Semiparametric Shape-restricted Estimators for Nonparametric Regression
arXiv (Cornell University)(2023)
摘要
Estimating the conditional mean function that relates predictive covariates to a response variable of interest is a fundamental task in statistics. In this paper, we propose some general nonparametric regression approaches that are widely applicable under very mild conditions. The method decomposes a function with a Lipschitz continuous $k$-th derivative into a sum of a $(k-1)$-monotone function and a parametric component. We implement well-established shape-restricted estimation procedures (such as isotonic regression) to handle the "nonparametric" components of the true regression function and combine them with a simple sample-splitting procedure to estimate the parametric components. The resulting estimators inherit several favorable properties from the shape-restricted regression estimators. Notably, it is (practically) tuning parameter-free, converges at the minimax rate, and exhibits a locally adaptive rate when the true regression function is "simple". Finally, a series of numerical studies are presented, confirming these theoretical properties.
更多查看译文
关键词
estimators,shape-restricted
AI 理解论文
溯源树
样例
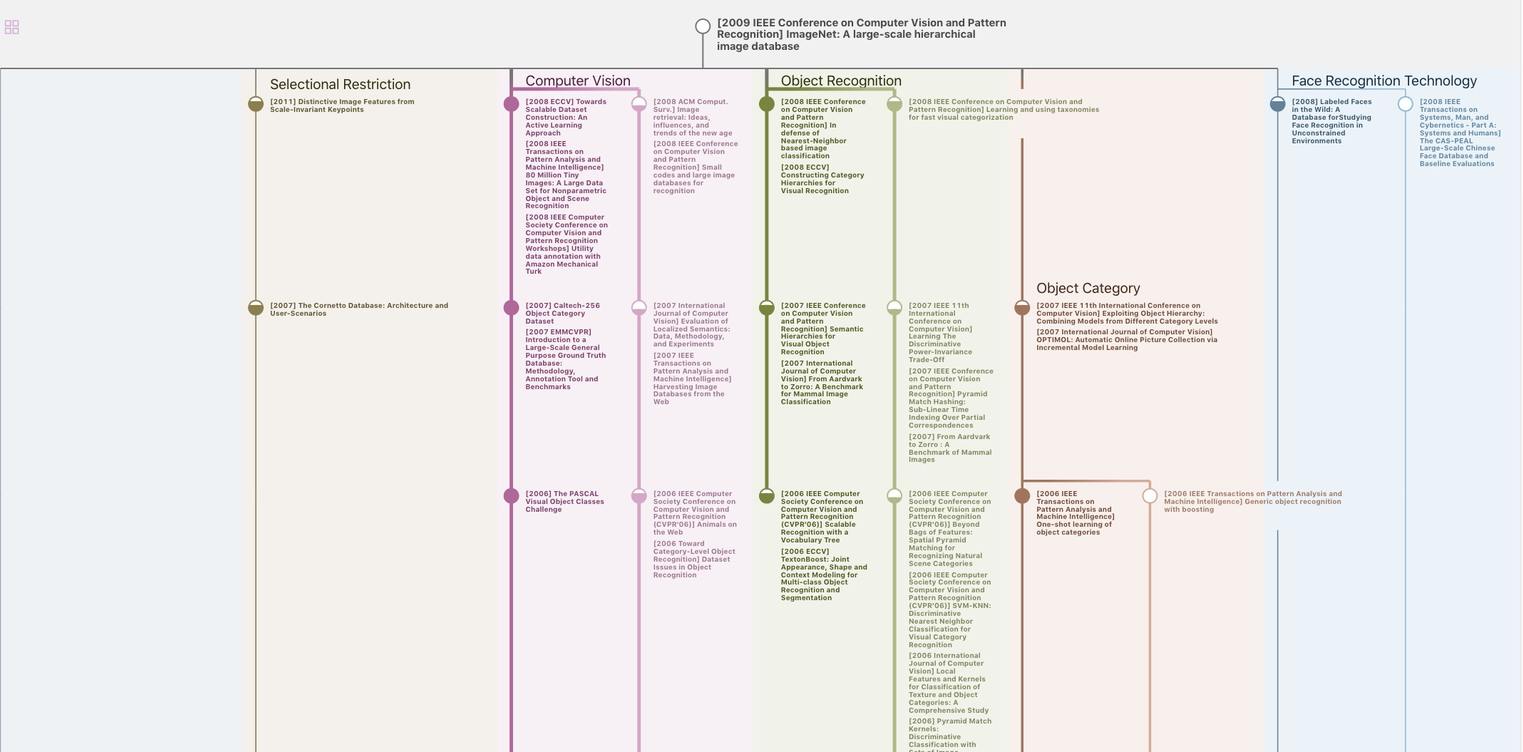
生成溯源树,研究论文发展脉络
Chat Paper
正在生成论文摘要