Age Estimation Based on 3D Pulp Segmentation of First Molars from CBCT Images Using U-Net.
Dento-maxillo-facial radiology/Dentomaxillofacial radiology(2023)
Abstract
Objective: To train a U- Net model to segment the intact pulp cavity of first molars and establish a reliable mathematical model for age estimation. Methods: We trained a U- Net model by 20 sets of cone- beam CT images and this model was able to segment the intact pulp cavity of first molars. Utilizing this model, 239 maxillary first molars and 234 mandibular first molars from 142 males and 135 females aged 15-69 years old were segmented and the intact pulp cavity volumes were calculated, followed by logarithmic regression analysis to establish the mathematical model with age as the dependent variable and pulp cavity volume as the independent variable. Another 256 first molars were collected to estimate ages with the established model. Mean absolute error and root mean square error between the actual and the estimated ages were used to assess the precision and accuracy of the model. Results: The dice similarity coefficient of the U- Net model was 95.6%. The established age estimation model was Age = 148.671 - 30.262 x ln V (V is the intact pulp cavity volume of the first molars). The coefficient of determination (R2), mean absolute error and root mean square error were 0.662, 6.72 years, and 8.26 years, respectively. Conclusion: The trained U- Net model can accurately segment pulp cavity of the first molars from three- dimensional cone- beam CT images. The segmented pulp cavity volumes could be used to estimate the human ages with reasonable precision and accuracy.
MoreTranslated text
Key words
Age estimation,CBCT,First molar,Medical image segmentation,U-Net
AI Read Science
Must-Reading Tree
Example
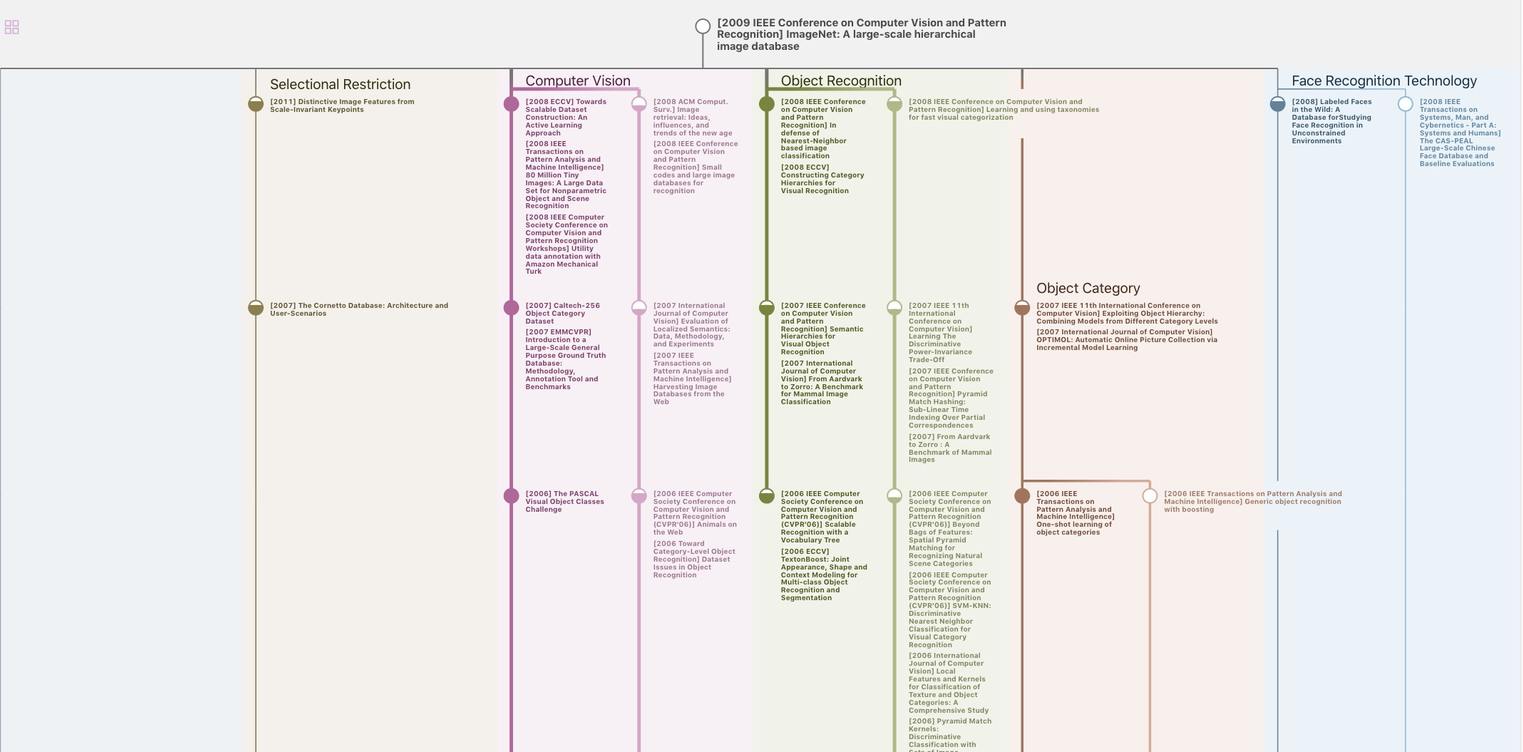
Generate MRT to find the research sequence of this paper
Chat Paper
Summary is being generated by the instructions you defined