Tackling Computational Heterogeneity in FL: A Few Theoretical Insights
CoRR(2023)
摘要
The future of machine learning lies in moving data collection along with training to the edge. Federated Learning, for short FL, has been recently proposed to achieve this goal. The principle of this approach is to aggregate models learned over a large number of distributed clients, i.e., resource-constrained mobile devices that collect data from their environment, to obtain a new more general model. The latter is subsequently redistributed to clients for further training. A key feature that distinguishes federated learning from data-center-based distributed training is the inherent heterogeneity. In this work, we introduce and analyse a novel aggregation framework that allows for formalizing and tackling computational heterogeneity in federated optimization, in terms of both heterogeneous data and local updates. Proposed aggregation algorithms are extensively analyzed from a theoretical, and an experimental prospective.
更多查看译文
关键词
computational heterogeneity,fl,few theoretical insights
AI 理解论文
溯源树
样例
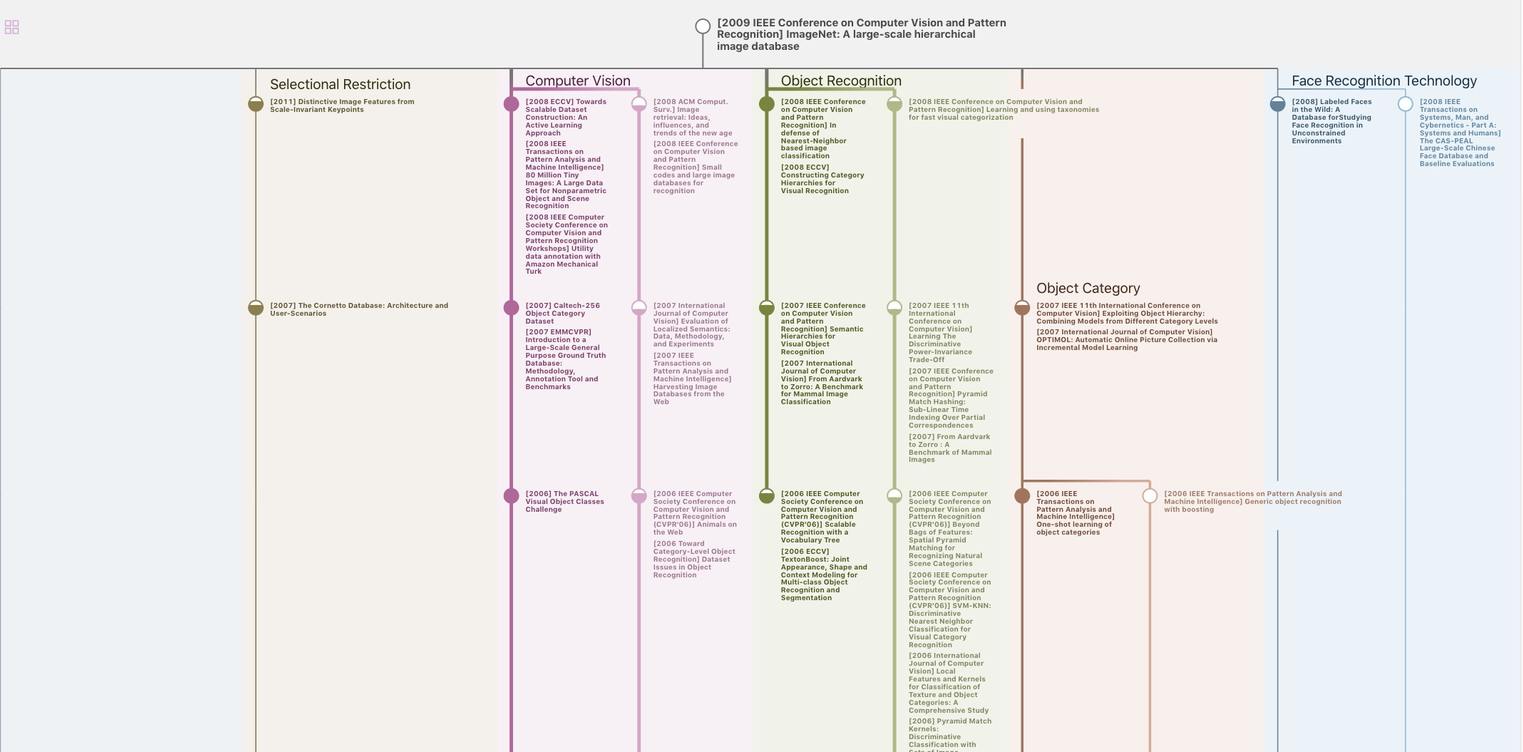
生成溯源树,研究论文发展脉络
Chat Paper
正在生成论文摘要