Toward high-performance energy and power battery cells with machine learning-based optimization of electrode manufacturing
JOURNAL OF POWER SOURCES(2024)
摘要
The optimization of the electrode manufacturing process is important for upscaling the application of Lithium -Ion Batteries (LIBs) to cater for growing energy demand. LIB manufacturing is important to be optimized because it determines the practical performance of the cells when the latter are being used in applications such as electric vehicles. In this study, we tackled the issue of high-performance electrodes for desired battery applications by proposing a data-driven approach supported by a deterministic machine learning-assisted pipeline for bi-objective optimization of the electrochemical performances. This pipeline allows the inverse design of the process parameters to adopt to manufacture electrodes for energy or power applications. This work is an analogy to our previous work that addressed the optimization of the electrode microstructures for kinetic, ionic, and electronic transport properties improvement. An electrochemical model is fed with the electrode properties characterizing the electrode microstructures generated by manufacturing simulations, and used to simulate the electrochemical performances. Secondly, the resulting dataset was used to train a deterministic model to implement fast optimizations to identify optimal electrodes. Our results suggested a high amount of active material, combined with intermediate values of solid content in the slurry and calendering degree, to achieve the optimal electrodes.
更多查看译文
关键词
Battery cell manufacturing,Bayesian optimization,Machine learning,Electrode,Numerical simulation
AI 理解论文
溯源树
样例
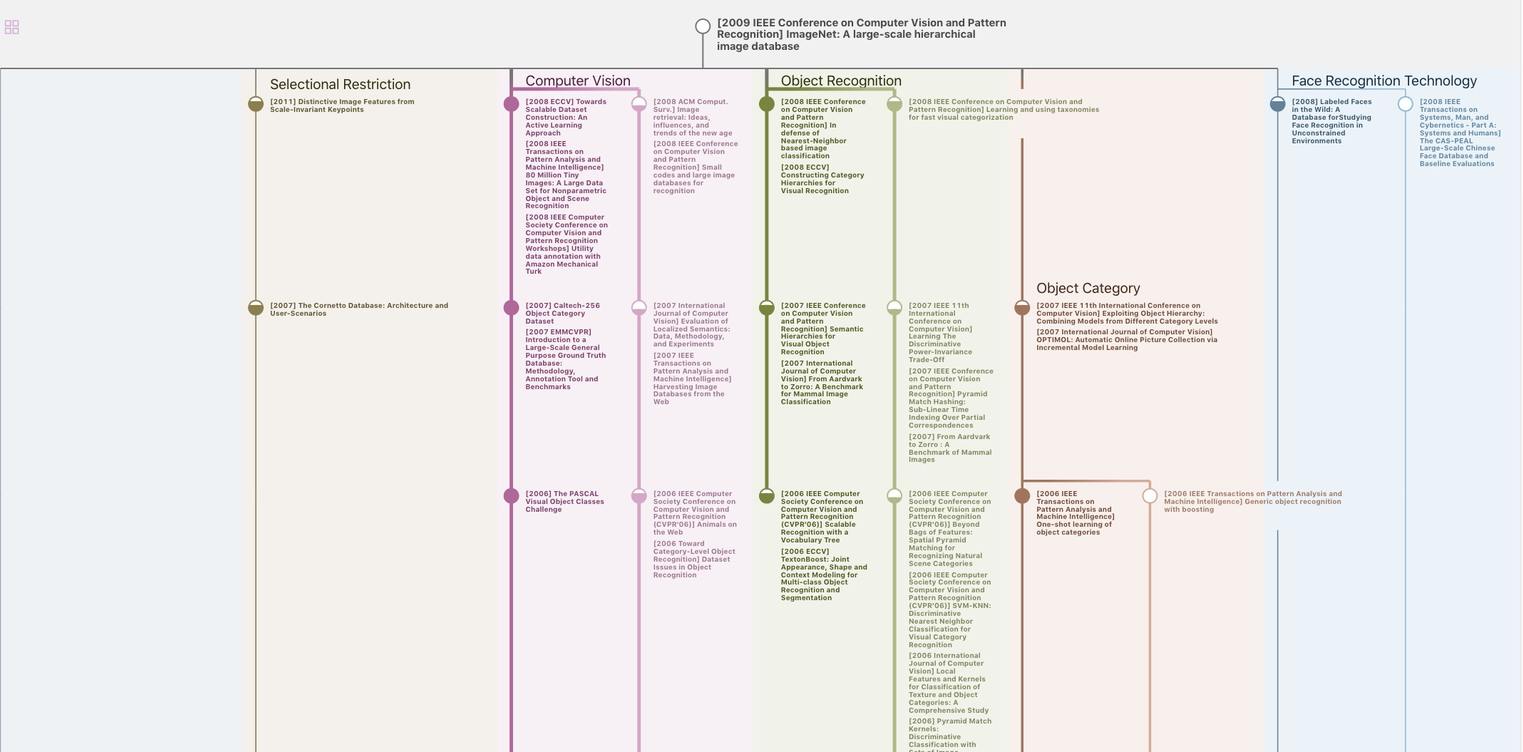
生成溯源树,研究论文发展脉络
Chat Paper
正在生成论文摘要