Local Feature Matters: Cascade Multi-scale MLP for Edge Segmentation of Medical Images.
IEEE transactions on nanobioscience(2023)
摘要
Convolution-based methods are increasingly being used in medical image segmentation tasks and have shown good performance, but there are always problems in segmenting edge parts. These methods all have the following challenges: (1) Previous methods do not highlight the relationship between foreground and background in segmented regions, which is helpful for complex segmentation edges. (2) The inductive bias of the convolutional layer leads to the fact that the extracted information is mainly the main part of the segmented area, and cannot effectively perceive complex edge changes and the aggregation of small and many segmented areas. (3) Different regions around the segmentation edge have different reference values for segmentation, and the ordering of these values is more important when the segmentation task is more complex. To address these challenges, we propose the CM-MLP framework on Multi-scale Feature Interaction (MFI) block and Axial Context Relation Encoder (ACRE) block for accurate segmentation of the edge of medical image. In the MFI block, we propose the Cascade Multi-scale MLP (Cascade MLP) to process all local information from the deeper layers of the network simultaneously, using Squeeze and Excitation in Space(SES) to process and redistribute the weights of all windows in Cascade MLP and utilize a cascade multi-scale mechanism to fuse discrete local information gradually. Then, multiple ACRE blocks cooperate with the deep super-vision mechanism to gradually explore the boundary relationship between the foreground and the background, and gradually fine-tune the edges of the medical image. The segmentation accuracy (Dice) of our proposed CM-MLP framework reaches 96.98%, 96.67%, and 83.83% on three benchmark datasets: CVC-ClinicDB dataset, sub-Kvasir dataset, and our in-house dataset, respectively, which significantly outperform the state-of-the-art method. The source code and trained models will be available at https://github.com/ProgrammerHyy/CM-MLP.
更多查看译文
关键词
MLP, medical image segmentation, semantic segmentation
AI 理解论文
溯源树
样例
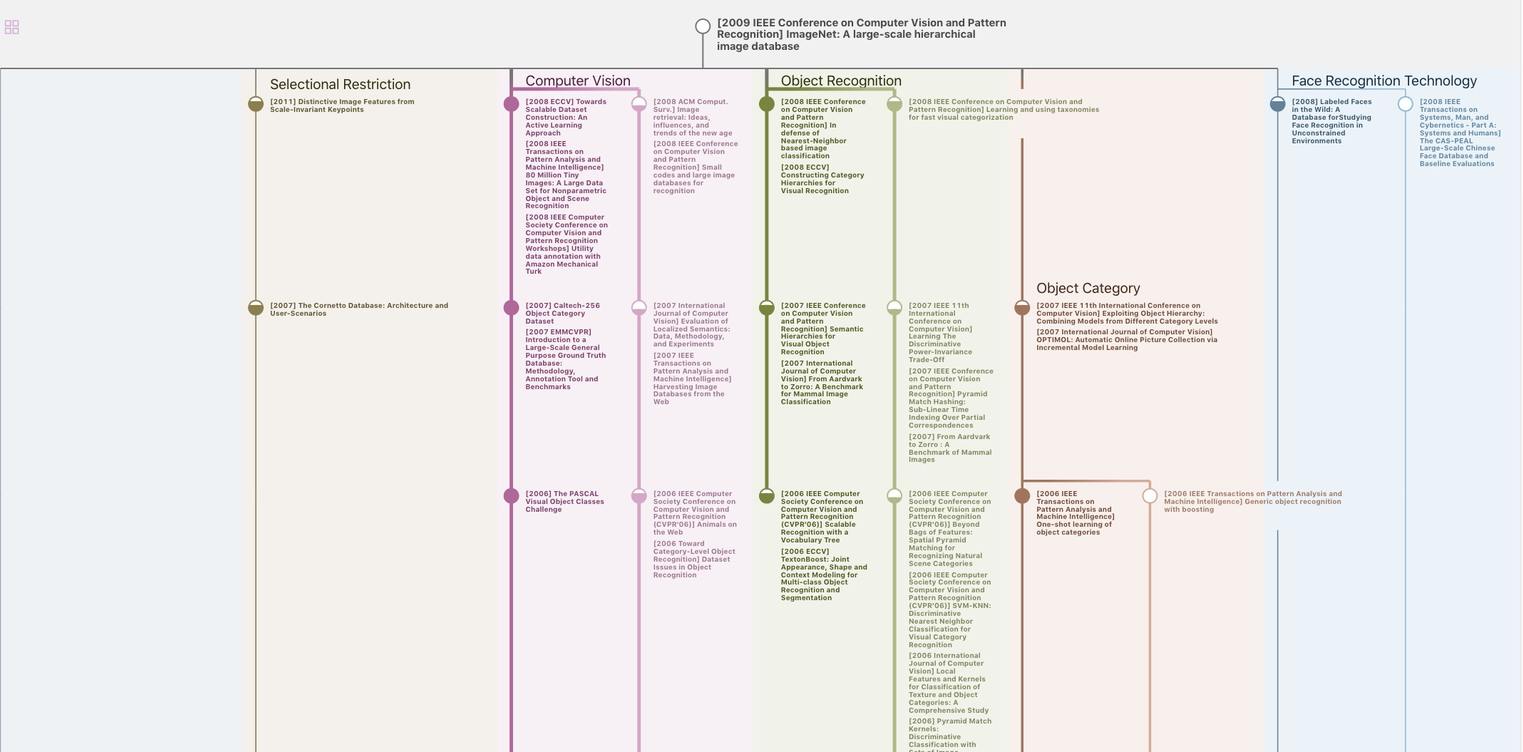
生成溯源树,研究论文发展脉络
Chat Paper
正在生成论文摘要