Evaluation of sequence-based predictors for phase-separating protein.
Briefings in bioinformatics(2023)
摘要
Liquid-liquid phase separation (LLPS) of proteins and nucleic acids underlies the formation of biomolecular condensates in cell. Dysregulation of protein LLPS is closely implicated in a range of intractable diseases. A variety of tools for predicting phase-separating proteins (PSPs) have been developed with the increasing experimental data accumulated and several related databases released. Comparing their performance directly can be challenging due to they were built on different algorithms and datasets. In this study, we evaluate eleven available PSPs predictors using negative testing datasets, including folded proteins, the human proteome, and non-PSPs under near physiological conditions, based on our recently updated LLPSDB v2.0 database. Our results show that the new generation predictors FuzDrop, DeePhase and PSPredictor perform better on folded proteins as a negative test set, while LLPhyScore outperforms other tools on the human proteome. However, none of the predictors could accurately identify experimentally verified non-PSPs. Furthermore, the correlation between predicted scores and experimentally measured saturation concentrations of protein A1-LCD and its mutants suggests that, these predictors could not consistently predict the protein LLPS propensity rationally. Further investigation with more diverse sequences for training, as well as considering features such as refined sequence pattern characterization that comprehensively reflects molecular physiochemical interactions, may improve the performance of PSPs prediction.
更多查看译文
关键词
Liquid-liquid phase separation, phase separating protein, prediction, evaluation
AI 理解论文
溯源树
样例
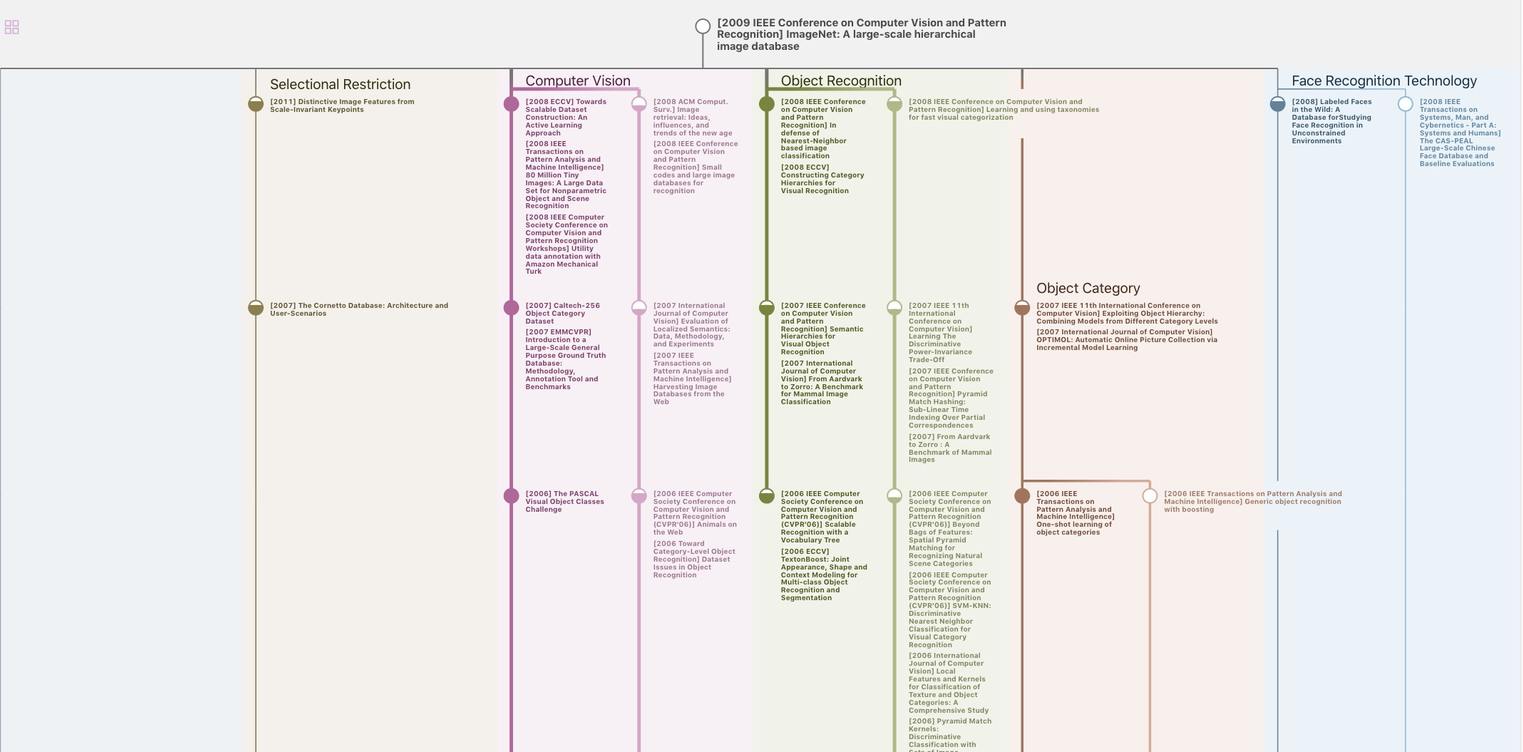
生成溯源树,研究论文发展脉络
Chat Paper
正在生成论文摘要