MS-FANet: Multi-scale feature attention network for liver tumor segmentation.
Computers in biology and medicine(2023)
摘要
Accurate segmentation of liver tumors is a prerequisite for early diagnosis of liver cancer. Segmentation networks extract features continuously at the same scale, which cannot adapt to the variation of liver tumor volume in computed tomography (CT). Hence, a multi-scale feature attention network (MS-FANet) for liver tumor segmentation is proposed in this paper. The novel residual attention (RA) block and multi-scale atrous downsampling (MAD) are introduced in the encoder of MS-FANet to sufficiently learn variable tumor features and extract tumor features at different scales simultaneously. The dual-path feature (DF) filter and dense upsampling (DU) are introduced in the feature reduction process to reduce effective features for the accurate segmentation of liver tumors. On the public LiTS dataset and 3DIRCADb dataset, MS-FANet achieved 74.2% and 78.0% of average Dice, respectively, outperforming most state-of-the-art networks, this strongly proves the excellent liver tumor segmentation performance and the ability to learn features at different scales.
更多查看译文
关键词
Liver tumor segmentation,MS-FANet,Multi-scale features,Feature reduction,Segmentation efficiency
AI 理解论文
溯源树
样例
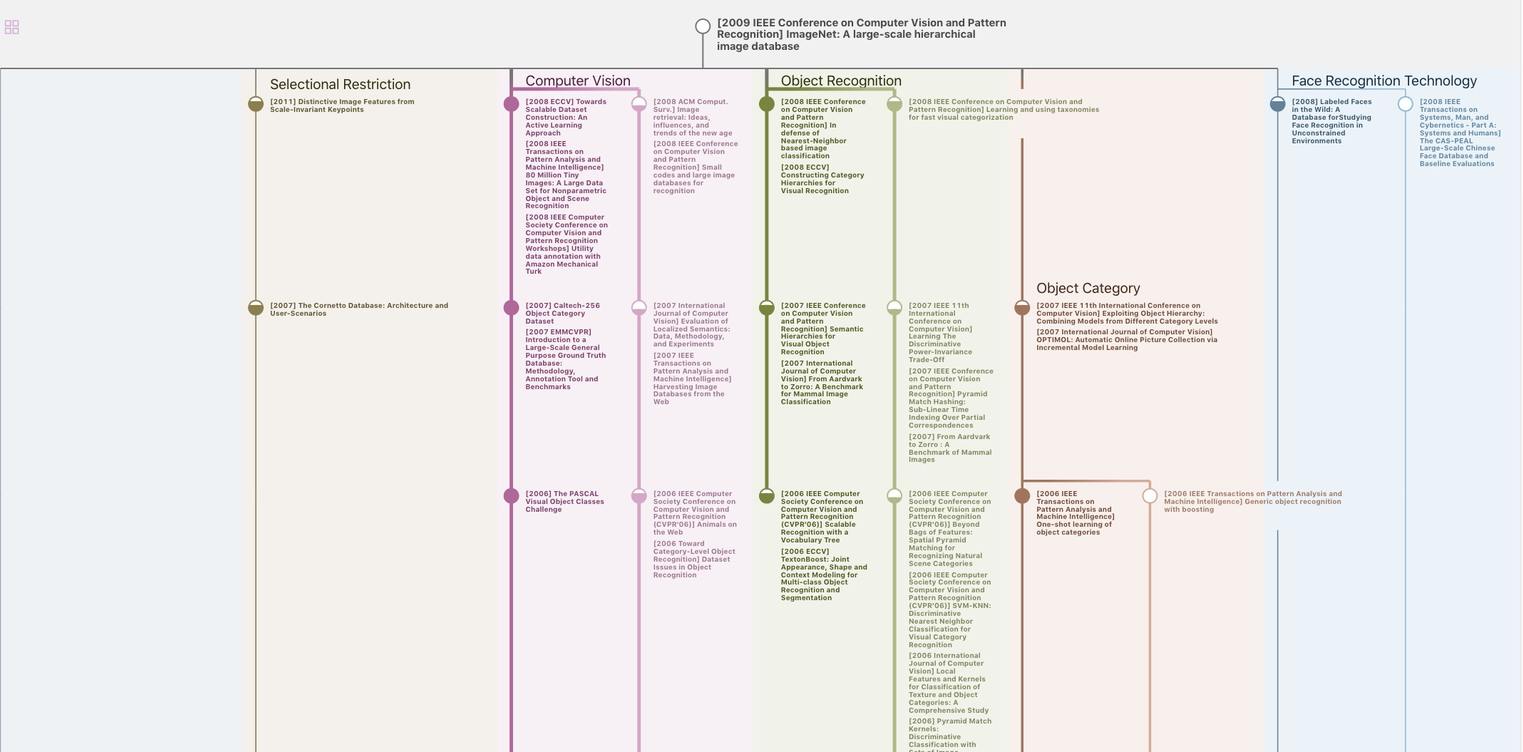
生成溯源树,研究论文发展脉络
Chat Paper
正在生成论文摘要