Detecting anomalies from liquid transfer videos in automated laboratory setting.
Frontiers in molecular biosciences(2023)
摘要
In this work, we address the problem of detecting anomalies in a certain laboratory automation setting. At first, we collect video images of liquid transfer in automated laboratory experiments. We mimic the real-world challenges of developing an anomaly detection model by considering two points. First, the size of the collected dataset is set to be relatively small compared to large-scale video datasets. Second, the dataset has a class imbalance problem where the majority of the collected videos are from abnormal events. Consequently, the existing learning-based video anomaly detection methods do not perform well. To this end, we develop a practical human-engineered feature extraction method to detect anomalies from the liquid transfer video images. Our simple yet effective method outperforms state-of-the-art anomaly detection methods with a notable margin. In particular, the proposed method provides 19% and 76% average improvement in AUC and Equal Error Rate, respectively. Our method also quantifies the anomalies and provides significant benefits for deployment in the real-world experimental setting.
更多查看译文
关键词
lab automation, video anomaly detection, action recognition, machine learning, feature extraction
AI 理解论文
溯源树
样例
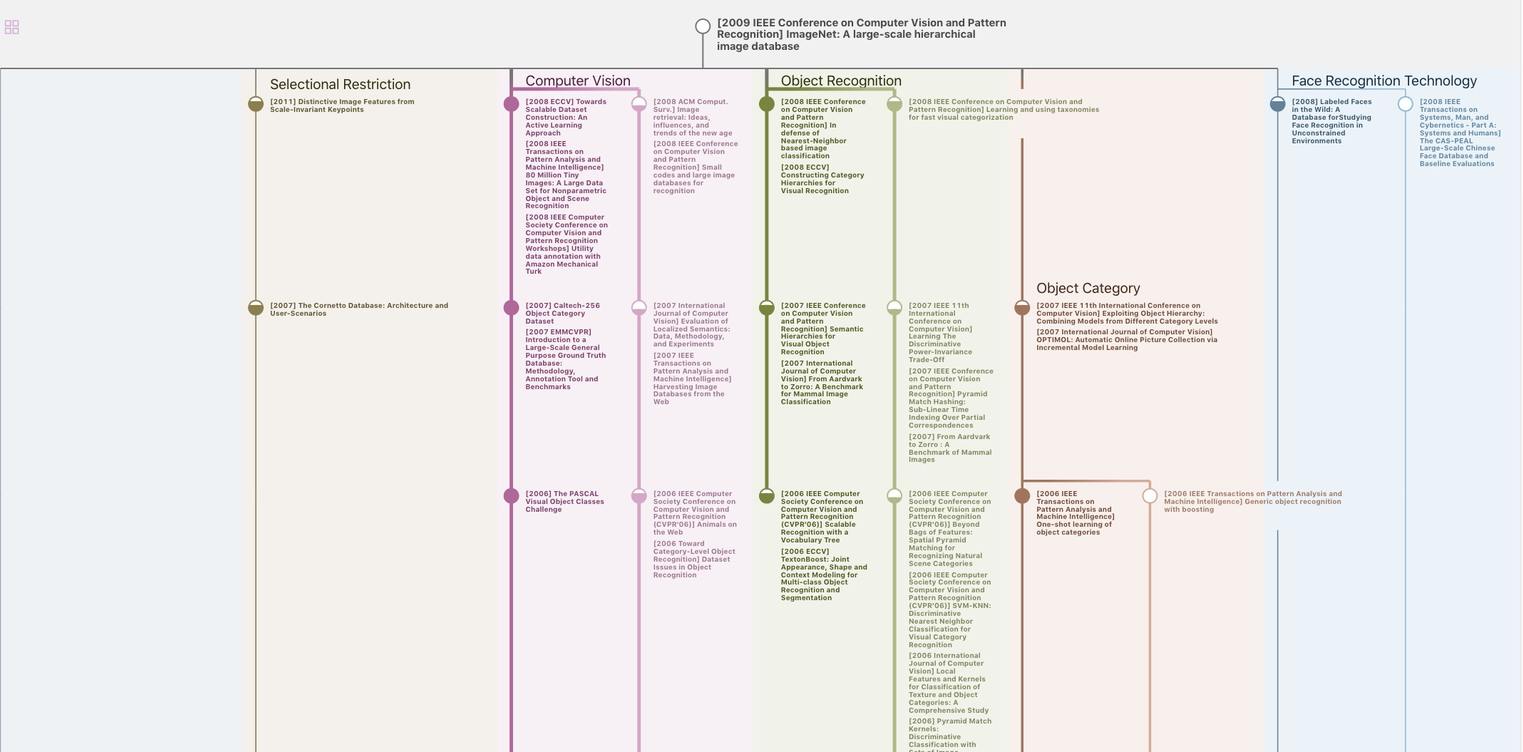
生成溯源树,研究论文发展脉络
Chat Paper
正在生成论文摘要