Quantum Chemistry-Machine Learning Approach for Predicting Properties of Lewis Acid-Lewis Base Adducts
ACS omega(2023)
摘要
Synthetic design allowing predictive control of chargetransferand other optoelectronic properties of Lewis acid adducts remainselusive. This challenge must be addressed through complementary methodscombining experimental with computational insights from first principles. Ab initio calculations for optoelectronic properties canbe computationally expensive and less straightforward than those sufficientfor simple ground-state properties, especially for adducts of largeconjugated molecules and Lewis acids. In this contribution, we showthat machine learning (ML) can accurately predict density functionaltheory (DFT)-calculated charge transfer and even properties associatedwith excited states of adducts from readily obtained molecular descriptors.Seven ML models, built from a dataset of over 1000 adducts, show exceptionalperformance in predicting charge transfer and other optoelectronicproperties with a Pearson correlation coefficient of up to 0.99. Moreimportantly, the influence of each molecular descriptor on predictedproperties can be quantitatively evaluated from ML models. This contributesto the optimization of a priori design of Lewis adducts for futureapplications, especially in organic electronics.
更多查看译文
关键词
lewis base adducts,lewis acid–lewis,predicting properties,quantum
AI 理解论文
溯源树
样例
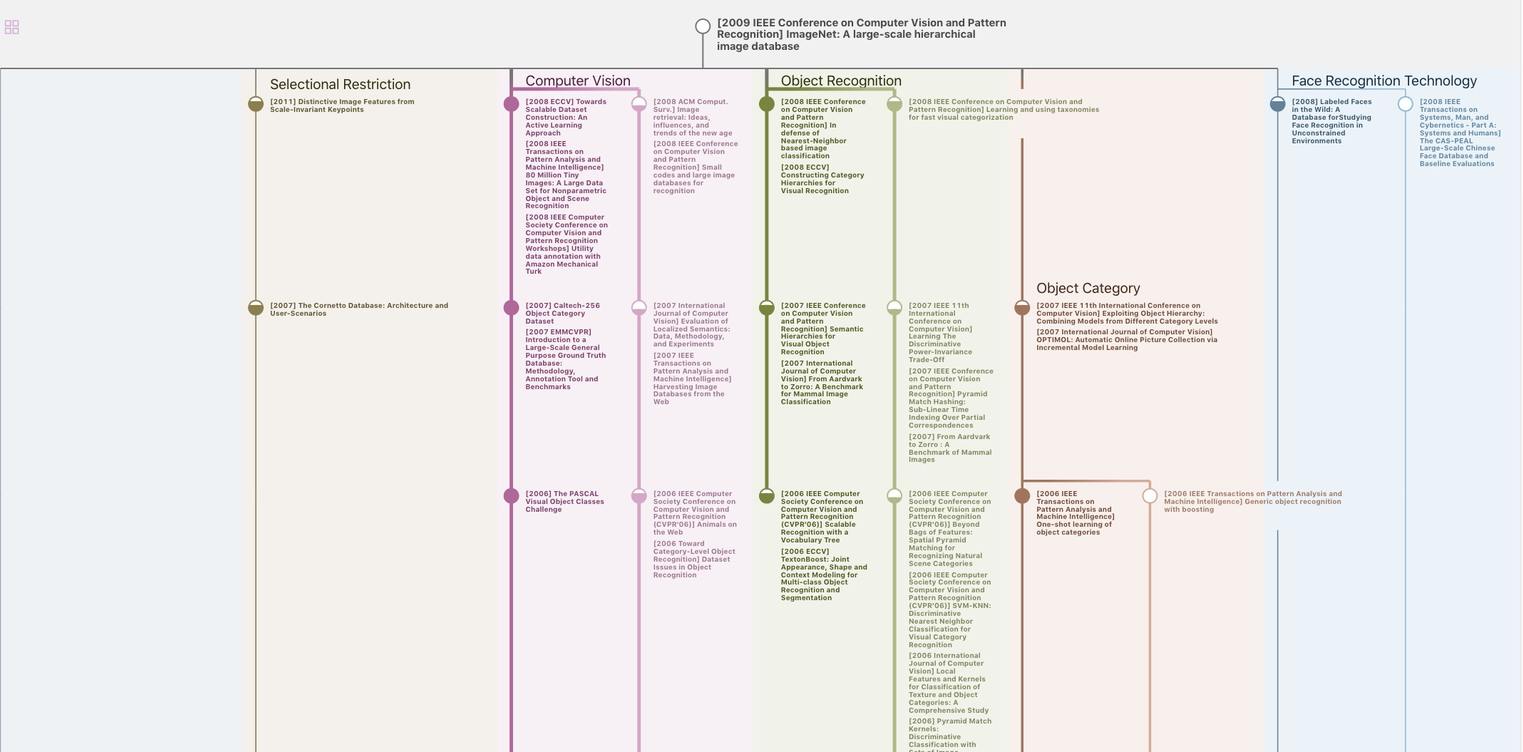
生成溯源树,研究论文发展脉络
Chat Paper
正在生成论文摘要