Diabetes Detection Models in Mexican Patients by Combining Machine Learning Algorithms and Feature Selection Techniques for Clinical and Paraclinical Attributes: A Comparative Evaluation.
Journal of diabetes research(2023)
摘要
The development of medical diagnostic models to support healthcare professionals has witnessed remarkable growth in recent years. Among the prevalent health conditions affecting the global population, diabetes stands out as a significant concern. In the domain of diabetes diagnosis, machine learning algorithms have been widely explored for generating disease detection models, leveraging diverse datasets primarily derived from clinical studies. The performance of these models heavily relies on the selection of the classifier algorithm and the quality of the dataset. Therefore, optimizing the input data by selecting relevant features becomes essential for accurate classification. This research presents a comprehensive investigation into diabetes detection models by integrating two feature selection techniques: the Akaike information criterion and genetic algorithms. These techniques are combined with six prominent classifier algorithms, including support vector machine, random forest, k-nearest neighbor, gradient boosting, extra trees, and naive Bayes. By leveraging clinical and paraclinical features, the generated models are evaluated and compared to existing approaches. The results demonstrate superior performance, surpassing accuracies of 94%. Furthermore, the use of feature selection techniques allows for working with a reduced dataset. The significance of feature selection is underscored in this study, showcasing its pivotal role in enhancing the performance of diabetes detection models. By judiciously selecting relevant features, this approach contributes to the advancement of medical diagnostic capabilities and empowers healthcare professionals in making informed decisions regarding diabetes diagnosis and treatment.
更多查看译文
关键词
feature selection techniques,diabetes,machine learning algorithms,mexican patients,machine learning
AI 理解论文
溯源树
样例
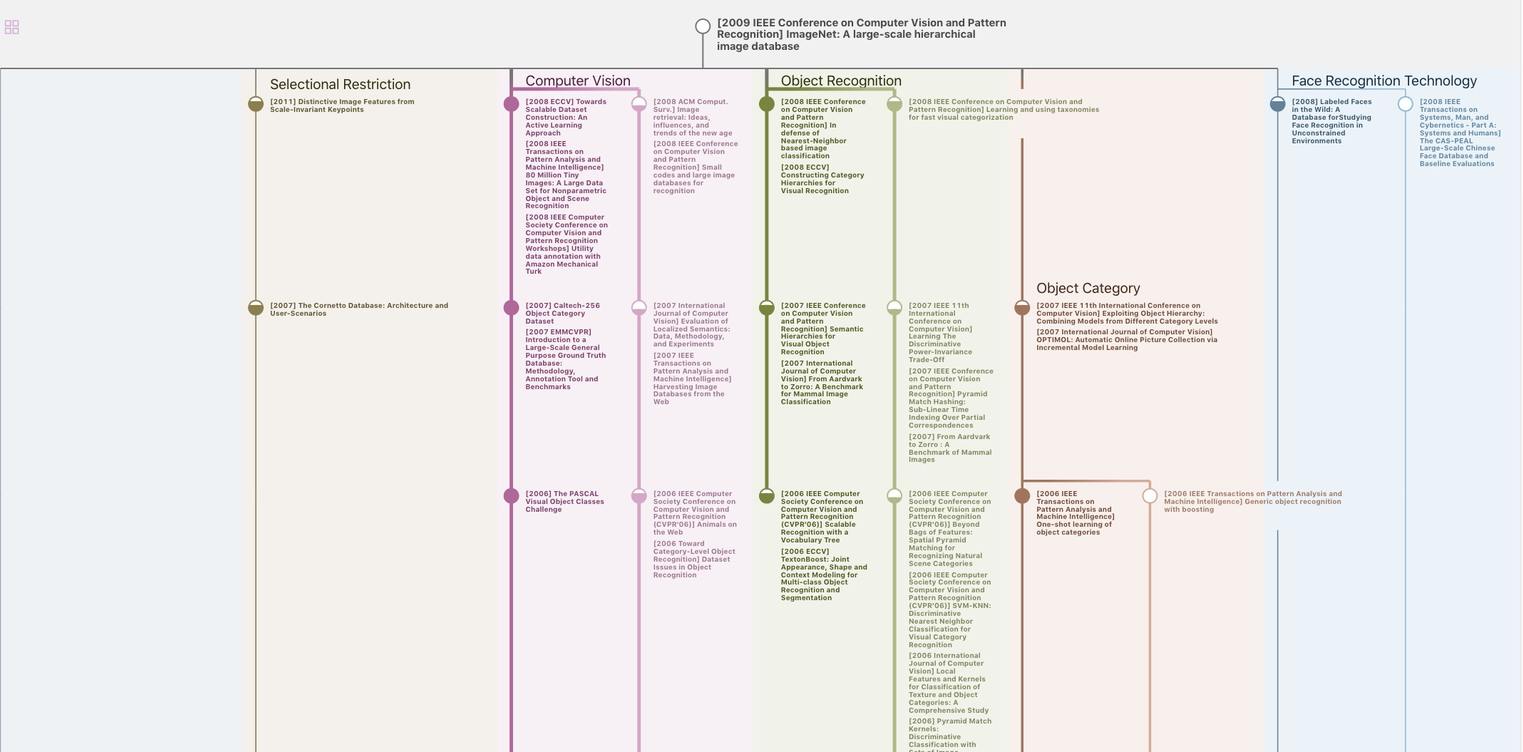
生成溯源树,研究论文发展脉络
Chat Paper
正在生成论文摘要