Prediction of LncRNA-Protein Interactions Based on Kernel Combinations and Graph Convolutional Networks.
IEEE journal of biomedical and health informatics(2024)
摘要
The complexes of long non-coding RNAs bound to proteins can be involved in regulating life activities at various stages of organisms. However, in the face of the growing number of lncRNAs and proteins, verifying LncRNA-Protein Interactions (LPI) based on traditional biological experiments is time-consuming and laborious. Therefore, with the improvement of computing power, predicting LPI has met new development opportunity. In virtue of the state-of-the-art works, a framework called LncRNA-Protein Interactions based on Kernel Combinations and Graph Convolutional Networks (LPI-KCGCN) has been proposed in this article. We first construct kernel matrices by taking advantage of extracting both the lncRNAs and protein concerning the sequence features, sequence similarity features, expression features, and gene ontology. Then reconstruct the existent kernel matrices as the input of the next step. Combined with known LPI interactions, the reconstructed similarity matrices, which can be used as features of the topology map of the LPI network, are exploited in extracting potential representations in the lncRNA and protein space using a two-layer Graph Convolutional Network. The predicted matrix can be finally obtained by training the network to produce scoring matrices w.r.t. lncRNAs and proteins. Different LPI-KCGCN variants are ensemble to derive the final prediction results and testify on balanced and unbalanced datasets. The 5-fold cross-validation shows that the optimal feature information combination on a dataset with 15.5% positive samples has an AUC value of 0.9714 and an AUPR value of 0.9216. On another highly unbalanced dataset with only 5% positive samples, LPI-KCGCN also has outperformed the state-of-the-art works, which achieved an AUC value of 0.9907 and an AUPR value of 0.9267.
更多查看译文
AI 理解论文
溯源树
样例
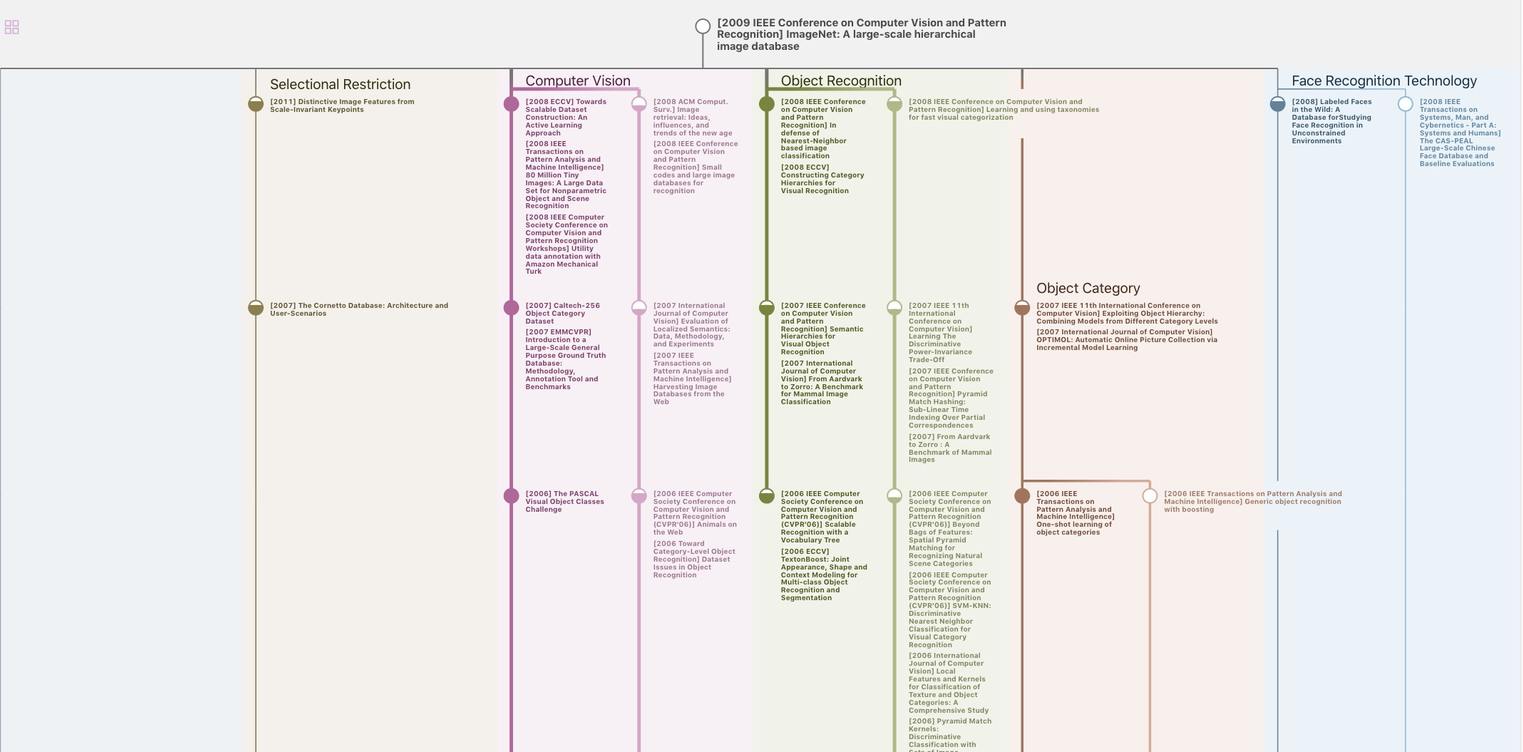
生成溯源树,研究论文发展脉络
Chat Paper
正在生成论文摘要