Reaction performance prediction with an extrapolative and interpretable graph model based on chemical knowledge
Nature Communications(2023)
摘要
Accurate prediction of reactivity and selectivity provides the desired guideline for synthetic development. Due to the high-dimensional relationship between molecular structure and synthetic function, it is challenging to achieve the predictive modelling of synthetic transformation with the required extrapolative ability and chemical interpretability. To meet the gap between the rich domain knowledge of chemistry and the advanced molecular graph model, herein we report a knowledge-based graph model that embeds the digitalized steric and electronic information. In addition, a molecular interaction module is developed to enable the learning of the synergistic influence of reaction components. In this study, we demonstrate that this knowledge-based graph model achieves excellent predictions of reaction yield and stereoselectivity, whose extrapolative ability is corroborated by additional scaffold-based data splittings and experimental verifications with new catalysts. Because of the embedding of local environment, the model allows the atomic level of interpretation of the steric and electronic influence on the overall synthetic performance, which serves as a useful guide for the molecular engineering towards the target synthetic function. This model offers an extrapolative and interpretable approach for reaction performance prediction, pointing out the importance of chemical knowledge-constrained reaction modelling for synthetic purpose.
更多查看译文
关键词
interpretable graph model,chemical,prediction,knowledge,reaction
AI 理解论文
溯源树
样例
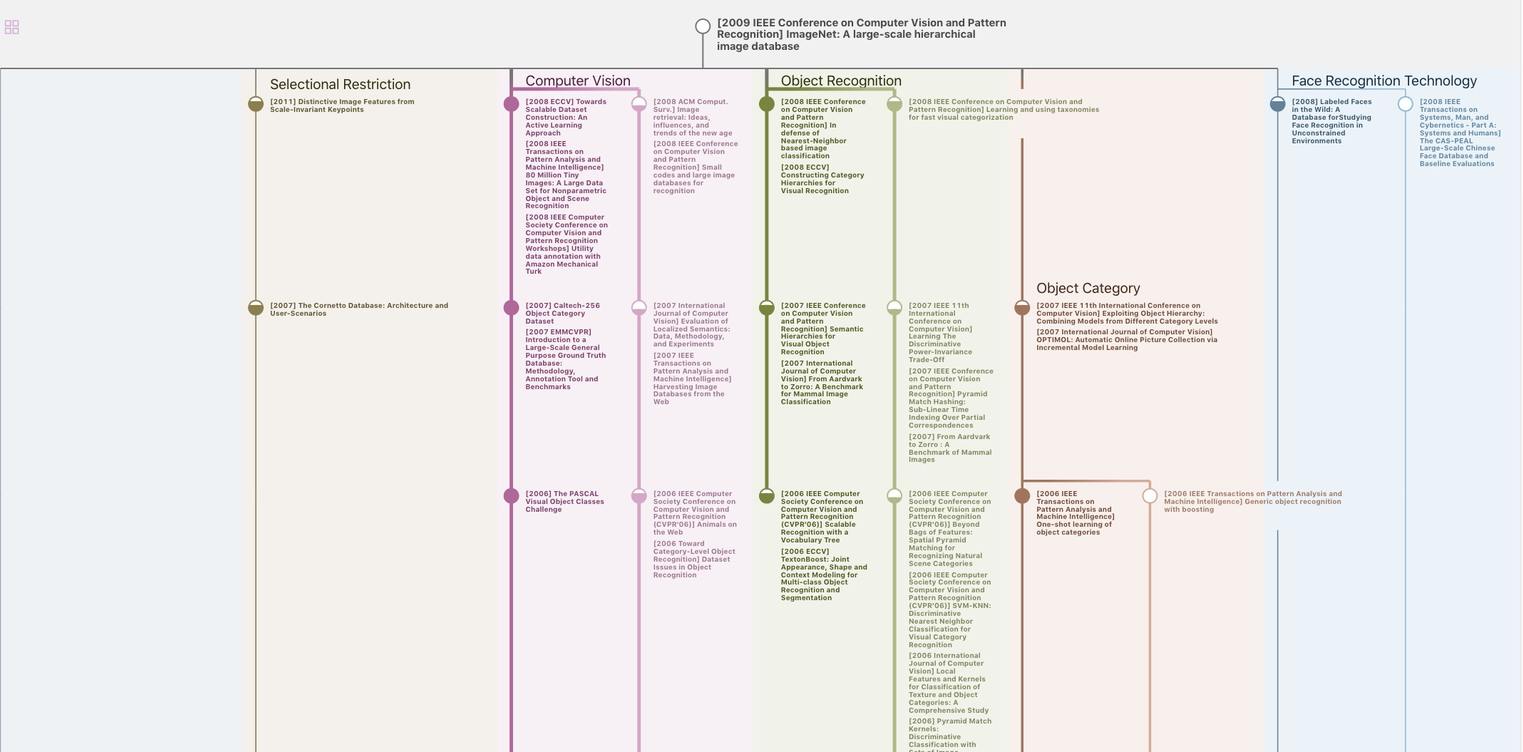
生成溯源树,研究论文发展脉络
Chat Paper
正在生成论文摘要