Understanding the Semantics of GPS-based Trajectories for Road Closure Detection.
KDD(2023)
摘要
The accurate detection of road closures is of great value for real-time updating of digital maps. The existing methods mainly follow the paradigm of detecting the drastic changes in traffic statistical values (e.g., traffic flow), but they may lead to misidentifying since 1) drastic changes of traffic statistical values are hard to be observed in low-heat roads where the passing vehicles are sparse; 2) statistical values are sensitive to noise (e.g., traffic flow for tiny roads and tunnels is prone to miscounting); and 3) statistical values are naturally delayed, and misidentifying may occur when they have not yet shown significant changes. Surprisingly, since GPS-based trajectories can also exhibit significant abnormal patterns for road closures and have the superiority in fine granularity and timeliness, they can naturally tackle the above challenges. In this paper, we present a novel road closure detection framework based on mining the semantics of trajectories, called T-Closure. We first construct a heterogeneous graph based on the trajectory and the planned route to extract the spatial-topological property of each trajectory, where a node-level auxiliary task is proposed to guide the learning of feature encoders. A multi-view heterogeneous graph neural network (MVH-GNN) with a graph-level auxiliary task is then introduced to capture the semantics of trajectories, where intra-category relevance and inter-category interaction are both considered. Finally, a sequence-level auxiliary task refines the ability of LSTM in modeling the semantic relevance among trajectories while enhancing the robustness of our framework. Experiments on four real-world road closure datasets demonstrate the superiority of T-Closure. Online performance shows that T-Closure can detect 7000+ closure events monthly, with a delay within 1.5 hours.
更多查看译文
关键词
road closure detection,graph neural networks,contrastive learning
AI 理解论文
溯源树
样例
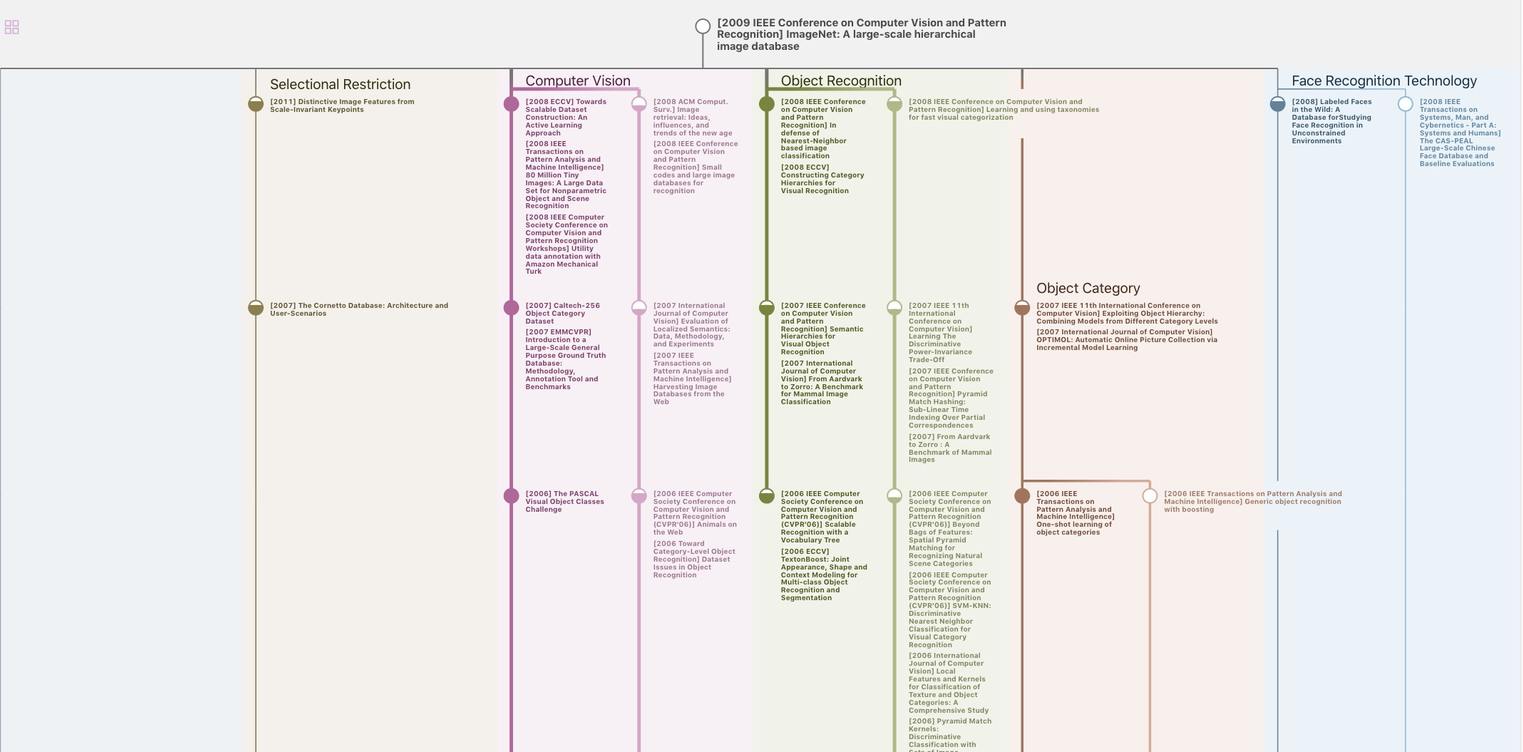
生成溯源树,研究论文发展脉络
Chat Paper
正在生成论文摘要