Segmentation-Free OCT-Volume-Based Deep Learning Model Improves Pointwise Visual Field Sensitivity Estimation
TRANSLATIONAL VISION SCIENCE & TECHNOLOGY(2023)
摘要
Purpose: The structural changes measured by optical coherence tomography (OCT) are related to functional changes in visual fields (VFs). This study aims to accurately assess the structure-function relationship and overcome the challenges brought by the minimal measurable level (floor effect) of segmentation-dependent OCT measurements commonly used in prior studies.Methods: We developed a deep learning model to estimate the functional performance directly from three-dimensional (3D) OCT volumes and compared it to the model trained with segmentation-dependent two-dimensional (2D) OCT thickness maps. Moreover, we proposed a gradient loss to utilize the spatial information of VFs.Results: Our 3D model was significantly better than the 2D model both globally and pointwise regarding both mean absolute error (MAE = 3.11 + 3.54 vs. 3.47 +/- 3.75 dB, P < 0.001) and Pearson's correlation coefficient (0.80 vs. 0.75, P < 0.001). On a subset of test data with floor effects, the 3D model showed less influence from floor effects than the 2D model (MAE = 5.24 +/- 3.99 vs. 6.34 +/- 4.58 dB, P < 0.001, and correlation 0.83 vs. 0.74, P < 0.001). The gradient loss improved the estimation error for low-sensitivity values. Furthermore, our 3D model outperformed all prior studies.Conclusions: By providing a better quantitative model to encapsulate the structure-function relationship more accurately, our method may help deriving VF test surrogates.
更多查看译文
关键词
structure-function relationship,deep learning,ocular imaging
AI 理解论文
溯源树
样例
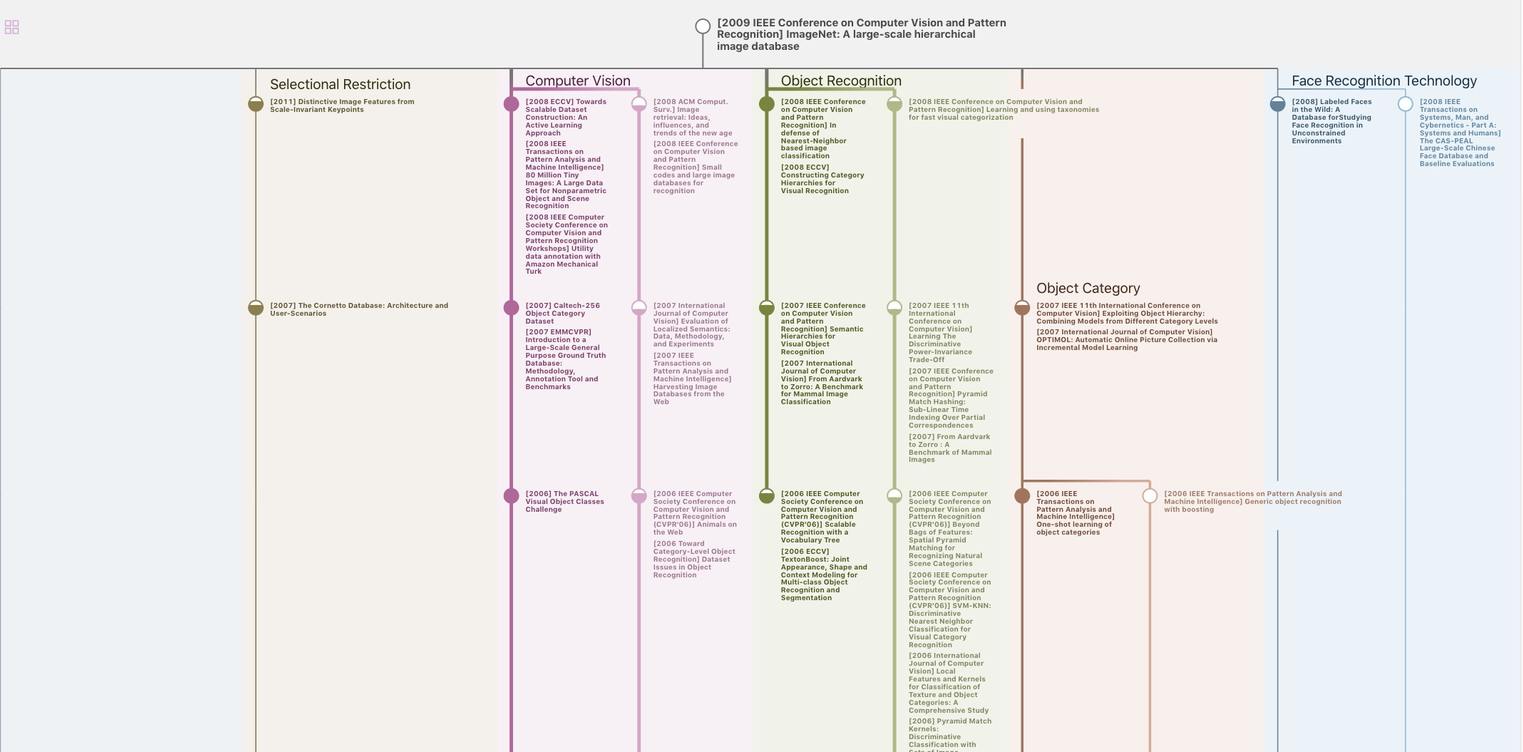
生成溯源树,研究论文发展脉络
Chat Paper
正在生成论文摘要