Using quantitative systems pharmacology modeling to optimize combination therapy of anti-PD-L1 checkpoint inhibitor and T cell engager.
Frontiers in pharmacology(2023)
摘要
Although immune checkpoint blockade therapies have shown evidence of clinical effectiveness in many types of cancer, the outcome of clinical trials shows that very few patients with colorectal cancer benefit from treatments with checkpoint inhibitors. Bispecific T cell engagers (TCEs) are gaining popularity because they can improve patients' immunological responses by promoting T cell activation. The possibility of combining TCEs with checkpoint inhibitors to increase tumor response and patient survival has been highlighted by preclinical and clinical outcomes. However, identifying predictive biomarkers and optimal dose regimens for individual patients to benefit from combination therapy remains one of the main challenges. In this article, we describe a modular quantitative systems pharmacology (QSP) platform for immuno-oncology that includes specific processes of immune-cancer cell interactions and was created based on published data on colorectal cancer. We generated a virtual patient cohort with the model to conduct virtual clinical trials for combination therapy of a PD-L1 checkpoint inhibitor (atezolizumab) and a bispecific T cell engager (cibisatamab). Using the model calibrated against the clinical trials, we conducted several virtual clinical trials to compare various doses and schedules of administration for two drugs with the goal of therapy optimization. Moreover, we quantified the score of drug synergy for these two drugs to further study the role of the combination therapy.
更多查看译文
关键词
quantitative systems pharmacology modeling,combination therapy,inhibitor,anti-pd-l
AI 理解论文
溯源树
样例
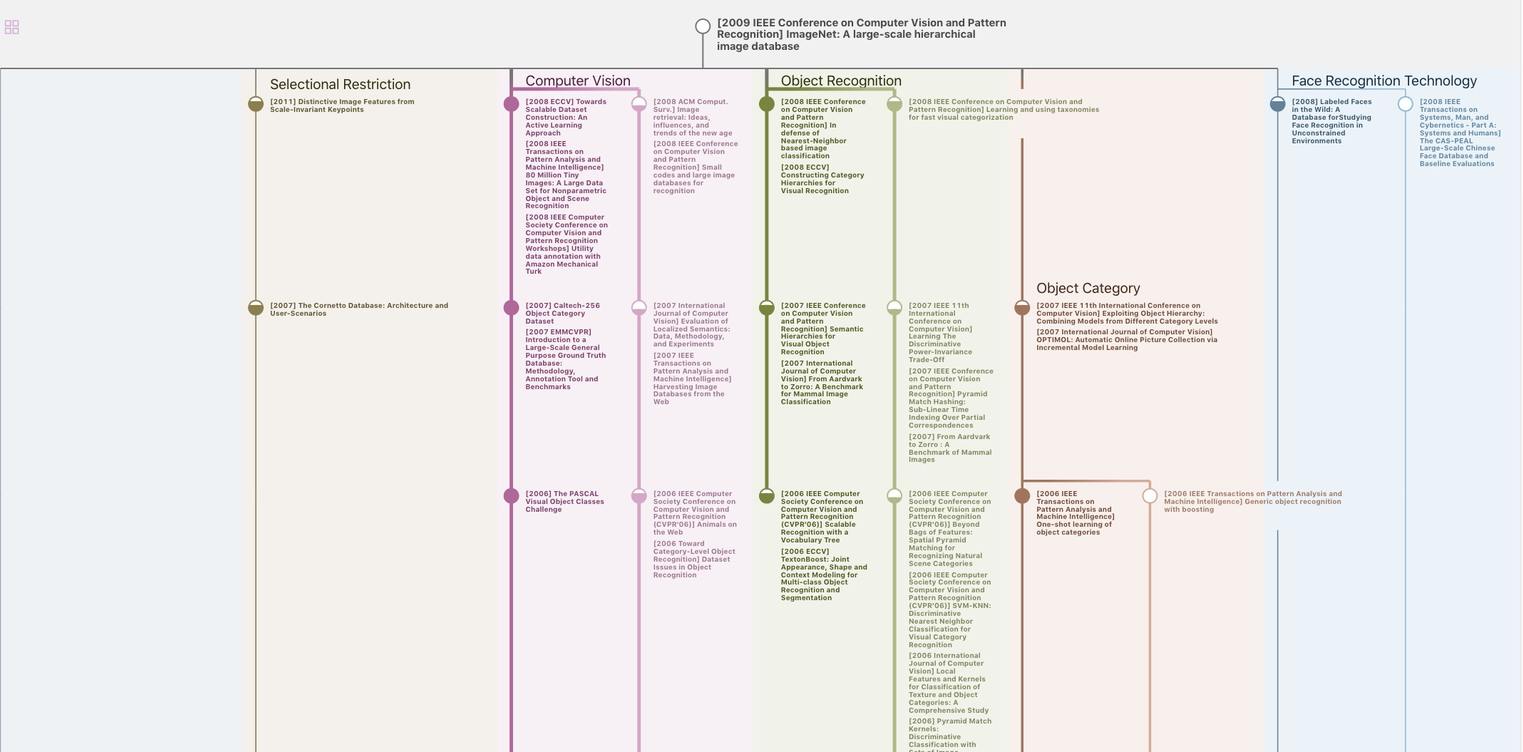
生成溯源树,研究论文发展脉络
Chat Paper
正在生成论文摘要