3D-2D Distance Maps Conversion Enhances Classification of Craniosynostosis
IEEE transactions on bio-medical engineering(2023)
Abstract
Objective: Diagnosis of craniosynostosis using photogrammetric 3D surface scans is a promising radiation-free alternative to traditional computed tomography. We propose a 3D surface scan to 2D distance map conversion enabling the usage of the first convolutional neural networks (CNNs)-based classification of craniosynostosis. Benefits of using 2D images include preserving patient anonymity, enabling data augmentation during training, and a strong under-sampling of the 3D surface with good classification performance. Methods: The proposed distance maps sample 2D images from 3D surface scans using a coordinate transformation, ray casting, and distance extraction. We introduce a CNN-based classification pipeline and compare our classifier to alternative approaches on a dataset of 496 patients. We investigate into low-resolution sampling, data augmentation, and attribution mapping. Results: Resnet18 outperformed alternative classifiers on our dataset with an F1-score of 0.964 and an accuracy of 98.4%. Data augmentation on 2D distance maps increased performance for all classifiers. Under-sampling allowed 256-fold computation reduction during ray casting while retaining an F1-score of 0.92. Attribution maps showed high amplitudes on the frontal head. Conclusion: We demonstrated a versatile mapping approach to extract a 2D distance map from the 3D head geometry increasing classification performance, enabling data augmentation during training on 2D distance maps, and the usage of CNNs. We found that low-resolution images were sufficient for a good classification performance. Significance: Photogrammetric surface scans are a suitable craniosynostosis diagnosis tool for clinical practice. Domain transfer to computed tomography seems likely and can further contribute to reducing ionizing radiation exposure for infants.
MoreTranslated text
Key words
2D conversion,classification,CNN,convolutional neural network,craniosynostosis,data augmentation,distance map,photogrammetric surface scans,resolution
AI Read Science
Must-Reading Tree
Example
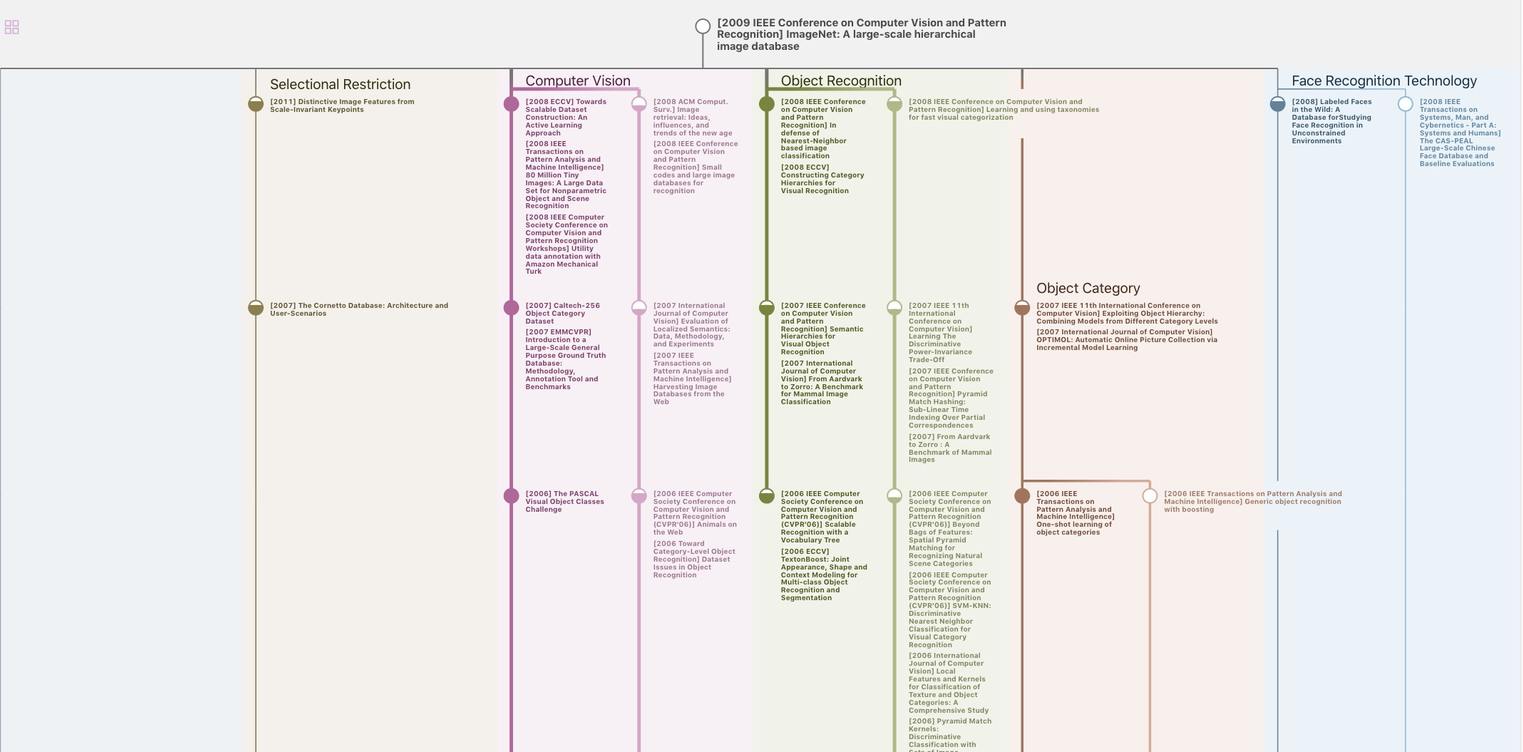
Generate MRT to find the research sequence of this paper
Chat Paper
Summary is being generated by the instructions you defined