Machine Learning Classifier for Preoperative Prediction of Early Recurrence after Bronchial Arterial Chemoembolization Treatment in Lung Cancer Patients
ACADEMIC RADIOLOGY(2023)
Abstract
Rationale and objectives: Bronchial arterial chemoembolization (BACE) was deemed as an effective and safe approach for advanced standard treatment-ineligible/rejected lung cancer patients. However, the therapeutic outcome of BACE varies greatly and there is no reliable prognostic tool in clinical practice. This study aimed to investigate the effectiveness of radiomics features in predicting tumor recurrence after BACE treatment in lung cancer patients.Materials and methods: A total of 116 patients with pathologically confirmed lung cancer who received BACE treatment were retrospectively recruited. All patients underwent contrast-enhanced CT within 2 weeks before BACE treatment and were followed up for more than 6 months. We conducted a machine learning-based characterization of each lesion on the preoperative contrast-enhanced CT images. In the training cohort, recurrence-related radiomics features were screened by least absolute shrinkage and selection operator (LASSO) regression. Three predictive radiomics signatures were built with linear discriminant analysis (LDA), support vector machine (SVM) and logistic regression (LR) algorithms, respectively. Univariate and multivariate LR analyses were performed to select the independent clinical predictors for recurrence. The radiomics signature with best predictive performance was integrated with the clinical predictors to form a combined model, which was visualized as a nomogram. The performance of the combined model was assessed by receiver operating characteristic curve (ROC), calibration curve, and decision curve analysis (DCA).Results: Nine recurrence-related radiomics features were screened out, and three radiomics signatures (Radscore(LDA), Radscore(SVM) and Radscore(LR)) were built based on these features. Patients were classified into the low-risk and high-risk groups based on the optimal threshold of three signatures. Progression-free survival (PFS) analysis showed that patients of low-risk group achieved longer PFS than patients of high-risk group (P < 0.05). The combined model including Radscore(LDA) and independent clinical predictors (tumor size, carcinoembryonic antigen and pro-gastrin releasing peptide) achieved the best predictive performance for recurrence after BACE treatment. It yields AUCs of 0.865 and 0.867 in the training and validation cohorts, with accuracy (ACC) of 0.804 and 0.750, respectively. Calibration curves indicated that the probability of recurrence predicted by the model fits well with the actual recurrence probability. DCA showed that the radiomics nomogram was clinically useful.Conclusion: The radiomics and clinical predictors-based nomogram can predict tumor recurrence after BACE treatment effectively, which allowing oncologists to identify potential recurrence and enable better patient management and clinical decision-making.
MoreTranslated text
Key words
Bronchial arterial chemoembolization,Lung cancer,Early recurrence,Linear discriminant analysis,Support vector machine
AI Read Science
Must-Reading Tree
Example
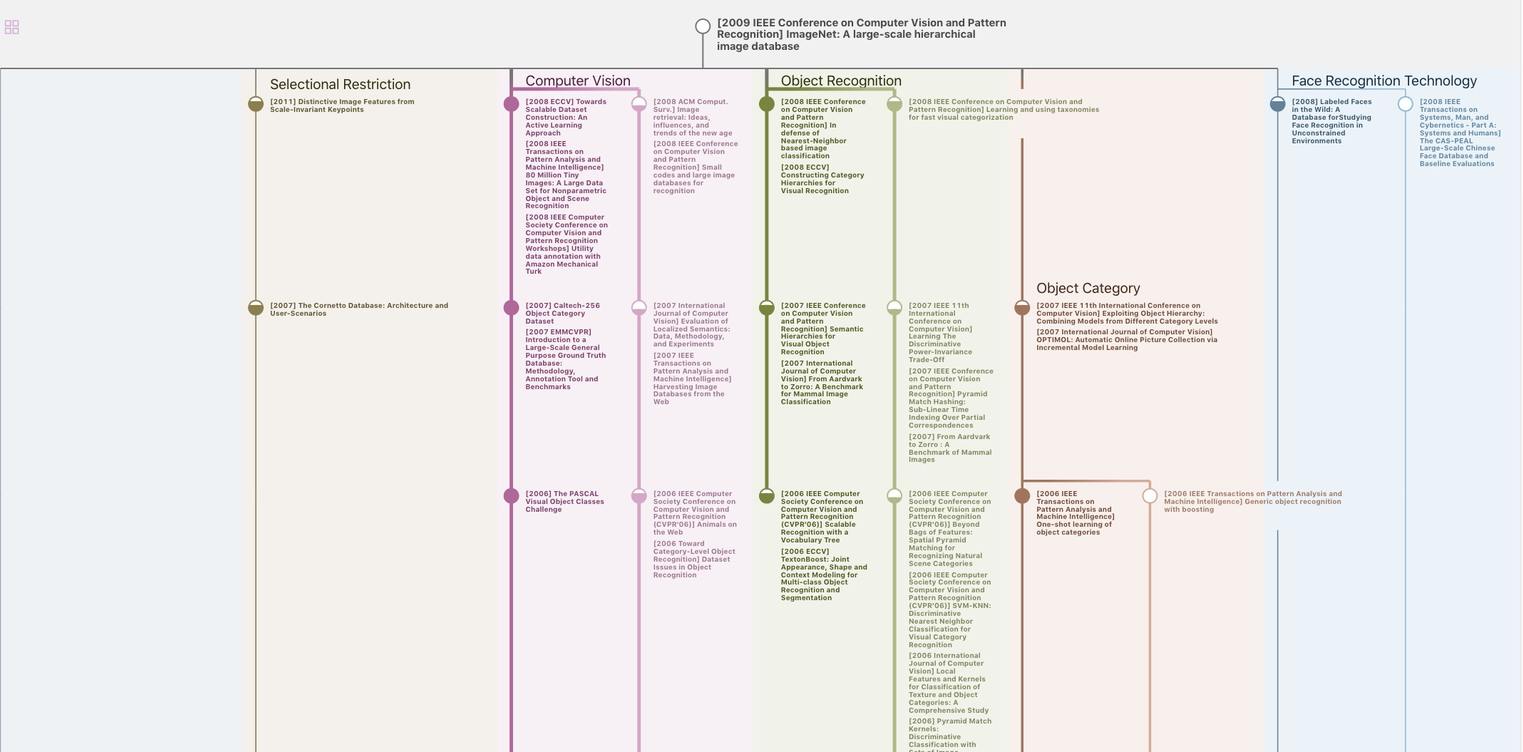
Generate MRT to find the research sequence of this paper
Chat Paper
Summary is being generated by the instructions you defined