scFED: Clustering Identifying Cell Types of scRNA-Seq Data Based on Feature Engineering Denoising
Interdisciplinary sciences, computational life sciences(2023)
摘要
Recently developed single-cell RNA-seq (scRNA-seq) technology has given researchers the chance to investigate single-cell level of disease development. Clustering is one of the most essential strategies for analyzing scRNA-seq data. Choosing high-quality feature sets can significantly enhance the outcomes of single-cell clustering and classification. But computationally burdensome and highly expressed genes cannot afford a stabilized and predictive feature set for technical reasons. In this study, we introduce scFED, a feature-engineered gene selection framework. scFED identifies prospective feature sets to eliminate the noise fluctuation. And fuse them with existing knowledge from the tissue-specific cellular taxonomy reference database (CellMatch) to avoid the influence of subjective factors. Then present a reconstruction approach for noise reduction and crucial information amplification. We apply scFED on four genuine single-cell datasets and compare it with other techniques. According to the results, scFED improves clustering, decreases dimension of the scRNA-seq data, improves cell type identification when combined with clustering algorithms, and has higher performance than other methods. Therefore, scFED offers certain benefits in scRNA-seq data gene selection. Graphical abstract
更多查看译文
关键词
scRNA-seq, Feature engineering, Reconstruction, Clustering, Classification
AI 理解论文
溯源树
样例
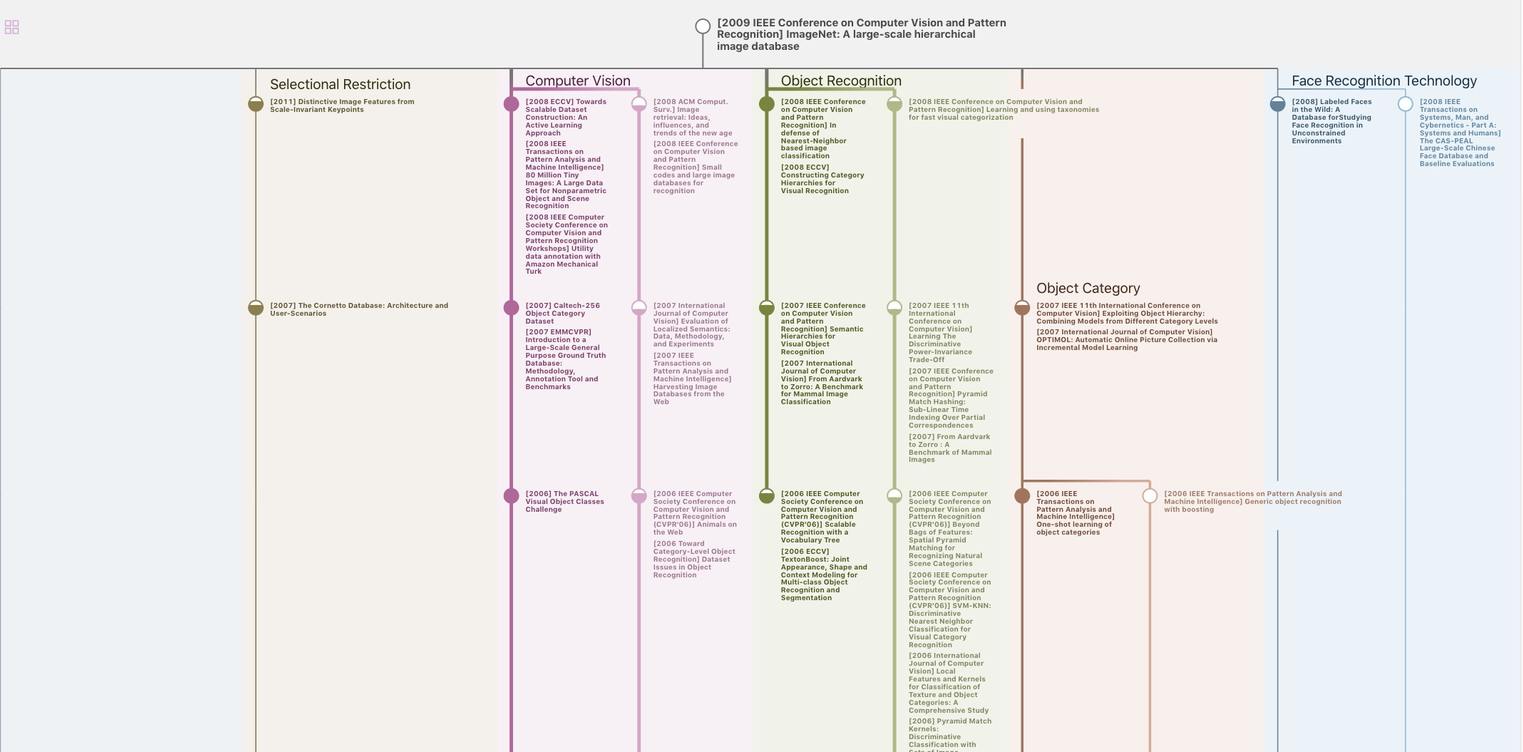
生成溯源树,研究论文发展脉络
Chat Paper
正在生成论文摘要