FCAN-XGBoost: A Novel Hybrid Model for EEG Emotion Recognition.
Sensors (Basel, Switzerland)(2023)
摘要
In recent years, artificial intelligence (AI) technology has promoted the development of electroencephalogram (EEG) emotion recognition. However, existing methods often overlook the computational cost of EEG emotion recognition, and there is still room for improvement in the accuracy of EEG emotion recognition. In this study, we propose a novel EEG emotion recognition algorithm called FCAN-XGBoost, which is a fusion of two algorithms, FCAN and XGBoost. The FCAN module is a feature attention network (FANet) that we have proposed for the first time, which processes the differential entropy () and power spectral density () features extracted from the four frequency bands of the EEG signal and performs feature fusion and deep feature extraction. Finally, the deep features are fed into the eXtreme Gradient Boosting (XGBoost) algorithm to classify the four emotions. We evaluated the proposed method on the DEAP and DREAMER datasets and achieved a four-category emotion recognition accuracy of 95.26% and 94.05%, respectively. Additionally, our proposed method reduces the computational cost of EEG emotion recognition by at least 75.45% for computation time and 67.51% for memory occupation. The performance of FCAN-XGBoost outperforms the state-of-the-art four-category model and reduces computational costs without losing classification performance compared with other models.
更多查看译文
关键词
EEG, emotion recognition, feature fusion, FANet, FCAN-XGBoost
AI 理解论文
溯源树
样例
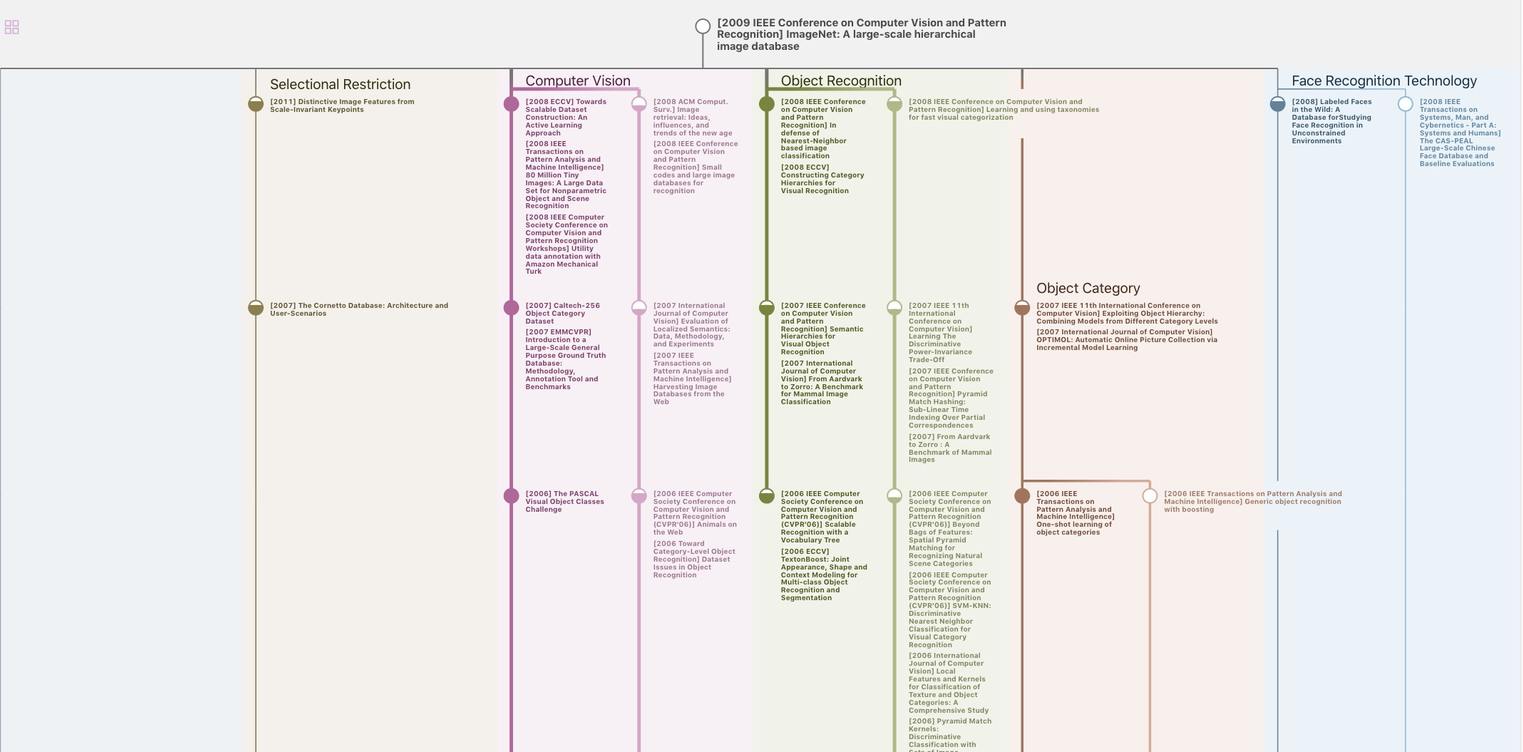
生成溯源树,研究论文发展脉络
Chat Paper
正在生成论文摘要