Quantum Graph Neural Network Models for Materials Search.
Materials (Basel, Switzerland)(2023)
摘要
Inspired by classical graph neural networks, we discuss a novel quantum graph neural network (QGNN) model to predict the chemical and physical properties of molecules and materials. QGNNs were investigated to predict the energy gap between the highest occupied and lowest unoccupied molecular orbitals of small organic molecules. The models utilize the equivariantly diagonalizable unitary quantum graph circuit (EDU-QGC) framework to allow discrete link features and minimize quantum circuit embedding. The results show QGNNs can achieve lower test loss compared to classical models if a similar number of trainable variables are used, and converge faster in training. This paper also provides a review of classical graph neural network models for materials research and various QGNNs.
更多查看译文
关键词
materials search,quantum,models
AI 理解论文
溯源树
样例
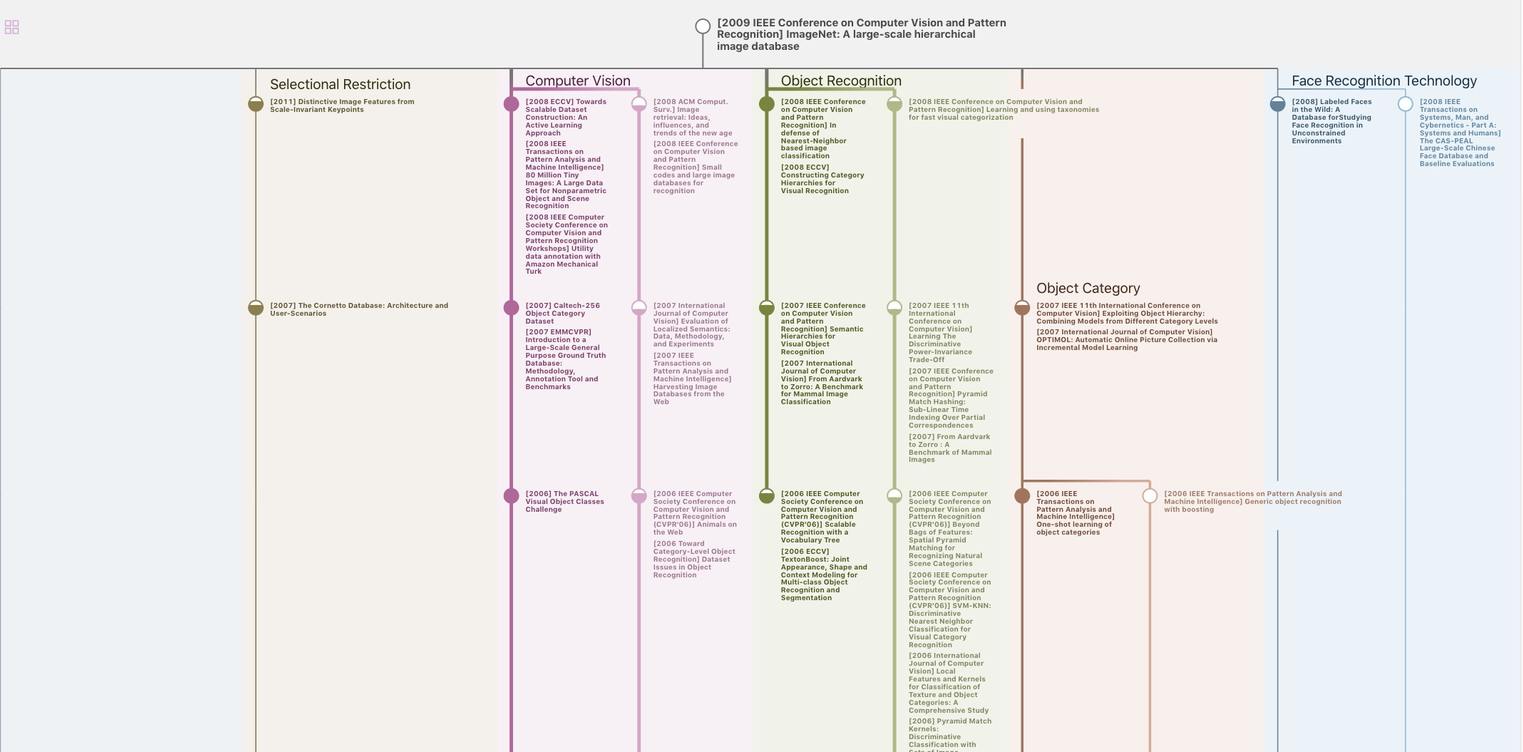
生成溯源树,研究论文发展脉络
Chat Paper
正在生成论文摘要