The Three-Lead EEG Sensor: Introducing an EEG-Assisted Depression Diagnosis System Based on Ant Lion Optimization
IEEE TRANSACTIONS ON BIOMEDICAL CIRCUITS AND SYSTEMS(2023)
摘要
For depression diagnosis, traditional methods such as interviews and clinical scales have been widely leveraged in the past few decades, but they are subjective, time-consuming, and labor-consuming. With the development of affective computing and Artificial Intelligence (AI) technologies, Electroencephalogram (EEG)-based depression detection methods have emerged. However, previous research has virtually neglected practical application scenarios, as most studies have focused on analyzing and modeling EEG data. Furthermore, EEG data is typically obtained from specialized devices that are large, complex to operate, and poorly ubiquitous. To address these challenges, a wearable three-lead EEG sensor with flexible electrodes was developed to obtain prefrontal lobe EEG data. Experimental measurements show that the EEG sensor achieves promising performance (background noise of no more than 0.91 mu Vpp, Signal-to-Noise Ratio (SNR) of 26-48 dB, and electrode-skin contact impedance of less than 1 K Omega). In addition, EEG data from 70 depressed patients and 108 healthy controls were collected using the EEG sensor, and the linear and nonlinear features were extracted. The features were then weighted and selected using the Ant Lion Optimization (ALO) algorithm to improve classification performance. The experimental results show that the k-NN classifier achieves a classification accuracy of 90.70%, specificity of 96.53%, and sensitivity of 81.79%, indicating the promising potential of the three-lead EEG sensor combined with the ALO algorithm and the k-NN classifier for EEG-assisted depression diagnosis.
更多查看译文
关键词
Depression diagnosis,wearable EEG sensor,ALO algorithm,feature weighting and feature selection
AI 理解论文
溯源树
样例
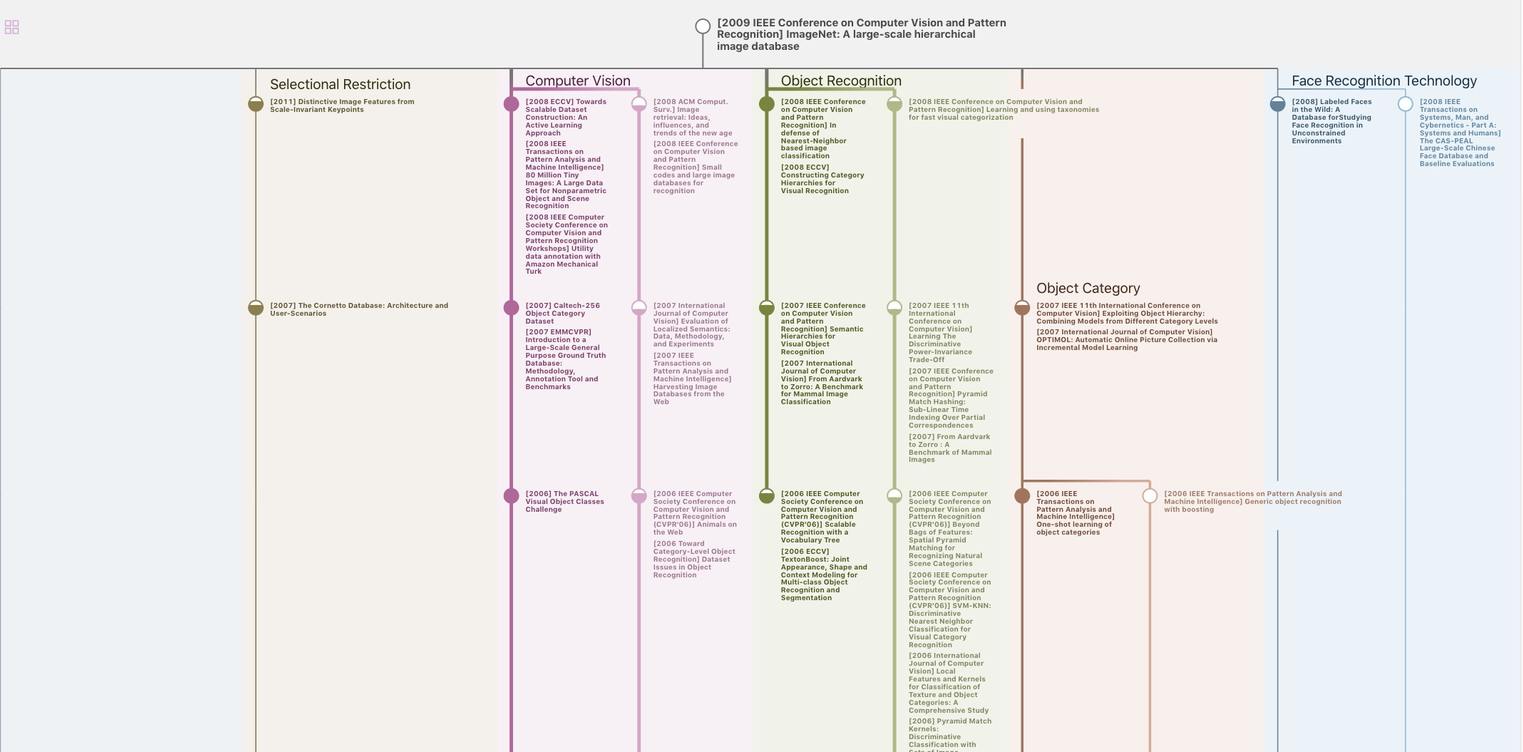
生成溯源树,研究论文发展脉络
Chat Paper
正在生成论文摘要