Predicting Immunological Risk for Stage 1 and Stage 2 Diabetes Using a 1-Week CGM Home Test, Nocturnal Glucose Increments, and Standardized Liquid Mixed Meal Breakfasts, with Classification Enhanced by Machine Learning
DIABETES TECHNOLOGY & THERAPEUTICS(2023)
摘要
Background: Predicting the risk for type 1 diabetes (T1D) is a significant challenge. We use a 1-week continuous glucose monitoring (CGM) home test to characterize differences in glycemia in at-risk healthy individuals based on autoantibody presence and develop a machine-learning technology for CGM-based islet autoantibody classification.Methods: Sixty healthy relatives of people with T1D with mean +/- standard deviation age of 23.7 +/- 10.7 years, HbA1c of 5.3% +/- 0.3%, and body mass index of 23.8 +/- 5.6 kg/m(2) with zero (n = 21), one (n = 18), and >= 2 (n = 21) autoantibodies were enrolled in an National Institutes of Health TrialNet ancillary study. Participants wore a CGM for a week and consumed three standardized liquid mixed meals (SLMM) instead of three breakfasts. Glycemic outcomes were computed from weekly, overnight (12:00-06:00), and post-SLMM CGM traces, compared across groups, and used in four supervised machine-learning autoantibody status classifiers. Classifiers were evaluated through 10-fold cross-validation using the receiver operating characteristic area under the curve (AUC-ROC) to select the best classification model.Results: Among all computed glycemia metrics, only three were different across the autoantibodies groups: percent time >180 mg/dL (T180) weekly (P = 0.04), overnight CGM incremental AUC (P = 0.005), and T180 for 75 min post-SLMM CGM traces (P = 0.004). Once overnight and post-SLMM features are incorporated in machine-learning classifiers, a linear support vector machine model achieved the best performance of classifying autoantibody positive versus autoantibody negative participants with AUC-ROC >= 0.81.Conclusion: A new technology combining machine learning with a potentially self-administered 1-week CGM home test can help improve T1D risk detection without the need to visit a hospital or use a medical laboratory.
更多查看译文
关键词
Continuous glucose monitoring,Immunological risk,Islet autoantibodies,Machine learning,Prediabetes,Type 1 diabetes
AI 理解论文
溯源树
样例
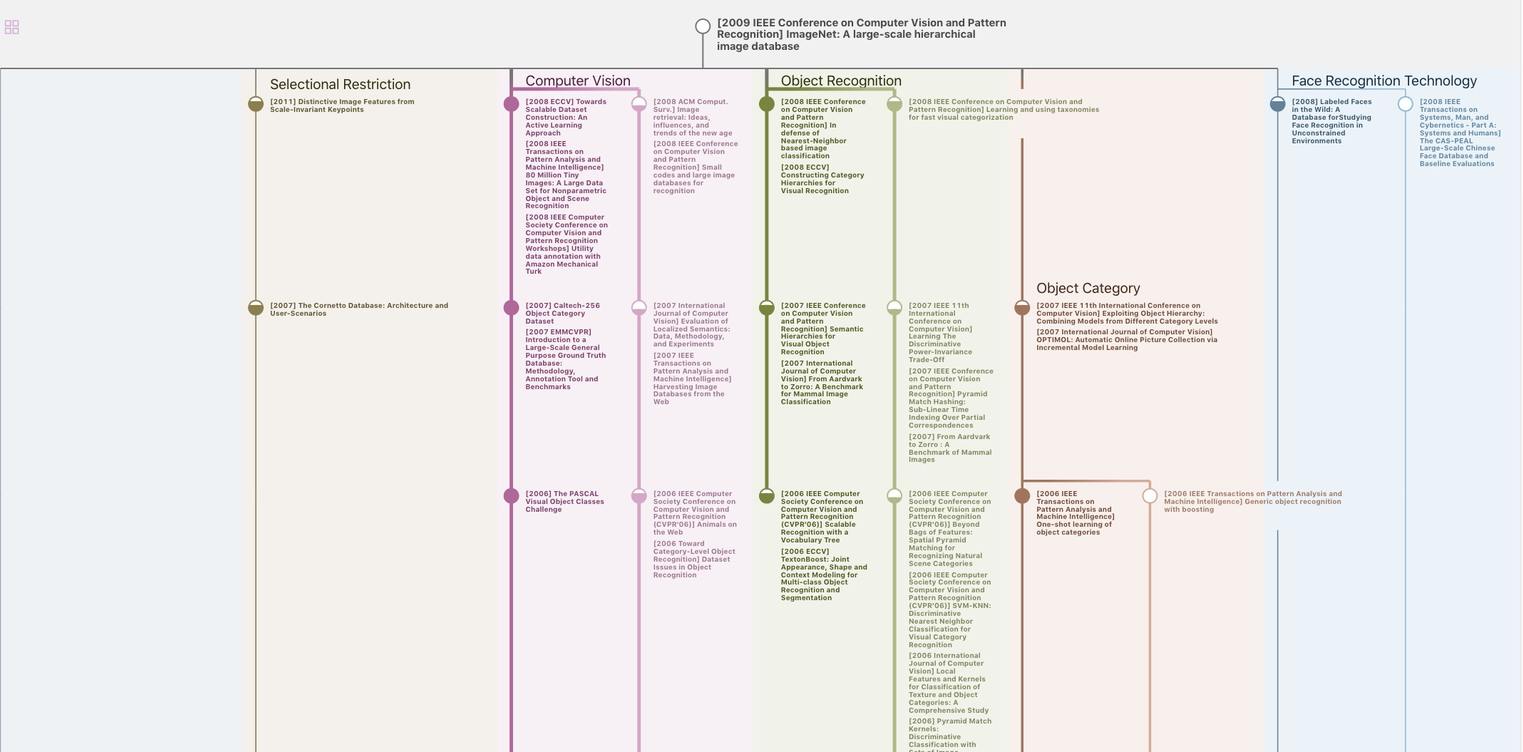
生成溯源树,研究论文发展脉络
Chat Paper
正在生成论文摘要