Efficient and Robust Video Object Segmentation through Isogenous Memory Sampling and Frame Relation Mining.
IEEE transactions on image processing : a publication of the IEEE Signal Processing Society(2023)
摘要
Recently, memory-based methods have achieved remarkable progress in video object segmentation. However, the segmentation performance is still limited by error accumulation and redundant memory, primarily because of 1) the semantic gap caused by similarity matching and memory reading via heterogeneous key-value encoding; 2) the continuously growing and inaccurate memory through directly storing unreliable predictions of all previous frames. To address these issues, we propose an efficient, effective, and robust segmentation method based on Isogenous Memory Sampling and Frame-Relation mining (IMSFR). Specifically, by utilizing an isogenous memory sampling module, IMSFR consistently conducts memory matching and reading between sampled historical frames and the current frame in an isogenous space, minimizing the semantic gap while speeding up the model through an efficient random sampling. Furthermore, to avoid key information loss during the sampling process, we further design a frame-relation temporal memory module to mine inter-frame relations, thereby effectively preserving contextual information from the video sequence and alleviating error accumulation. Extensive experiments demonstrate the effectiveness and efficiency of the proposed IMSFR method. In particular, our IMSFR achieves state-of-the-art performance on six commonly used benchmarks in terms of region similarity & contour accuracy and speed. Our model also exhibits strong robustness against frame sampling due to its large receptive field.
更多查看译文
关键词
Video object segmentation, isogenous memory sampling, frame-relation mining, temporal memory
AI 理解论文
溯源树
样例
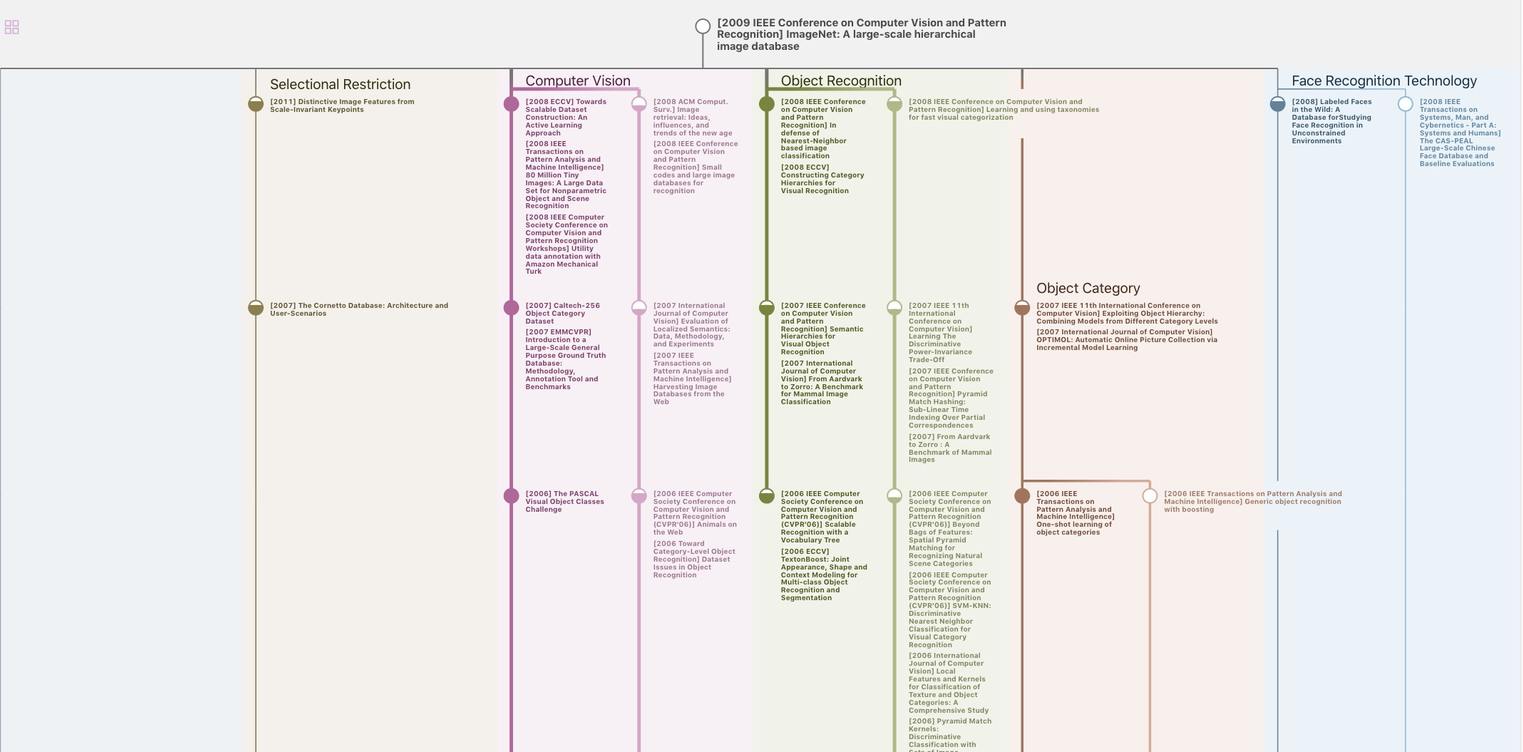
生成溯源树,研究论文发展脉络
Chat Paper
正在生成论文摘要