The development and validation of a non-invasive prediction model of hyperuricemia based on modifiable risk factors: baseline findings of a health examination population cohort.
Food & function(2023)
摘要
This study aims to establish a simple and non-invasive risk prediction model for hyperuricemia in Chinese adults based on modifiable risk factors. In 2020-2021, the baseline survey of the Beijing Health Management Cohort (BHMC) was conducted in Beijing city among the health examination population. Diverse life-style risk factors including dietary patterns and habits, cigarette smoking, alcohol intake, sleep duration and cell-phone use were collected. We developed hyperuricemia prediction models using three machine-learning techniques, namely logistic regression (LR), random forest (RF), and XGBoost. Performances in discrimination, calibration, and clinical applicability of the three methods were compared. Decision curve analysis (DCA) was used to assess the model's clinical usefulness. A total of 74 050 people were included in the study, of whom 55 537 (75%) were randomly selected into the training set and the other 18 513 (25%) were in the validation set. The prevalence of HUA was 38.43% in men and 13.29% in women. The XGBoost model has better performance than the LR and RF models. The area under the curve (AUC) (95% CI) in the training set for the LR, RF and XGBoost models were 0.754 (0.750-0.757), 0.844 (0.841-0.846) and 0.854 (0.851-0.856), respectively. The XGBoost model had a higher classification accuracy of 0.774 than the logistic (0.592) and RF (0.767) models. The AUC (95% CI) values in the validation set for the LR, RF and XGBoost models were 0.758 (0.749-0.765), 0.809 (0.802-0.816) and 0.820 (0.813-0.827), respectively. As demonstrated by the DCA curves, all the three models could bring net benefits within the appropriate threshold probability. XGBoost had better discrimination and accuracy. Various modifiable risk factors included in the model were helpful in facilitating the easy identification and life-style interventions of the HUA high-risk population.
更多查看译文
AI 理解论文
溯源树
样例
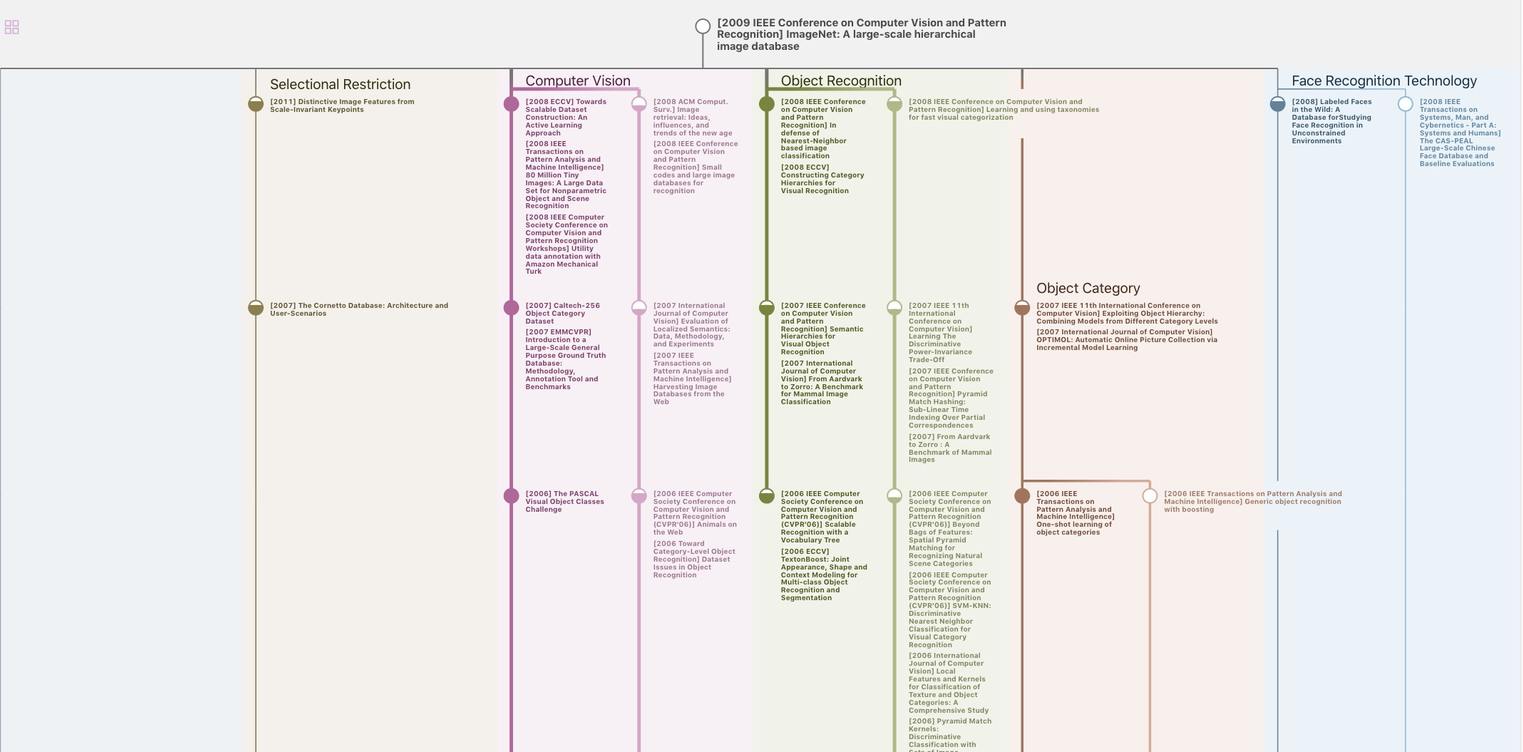
生成溯源树,研究论文发展脉络
Chat Paper
正在生成论文摘要