Rapid Whole-Knee Quantification of Cartilage Using T1, T2?, and TRAFF2 Mapping With Magnetic Resonance Fingerprinting
IEEE transactions on bio-medical engineering(2023)
摘要
Objective: Quantitative Magnetic Resonance Imaging (MRI) holds great promise for the early detection of cartilage deterioration. Here, a Magnetic Resonance Fingerprinting (MRF) framework is proposed for comprehensive and rapid quantification of T-1, T-2*, and T-RAFF2 with whole-knee coverage. Methods: A MRF framework was developed to achieve quantification of Relaxation Along a Fictitious Field in the 2nd rotating frame of reference ( T-RAFF2) along with T-1 and T-2*. The proposed sequence acquires 65 measurements of 25 high-resolution slices, interleaved with 7 inversion pulses and 40 RAFF2 trains, for whole-knee quantification in a total acquisition time of 3:25 min. Comparison with reference T-1, T-2*, and T-RAFF2 methods was performed in phantom and in seven healthy subjects at 3 T. Repeatability (test-retest) with and without repositioning was also assessed. Results: Phantom measurements resulted in good agreement between MRF and the reference with mean biases of -54, 2, and 5 ms for T-1, T-2*, and T-RAFF2, respectively. Complete characterization of the whole-knee cartilage was achieved for all subjects, and, for the femoral and tibial compartments, a good agreement between MRF and reference measurements was obtained. Across all subjects, the proposed MRF method yielded acceptable repeatability without repositioning ( R-2 >= 0.94) and with repositioning ( R-2 >= 0.57) for T-1, T-2*, and T-RAFF2. Significance: The short scan time combined with the whole-knee coverage makes the proposed MRF framework a promising candidate for the early assessment of cartilage degeneration with quantitative MRI, but further research may be warranted to improve repeatability after repositioning and assess clinical value in patients.
更多查看译文
关键词
Cartilage, magnetic resonance fingerprinting, quantitative MRI, RAFF mapping, T(1)mapping, T-2*mapping, whole-knee
AI 理解论文
溯源树
样例
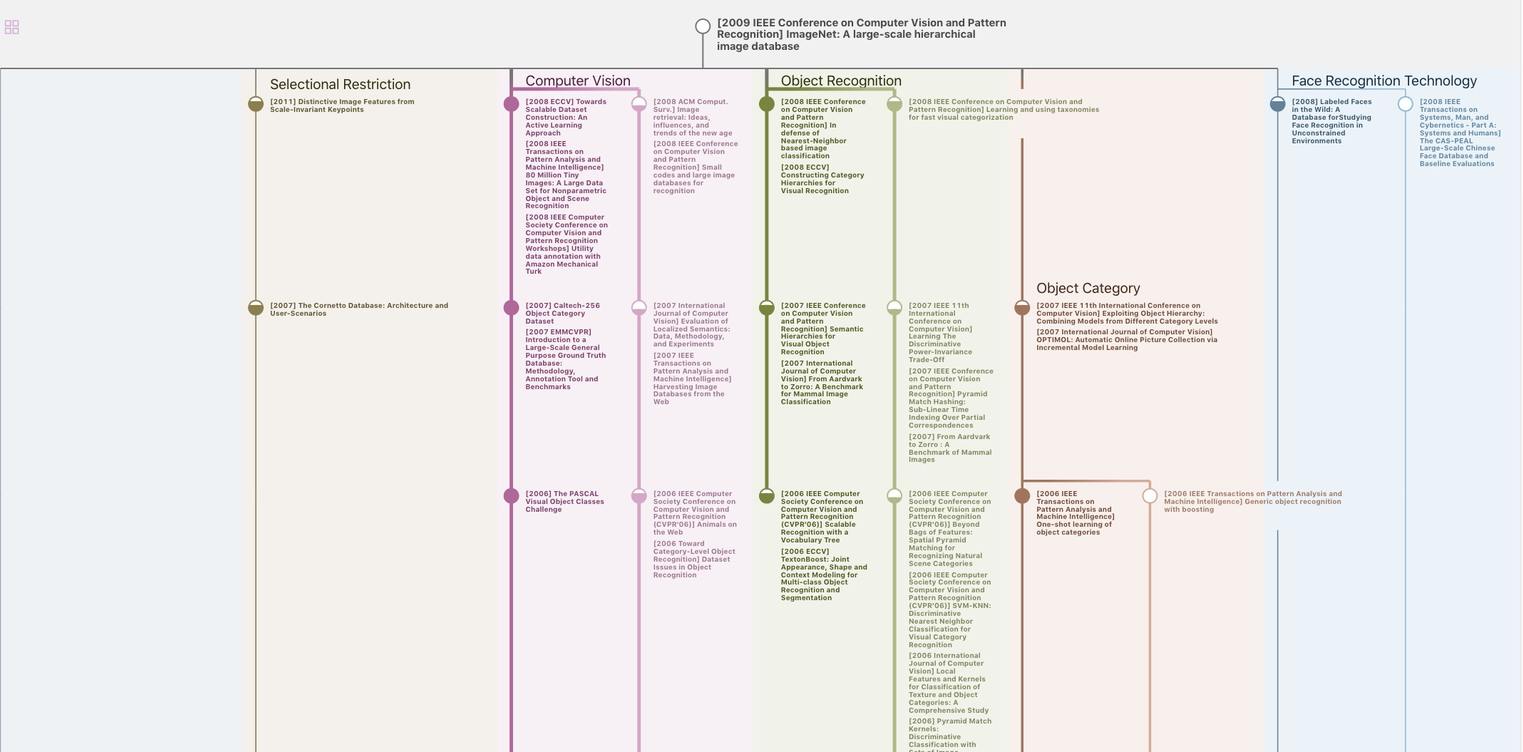
生成溯源树,研究论文发展脉络
Chat Paper
正在生成论文摘要