Empowering drug off-target discovery with metabolic and structural analysis
Nature Communications(2023)
摘要
Elucidating intracellular drug targets is a difficult problem. While machine learning analysis of omics data has been a promising approach, going from large-scale trends to specific targets remains a challenge. Here, we develop a hierarchic workflow to focus on specific targets based on analysis of metabolomics data and growth rescue experiments. We deploy this framework to understand the intracellular molecular interactions of the multi-valent dihydrofolate reductase-targeting antibiotic compound CD15-3. We analyse global metabolomics data utilizing machine learning, metabolic modelling, and protein structural similarity to prioritize candidate drug targets. Overexpression and in vitro activity assays confirm one of the predicted candidates, HPPK (folK), as a CD15-3 off-target. This study demonstrates how established machine learning methods can be combined with mechanistic analyses to improve the resolution of drug target finding workflows for discovering off-targets of a metabolic inhibitor. The authors present a workflow integrating metabolic perturbations with protein structural analysis to identify drug off-targets, demonstrating how combining machine learning methods with mechanistic analyses can benefit off-target identification.
更多查看译文
关键词
metabolic,drug,discovery,structural analysis,off-target
AI 理解论文
溯源树
样例
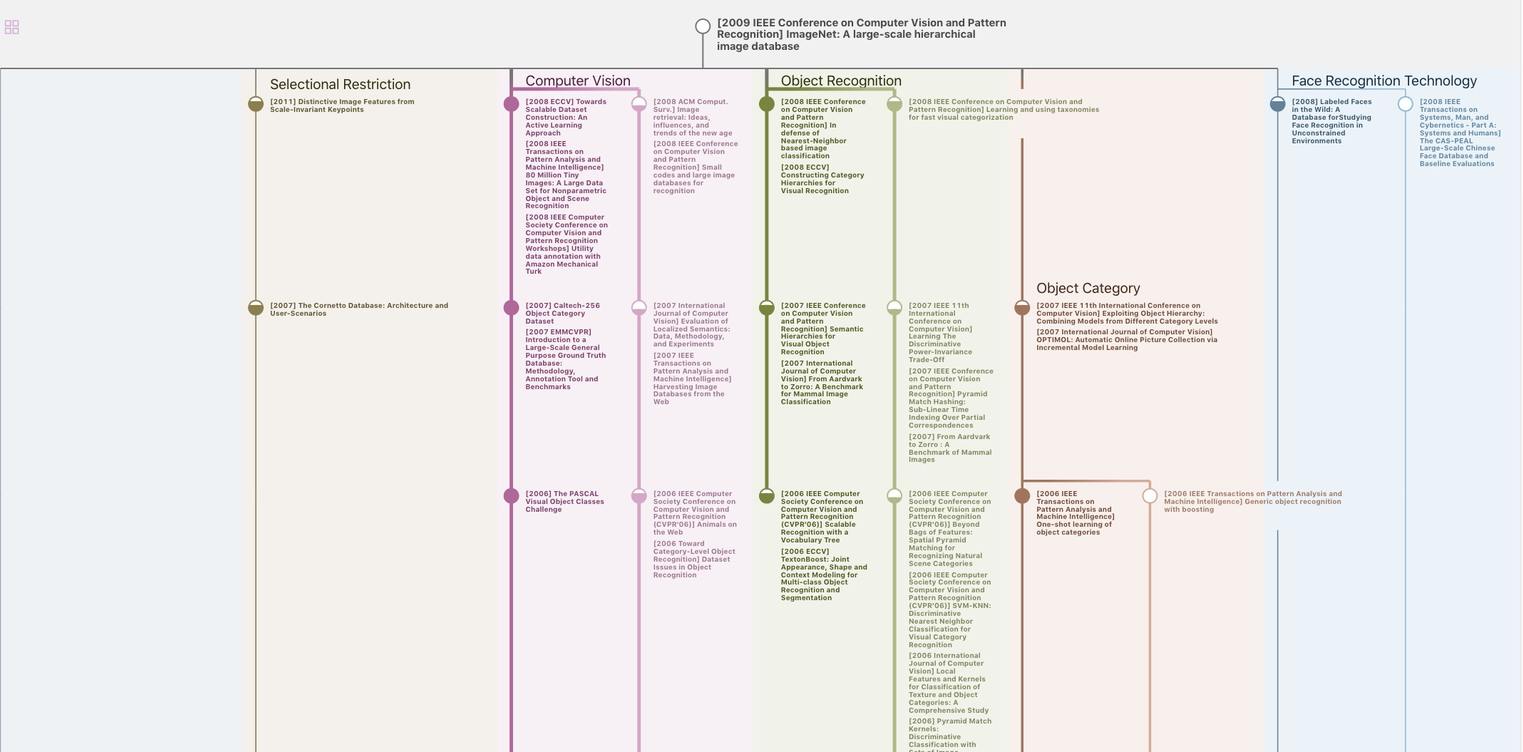
生成溯源树,研究论文发展脉络
Chat Paper
正在生成论文摘要