Predicting the prognosis of HER2-positive breast cancer patients by fusing pathological whole slide images and clinical features using multiple instance learning.
Mathematical biosciences and engineering : MBE(2023)
摘要
In 2022, breast cancer will become an important factor affecting women's public health and HER2 positivity for approximately 15-20$ \% $ invasive breast cancer cases. Follow-up data for HER2-positive patients are rare, and research on prognosis and auxiliary diagnosis is still limited. In light of the findings obtained from the analysis of clinical features, we have developed a novel multiple instance learning (MIL) fusion model that integrates hematoxylin-eosin (HE) pathological images and clinical features to accurately predict the prognostic risk of patients. Specifically, we segmented the HE pathology images of patients into patches, clustered them by K-means, aggregated them into a bag feature-level representation through graph attention networks (GATs) and multihead attention networks, and fused them with clinical features to predict the prognosis of patients. We divided West China Hospital (WCH) patients (n = 1069) into a training cohort and internal validation cohort and used The Cancer Genome Atlas (TCGA) patients (n = 160) as an external test cohort. The 3-fold average C-index of the proposed OS-based model was 0.668, the C-index of the WCH test set was 0.765, and the C-index of the TCGA independent test set was 0.726. By plotting the Kaplan-Meier curve, the fusion feature (P = 0.034) model distinguished high- and low-risk groups more accurately than clinical features (P = 0.19). The MIL model can directly analyze a large number of unlabeled pathological images, and the multimodal model is more accurate than the unimodal models in predicting Her2-positive breast cancer prognosis based on large amounts of data.
更多查看译文
关键词
breast cancer, HER2, multiple instance learning, deep learning, survival analysis, prognosis
AI 理解论文
溯源树
样例
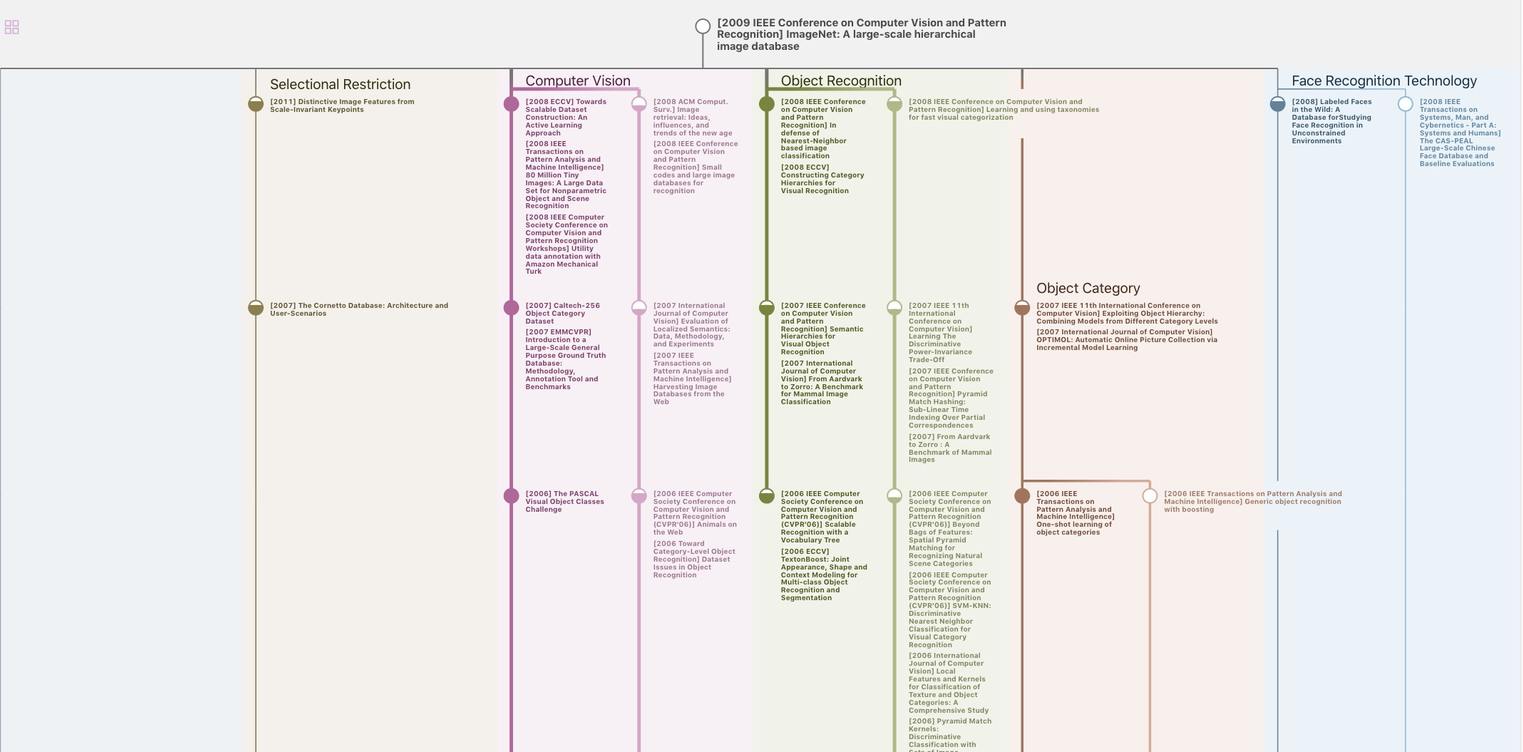
生成溯源树,研究论文发展脉络
Chat Paper
正在生成论文摘要