Learning Koopman Operators with Control Using Bi-level Optimization
2023 62ND IEEE CONFERENCE ON DECISION AND CONTROL, CDC(2023)
摘要
The accurate modeling and control of nonlinear dynamical effects are crucial for numerous robotic systems. The Koopman formalism emerges as a valuable tool for linear control design in nonlinear systems within unknown environments. However, it still remains a challenging task to learn the Koopman operator with control from data, and in particular, the simultaneous identification of the Koopman linear dynamics and the mapping between the physical and Koopman states. Conventionally, the simultaneous learning of the dynamics and mapping is achieved via single-level optimization based on one-step or multi-step discrete-time predictions, but the learned model may lack model robustness, training efficiency, and/or long-term predictive accuracy. This paper presents a bi-level optimization framework that jointly learns the Koopman embedding mapping and Koopman dynamics with exact long-term dynamical constraints. Our formulation allows backpropagation in standard learning framework and the use of state-of-the-art optimizers, yielding more accurate and stable system prediction in long-time horizon over various applications compared to conventional methods.
更多查看译文
AI 理解论文
溯源树
样例
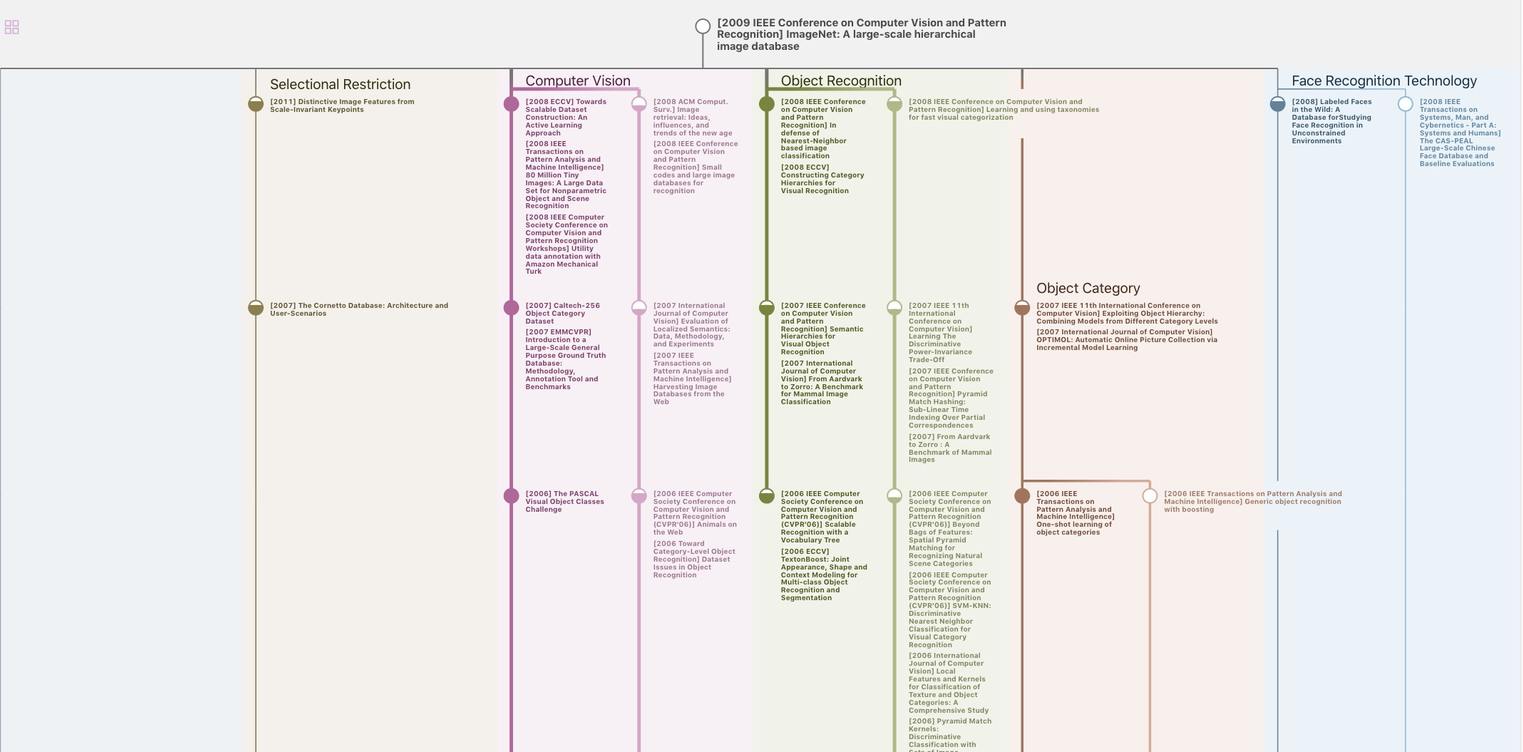
生成溯源树,研究论文发展脉络
Chat Paper
正在生成论文摘要