An integrated finite element method and machine learning algorithm for brain morphology prediction.
Cerebral cortex (New York, N.Y. : 1991)(2023)
摘要
The human brain development experiences a complex evolving cortical folding from a smooth surface to a convoluted ensemble of folds. Computational modeling of brain development has played an essential role in better understanding the process of cortical folding, but still leaves many questions to be answered. A major challenge faced by computational models is how to create massive brain developmental simulations with affordable computational sources to complement neuroimaging data and provide reliable predictions for brain folding. In this study, we leveraged the power of machine learning in data augmentation and prediction to develop a machine-learning-based finite element surrogate model to expedite brain computational simulations, predict brain folding morphology, and explore the underlying folding mechanism. To do so, massive finite element method (FEM) mechanical models were run to simulate brain development using the predefined brain patch growth models with adjustable surface curvature. Then, a GAN-based machine learning model was trained and validated with these produced computational data to predict brain folding morphology given a predefined initial configuration. The results indicate that the machine learning models can predict the complex morphology of folding patterns, including 3-hinge gyral folds. The close agreement between the folding patterns observed in FEM results and those predicted by machine learning models validate the feasibility of the proposed approach, offering a promising avenue to predict the brain development with given fetal brain configurations.
更多查看译文
关键词
brain development, cortical folding, computational modeling, machine learning, surrogate model
AI 理解论文
溯源树
样例
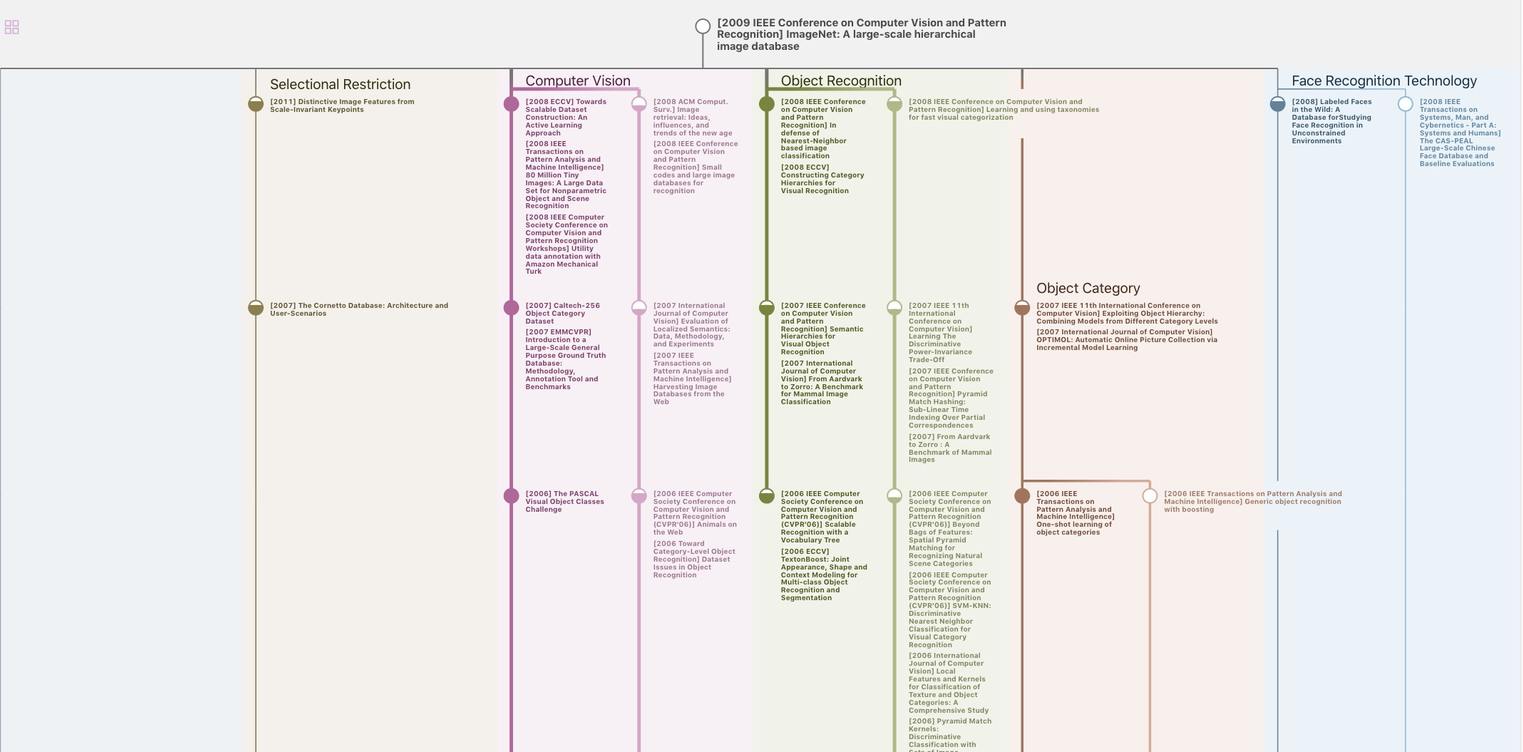
生成溯源树,研究论文发展脉络
Chat Paper
正在生成论文摘要