MM optimization: Proximal distance algorithms, path following, and trust regions.
Proceedings of the National Academy of Sciences of the United States of America(2023)
摘要
We briefly review the majorization-minimization (MM) principle and elaborate on the closely related notion of proximal distance algorithms, a generic approach for solving constrained optimization problems via quadratic penalties. We illustrate how the MM and proximal distance principles apply to a variety of problems from statistics, finance, and nonlinear optimization. Drawing from our selected examples, we also sketch a few ideas pertinent to the acceleration of MM algorithms: a) structuring updates around efficient matrix decompositions, b) path following in proximal distance iteration, and c) cubic majorization and its connections to trust region methods. These ideas are put to the test on several numerical examples, but for the sake of brevity, we omit detailed comparisons to competing methods. The current article, which is a mix of review and current contributions, celebrates the MM principle as a powerful framework for designing optimization algorithms and reinterpreting existing ones.
更多查看译文
关键词
proximal distance algorithms,mm optimization
AI 理解论文
溯源树
样例
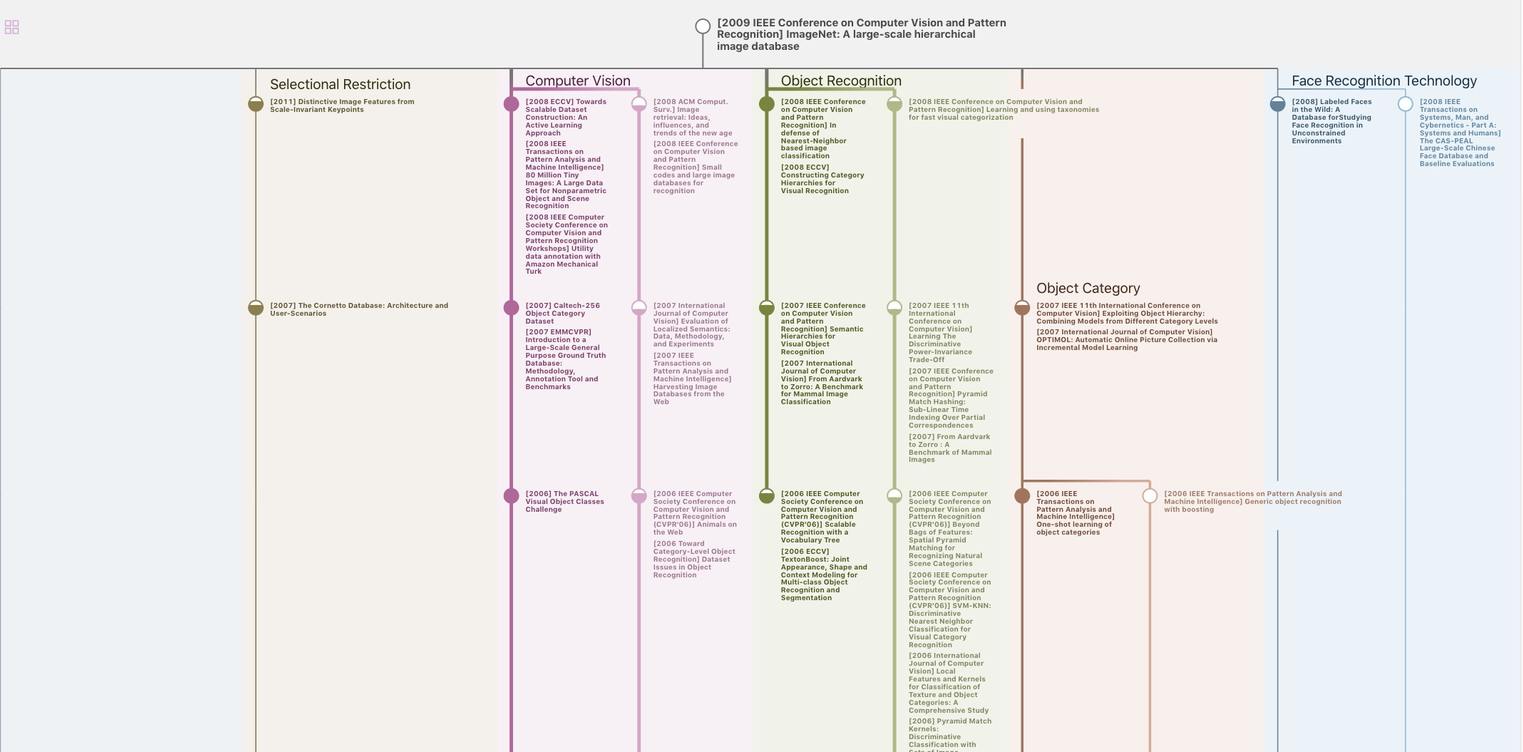
生成溯源树,研究论文发展脉络
Chat Paper
正在生成论文摘要