Predicting Rectal Cancer Response to Total Neoadjuvant Treatment Using an Artificial Intelligence Model Based on Magnetic Resonance Imaging and Clinical Data
Technology in cancer research & treatment(2023)
摘要
Purpose To develop a model for predicting response to total neoadjuvant treatment (TNT) for patients with locally advanced rectal cancer (LARC) based on baseline magnetic resonance imaging (MRI) and clinical data using artificial intelligence methods. Methods Baseline MRI and clinical data were curated from patients with LARC and analyzed using logistic regression (LR) and deep learning (DL) methods to predict TNT response retrospectively. We defined two groups of response to TNT as pathological complete response (pCR) versus non-pCR (Group 1), and high sensitivity [tumor regression grade (TRG) 0 and TRG 1] versus moderate sensitivity (TRG 2 or patients with TRG 3 and a reduction in tumor volume of at least 20% compared to baseline) versus low sensitivity (TRG 3 and a reduction in tumor volume <20% compared to baseline) (Group 2). We extracted and selected clinical and radiomic features on baseline T2WI. Then we built LR models and DL models. Receiver operating characteristic (ROC) curves analysis was performed to assess predictive performance of models. Results Eighty-nine patients were assigned to the training cohort, and 29 patients were assigned to the testing cohort. The area under receiver operating characteristics curve (AUC) of LR models, which were predictive of high sensitivity and pCR, were 0.853 and 0.866, respectively. Whereas the AUCs of DL models were 0.829 and 0.838, respectively. After 10 rounds of cross validation, the accuracy of the models in Group 1 is higher than in Group 2. Conclusion There was no significant difference between LR model and DL model. Artificial Intelligence-based radiomics biomarkers may have potential clinical implications for adaptive and personalized therapy.
更多查看译文
关键词
artificial intelligence,MRI,response,TNT,rectal cancer
AI 理解论文
溯源树
样例
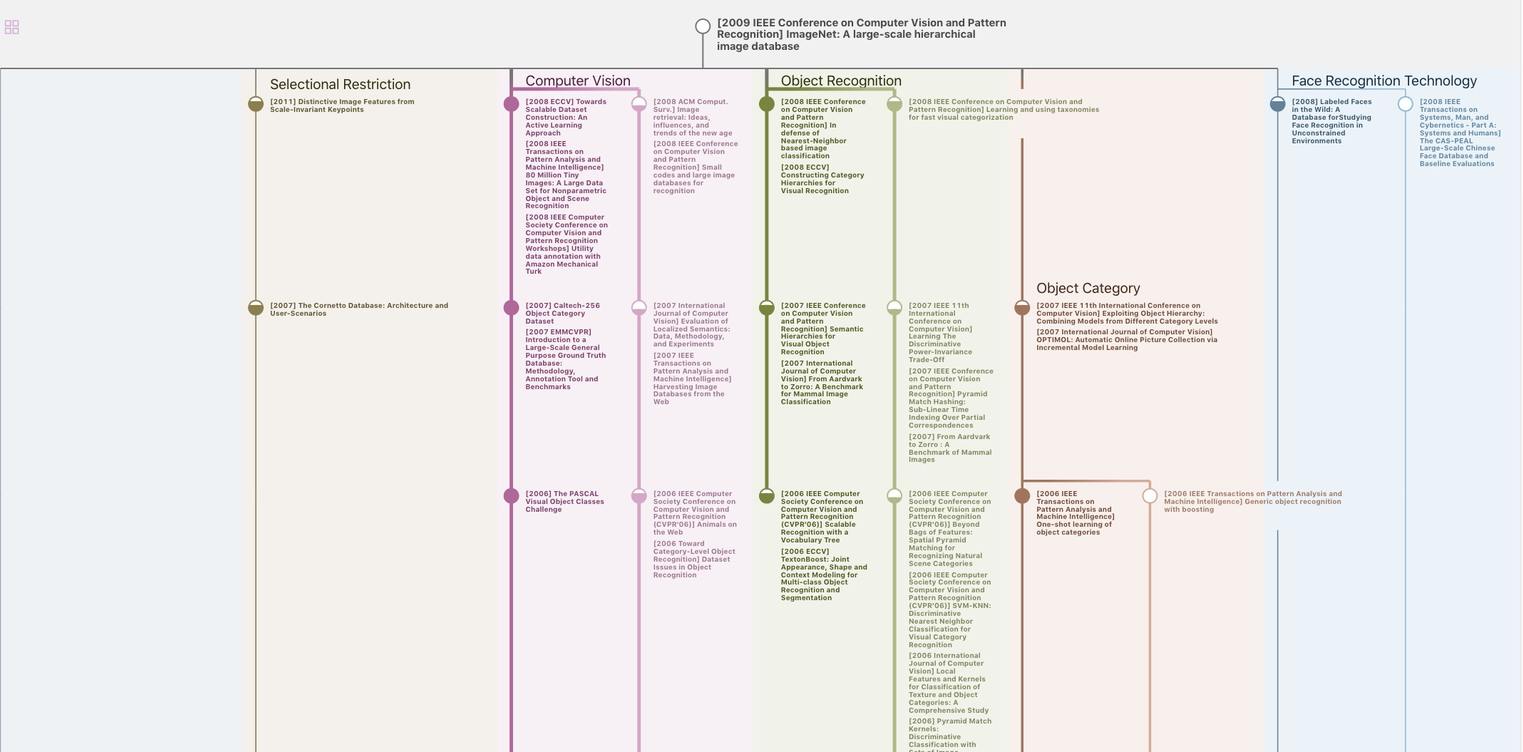
生成溯源树,研究论文发展脉络
Chat Paper
正在生成论文摘要