Graph Neural Network-Guided Contrastive Learning for Sequential Recommendation.
Sensors (Basel, Switzerland)(2023)
摘要
Sequential recommendation uses contrastive learning to randomly augment user sequences and alleviate the data sparsity problem. However, there is no guarantee that the augmented positive or negative views remain semantically similar. To address this issue, we propose graph neural network-guided contrastive learning for sequential recommendation (GC4SRec). The guided process employs graph neural networks to obtain user embeddings, an encoder to determine the importance score of each item, and various data augmentation methods to construct a contrast view based on the importance score. Experimental validation is conducted on three publicly available datasets, and the experimental results demonstrate that GC4SRec improves the hit rate and normalized discounted cumulative gain metrics by 1.4% and 1.7%, respectively. The model can enhance recommendation performance and mitigate the data sparsity problem.
更多查看译文
关键词
sequential recommendation, graph neural networks, contrastive learning, graph neural networks guided
AI 理解论文
溯源树
样例
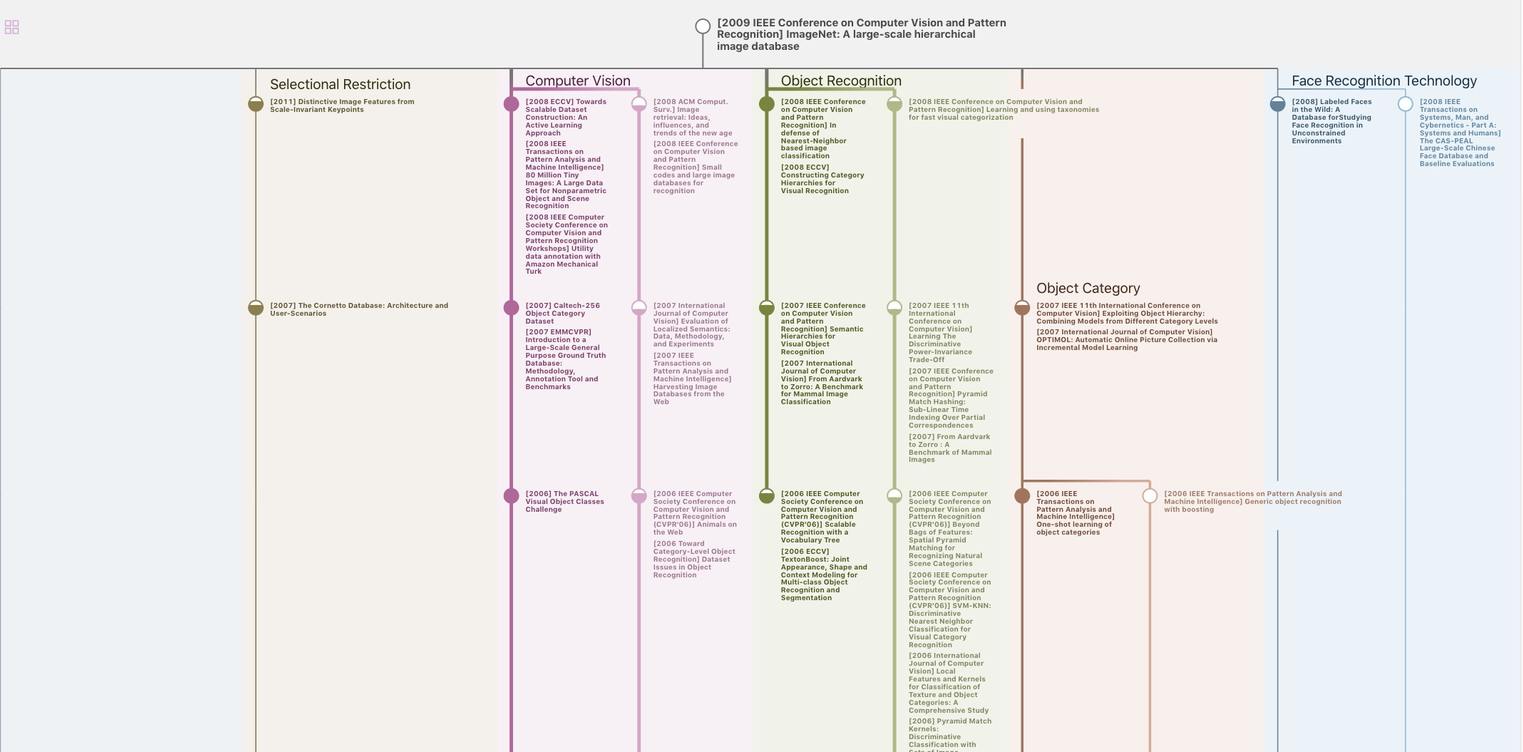
生成溯源树,研究论文发展脉络
Chat Paper
正在生成论文摘要