CFGL-LCR: A Counterfactual Graph Learning Framework for Legal Case Retrieval.
KDD(2023)
Abstract
Legal case retrieval, which aims to find relevant cases based on a short case description, serves as an important part of modern legal systems. Despite the success of existing retrieval methods based on Pretrained Language Models, there are still two issues in legal case retrieval that have not been well considered before. First, existing methods underestimate the semantics associations among legal elements, e.g., law articles and crimes, which played an essential role in legal case retrieval. These methods only adopt the pre-training language model to encode the whole legal case, instead of distinguishing different legal elements in the legal case. They randomly split a legal case into different segments, which may break the completeness of each legal element. Second, due to the difficulty in annotating the relevant labels of similar cases, legal case retrieval inevitably faces the problem of lacking training data. In this paper, we propose a counterfactual graph learning framework for legal case retrieval. Concretely, to overcome the above challenges, we transform the legal case document into a graph and model the semantics of the legal elements through a graph neural network. To alleviate the low resource and learn the causal relationship between the semantics of legal elements and relevance, a counterfactual data generator is designed to augment counterfactual data and enhance the legal case representation. Extensive experiments based on two publicly available legal benchmarks demonstrate that our CFGL-LCR can significantly outperform previous state-of-the-art methods in legal case retrieval.
MoreTranslated text
Key words
legal case retrieval,causal learning,graph neural network
AI Read Science
Must-Reading Tree
Example
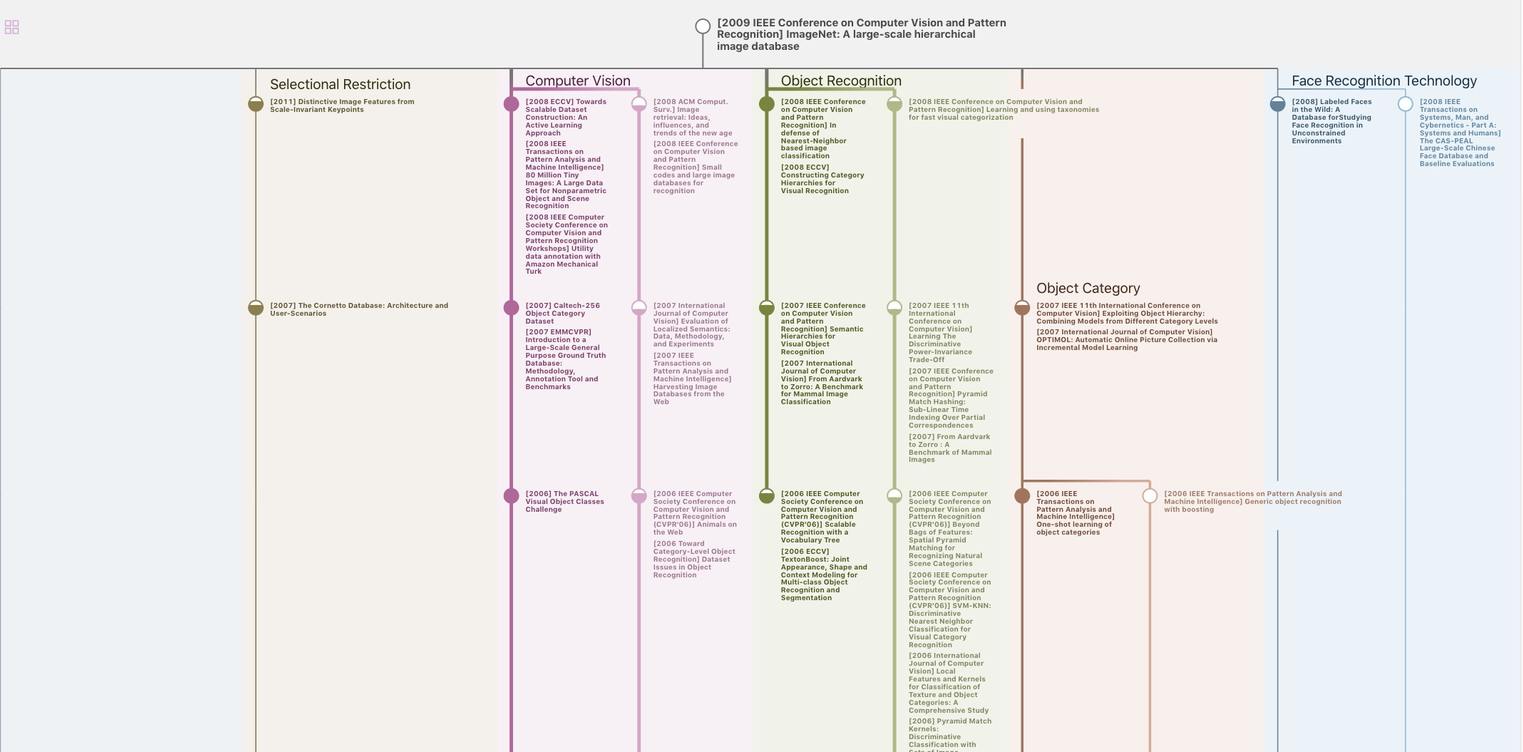
Generate MRT to find the research sequence of this paper
Chat Paper
Summary is being generated by the instructions you defined