Balancing Memorization and Generalization in RNNs for High Performance Brain-Machine Interfaces.
bioRxiv : the preprint server for biology(2023)
摘要
Brain-machine interfaces (BMIs) can restore motor function to people with paralysis but are currently limited by the accuracy of real-time decoding algorithms. Recurrent neural networks (RNNs) using modern training techniques have shown promise in accurately predicting movements from neural signals but have yet to be rigorously evaluated against other decoding algorithms in a closed-loop setting. Here we compared RNNs to other neural network architectures in real-time, continuous decoding of finger movements using intracortical signals from nonhuman primates. Across one and two finger online tasks, LSTMs (a type of RNN) outperformed convolutional and transformer-based neural networks, averaging 18% higher throughput than the convolution network. On simplified tasks with a reduced movement set, RNN decoders were allowed to memorize movement patterns and matched able-bodied control. Performance gradually dropped as the number of distinct movements increased but did not go below fully continuous decoder performance. Finally, in a two-finger task where one degree-of-freedom had poor input signals, we recovered functional control using RNNs trained to act both like a movement classifier and continuous decoder. Our results suggest that RNNs can enable functional real-time BMI control by learning and generating accurate movement patterns.
更多查看译文
关键词
rnns,memorization,brain-machine
AI 理解论文
溯源树
样例
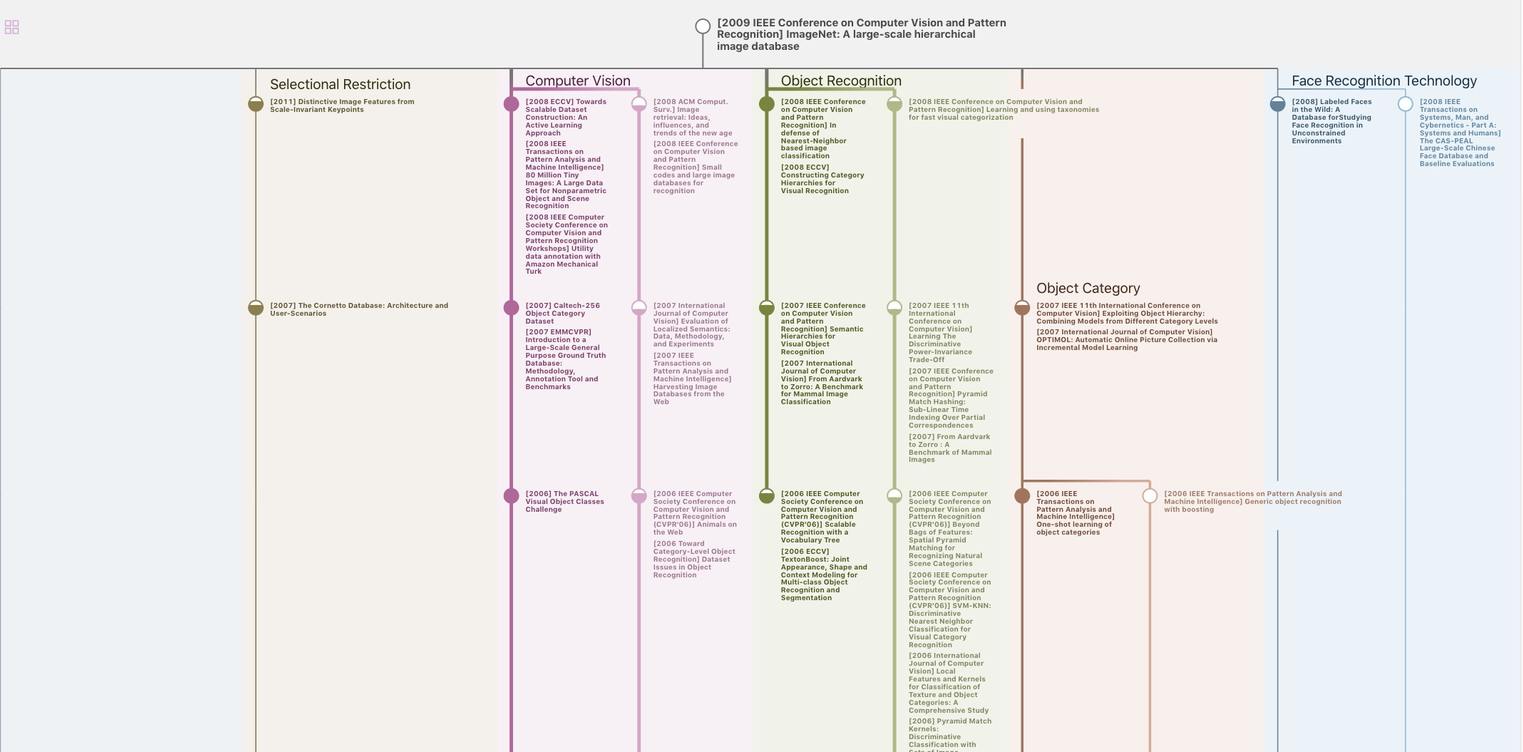
生成溯源树,研究论文发展脉络
Chat Paper
正在生成论文摘要