vector regression layer for survival time estimation (Co-DeepSVS) that improves the efficiency in utilizing censored data for prognostic prediction. First, we introduce a support vector regression layer in deep neural networks to deal with censored data and directly predict survival time, and more importantly to calculate the labeling confidence of each case. Then, we apply a semi-supervised multi-view co-training framework to achieve accurate prognostic prediction, where labeling confidence estimation with prior knowledge of pseudo time is conducted for each view. Experimental results demonstrate that the proposed Co-DeepSVS has a promising prognostic ability and surpasses most widely used methods on a multi-phase CT dataset. Besides, the introduction of SVR layer makes the model more robust in the presence of follow-up bias.
更多查看译文
关键词
prognostic prediction,multi-view,co-training,semi-supervised,image-based
AI 理解论文
溯源树
样例
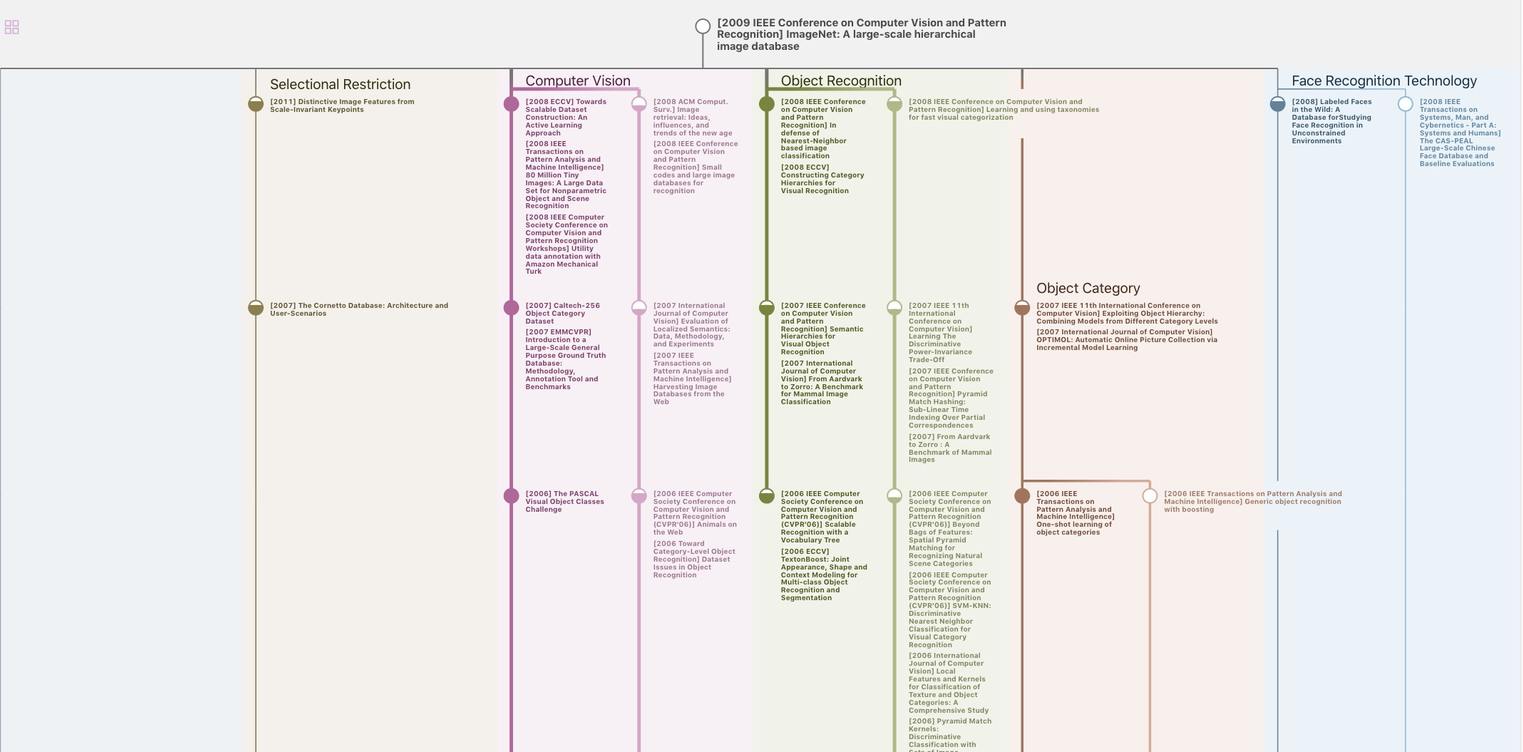
生成溯源树,研究论文发展脉络
Chat Paper
正在生成论文摘要