Automatic Recognition of Schizophrenia from Brain-Network Features Using Graph Convolutional Neural Network
ASIAN JOURNAL OF PSYCHIATRY(2023)
摘要
Schizophrenia is a severe mental illness that imposes considerable economic burden on families and society. However, its clinical diagnosis primarily relies on scales and doctors' clinical experience and lacks an objective and accurate diagnostic approach. In recent years, graph convolutional neural networks (GCN) have been used to assist in psychiatric diagnosis owing to their ability to learn spatial-association information. Therefore, this study proposes a schizophrenia automatic recognition model based on graph convolutional neural network. Herein, the resting-state electroencephalography (EEG) data of 103 first-episode schizophrenia patients and 92 normal controls (NCs) were obtained. The automatic recognition model was trained with a nodal feature matrix that comprised the time and frequency-domain features of the EEG signals and local features of the brain network. The most significant regions that contributed to the model classification were identified, and the correlation between the node topological features of each significant region and clinical evaluation metrics was explored. Experiments were conducted to evaluate the performance of the model using 10-fold cross-validation. The best performance in the theta frequency band with a 6 s epoch length and phase-locked value. The recognition accuracy was 90.01%. The most significant region for identifying with first-episode schizophrenia patients and NCs was located in the parietal lobe. The results of this study verify the applicability of the proposed novel method for the identification and diagnosis of schizophrenia.
更多查看译文
关键词
Schizophrenia,Graph convolutional neural network,Frequency band,Epoch length,Functional connectivity metrics
AI 理解论文
溯源树
样例
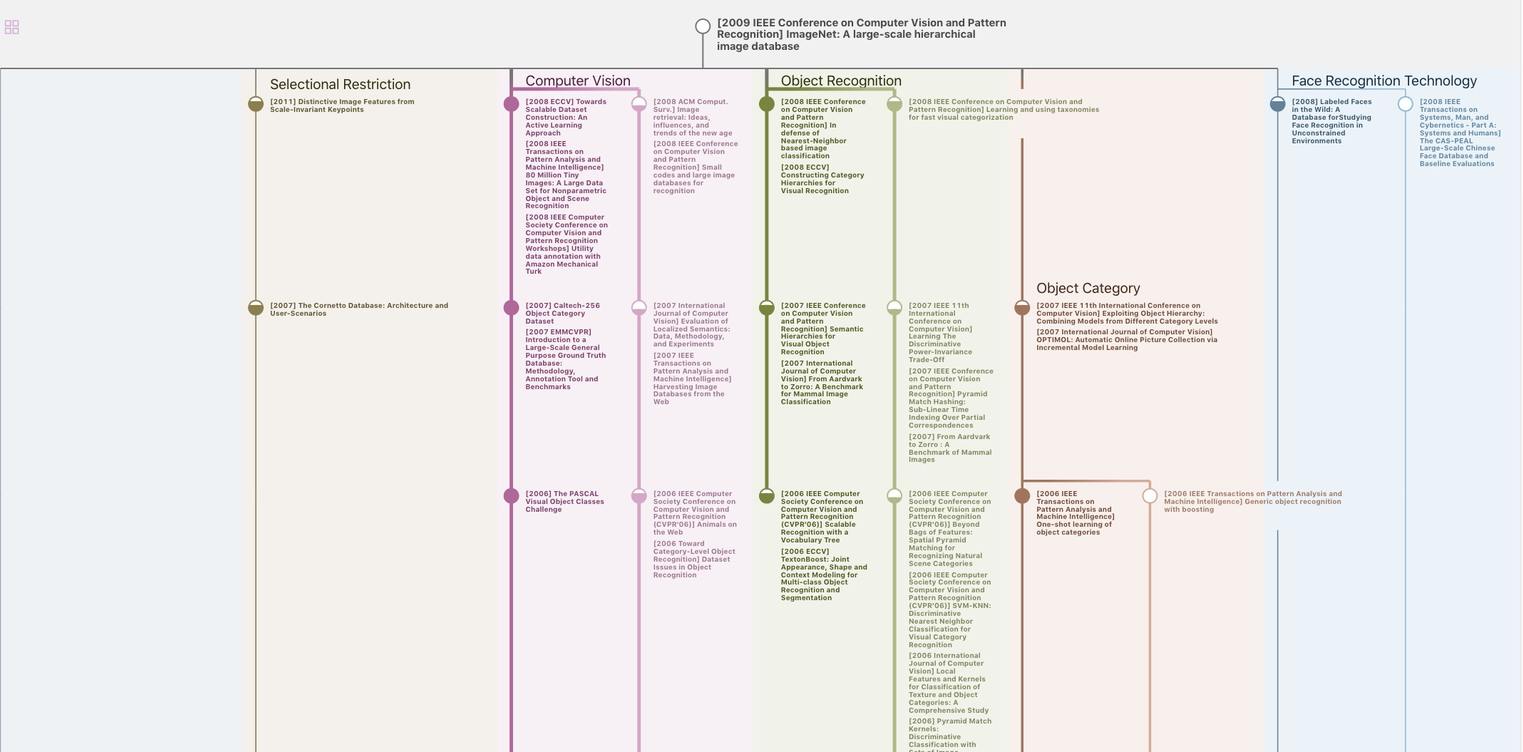
生成溯源树,研究论文发展脉络
Chat Paper
正在生成论文摘要