Mutate and observe: utilizing deep neural networks to investigate the impact of mutations on translation initiation.
Bioinformatics (Oxford, England)(2023)
摘要
MOTIVATION:The primary regulatory step for protein synthesis is translation initiation, which makes it one of the fundamental steps in the central dogma of molecular biology. In recent years, a number of approaches relying on deep neural networks (DNNs) have demonstrated superb results for predicting translation initiation sites. These state-of-the art results indicate that DNNs are indeed capable of learning complex features that are relevant to the process of translation. Unfortunately, most of those research efforts that employ DNNs only provide shallow insights into the decision-making processes of the trained models and lack highly sought-after novel biologically relevant observations.
RESULTS:By improving upon the state-of-the-art DNNs and large-scale human genomic datasets in the area of translation initiation, we propose an innovative computational methodology to get neural networks to explain what was learned from data. Our methodology, which relies on in silico point mutations, reveals that DNNs trained for translation initiation site detection correctly identify well-established biological signals relevant to translation, including (i) the importance of the Kozak sequence, (ii) the damaging consequences of ATG mutations in the 5'-untranslated region, (iii) the detrimental effect of premature stop codons in the coding region, and (iv) the relative insignificance of cytosine mutations for translation. Furthermore, we delve deeper into the Beta-globin gene and investigate various mutations that lead to the Beta thalassemia disorder. Finally, we conclude our work by laying out a number of novel observations regarding mutations and translation initiation.
AVAILABILITY AND IMPLEMENTATION:For data, models, and code, visit github.com/utkuozbulak/mutate-and-observe.
更多查看译文
关键词
mutations,deep neural networks,translation,initiation
AI 理解论文
溯源树
样例
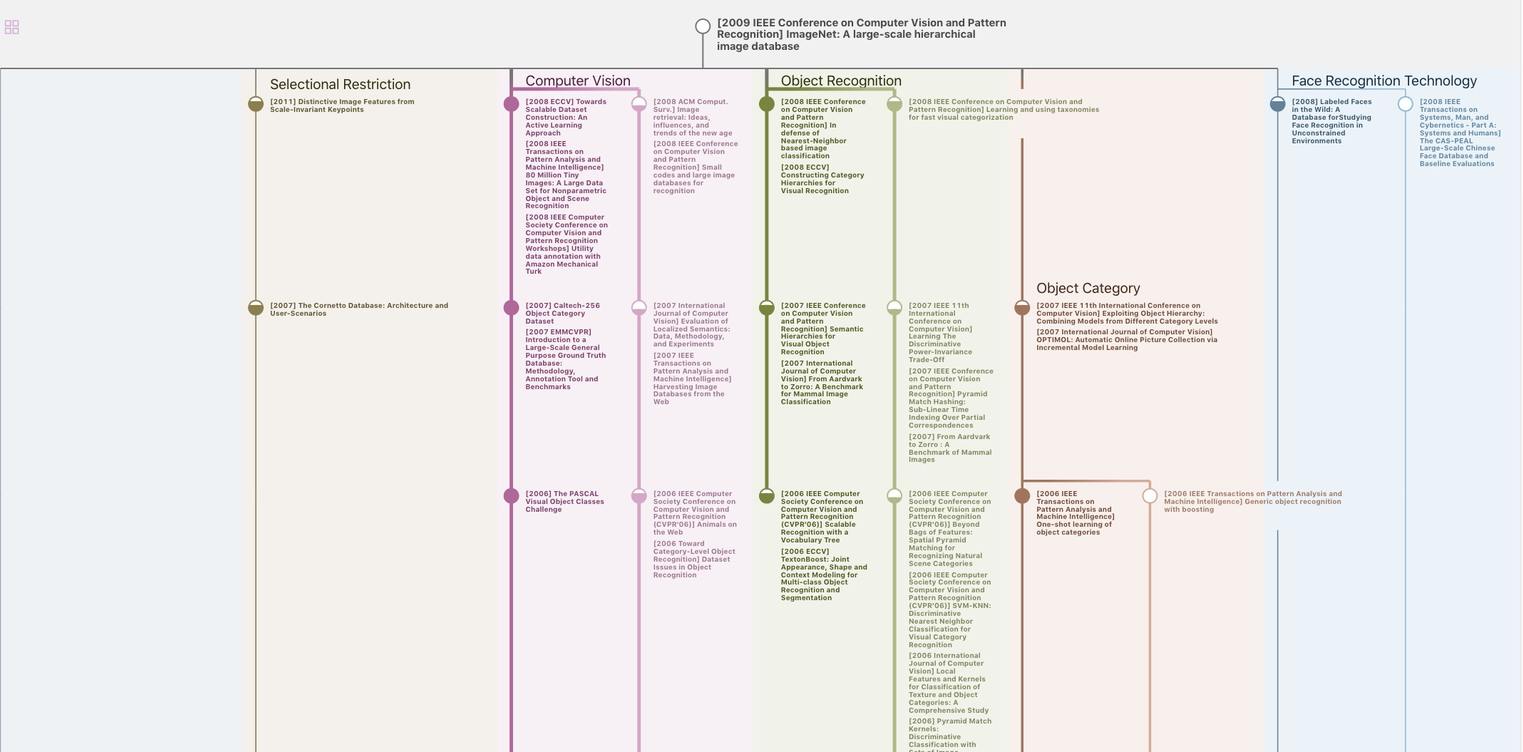
生成溯源树,研究论文发展脉络
Chat Paper
正在生成论文摘要