KEBLM: Knowledge-Enhanced Biomedical Language Models
Journal of biomedical informatics(2023)
摘要
Pretrained language models (PLMs) have demonstrated strong performance on many natural language process-ing (NLP) tasks. Despite their great success, these PLMs are typically pretrained only on unstructured free texts without leveraging existing structured knowledge bases that are readily available for many domains, especially scientific domains. As a result, these PLMs may not achieve satisfactory performance on knowledge-intensive tasks such as biomedical NLP. Comprehending a complex biomedical document without domain-specific knowledge is challenging, even for humans. Inspired by this observation, we propose a general framework for incorporating various types of domain knowledge from multiple sources into biomedical PLMs. We encode domain knowledge using lightweight adapter modules, bottleneck feed-forward networks that are inserted into different locations of a backbone PLM. For each knowledge source of interest, we pretrain an adapter module to capture the knowledge in a self-supervised way. We design a wide range of self-supervised objectives to accommodate diverse types of knowledge, ranging from entity relations to description sentences. Once a set of pretrained adapters is available, we employ fusion layers to combine the knowledge encoded within these adapters for downstream tasks. Each fusion layer is a parameterized mixer of the available trained adapters that can identify and activate the most useful adapters for a given input. Our method diverges from prior work by including a knowledge consolidation phase, during which we teach the fusion layers to effectively combine knowledge from both the original PLM and newly-acquired external knowledge using a large collection of unannotated texts. After the consolidation phase, the complete knowledge-enhanced model can be fine-tuned for any downstream task of interest to achieve optimal performance. Extensive experiments on many biomedical NLP datasets show that our proposed framework consistently improves the performance of the underlying PLMs on various downstream tasks such as natural language inference, question answering, and entity linking. These results demonstrate the benefits of using multiple sources of external knowledge to enhance PLMs and the effectiveness of the framework for incorporating knowledge into PLMs. While primarily focused on the biomedical domain in this work, our framework is highly adaptable and can be easily applied to other domains, such as the bioenergy sector.
更多查看译文
关键词
Pre-trained language models,Knowledge bases,Domain knowledge
AI 理解论文
溯源树
样例
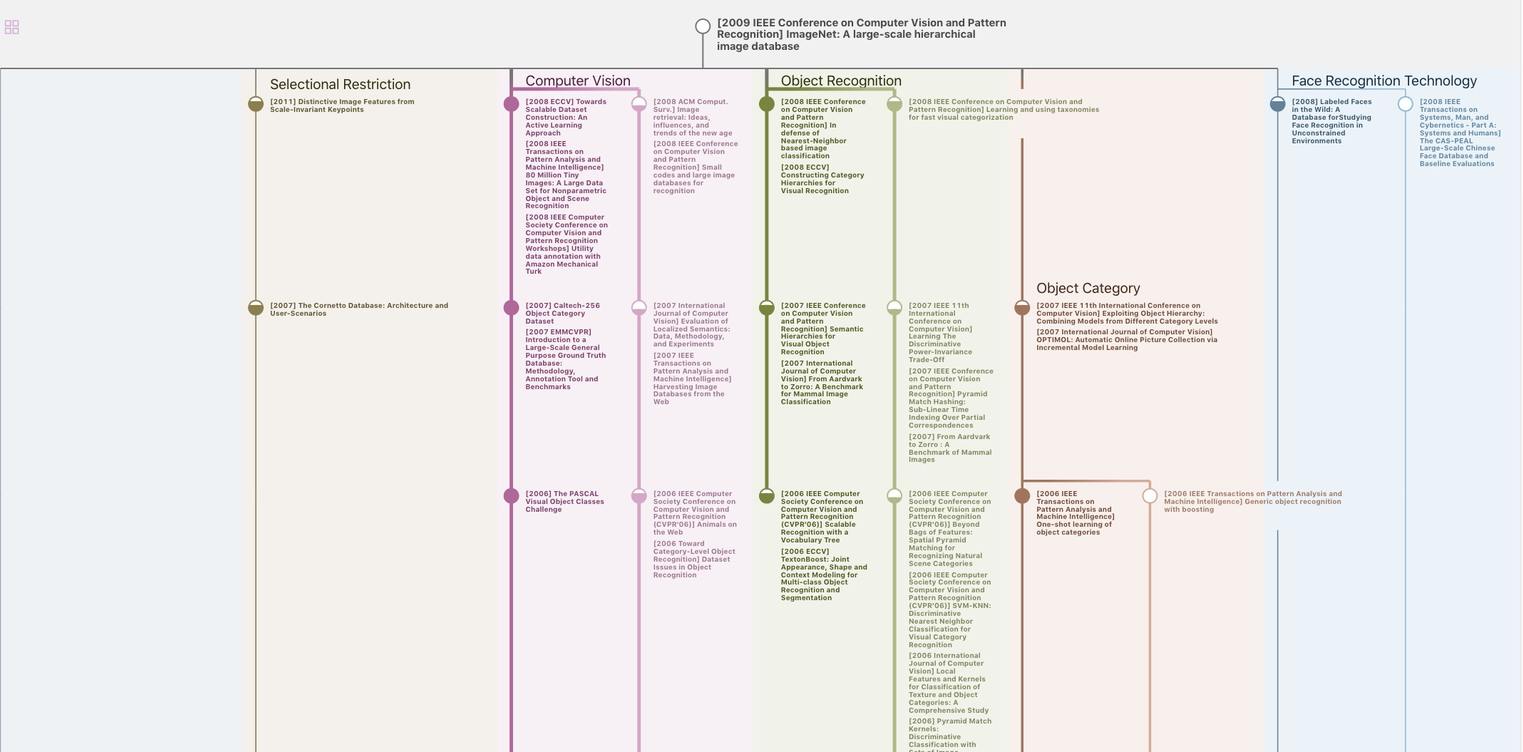
生成溯源树,研究论文发展脉络
Chat Paper
正在生成论文摘要