Evaluating model specification when using the parametric g-formula in the presence of censoring.
American journal of epidemiology(2023)
摘要
The noniterative conditional expectation (NICE) parametric g-formula can be used to estimate the causal effect of sustained treatment strategies. In addition to identifiability conditions, the validity of the NICE parametric g-formula generally requires the correct specification of models for time-varying outcomes, treatments, and confounders at each follow-up time. An informal approach for evaluating model specification is to compare the observed distributions of the outcome, treatments, and confounders with their parametric g-formula estimates under the "natural course." In the presence of losses to follow-up, however, the observed and natural course risks can differ even if the identifiability conditions of the parametric g-formula hold and there is no model misspecification. Here, we describe two approaches to evaluate model specification when using the parametric g-formula in the presence of censoring: (1) comparing factual risks estimated by the g-formula with nonparametric Kaplan-Meier estimates, and (2) comparing natural course risks estimated by inverse probability weighting with those estimated by the g-formula. We also describe how to correctly compute natural course estimates of time-varying covariate means when using a computationally efficient g-formula algorithm. We evaluate the proposed methods via simulation and implement them to estimate the effects of dietary interventions in two cohort studies.
更多查看译文
关键词
censoring, inverse probability weighting, model misspecification, noniterative conditional expectation parametric g-formula
AI 理解论文
溯源树
样例
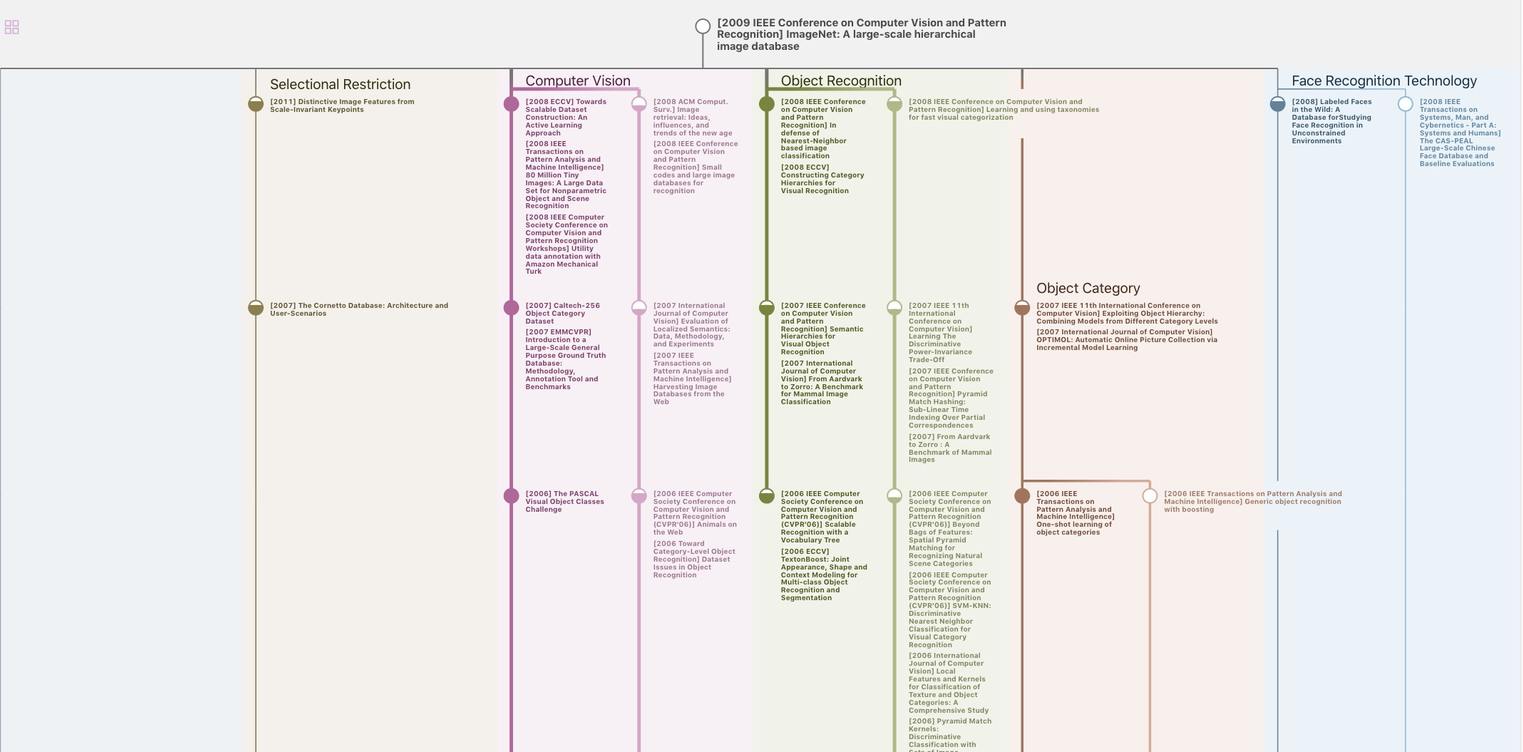
生成溯源树,研究论文发展脉络
Chat Paper
正在生成论文摘要