Fragility Index: A New Approach for Binary Classification.
KDD(2023)
摘要
In binary classification problems, many performance metrics evaluate the probability that some error exceeds a threshold. Nevertheless, they focus more on the probability and fail to capture the magnitude of the error, which evaluates how large this error exceeds the threshold. Capturing the magnitude of error is desired in many applications. For example, in detecting disease and predicting credit default, the magnitude of error illustrates the confidence in making the wrong prediction. We propose a novel metric, the Fragility Index (FI), to evaluate the performance of binary classifiers by capturing the magnitude of the error. FI alleviates the risk of misclassification by penalizing the large error greatly, which is seldom considered by standard metrics. Moreover, to strengthen the generalization ability and handle unseen samples, we adopt the framework of distributionally robust optimization and robust satisficing, which allows us to derive and control the maximum degree of fragility of the classifier when the distribution of samples shifts. We show that FI can be easily calculated and optimized for common probabilistic distance measures. Experiments with real datasets demonstrate the new insights brought by FI and the advantages of classifiers selected under FI, which always improve the robustness and reduce the risk of large errors as compared to classifiers selected by alternative metrics.
更多查看译文
关键词
Binary classification,Robust optimization,Robust satisficing
AI 理解论文
溯源树
样例
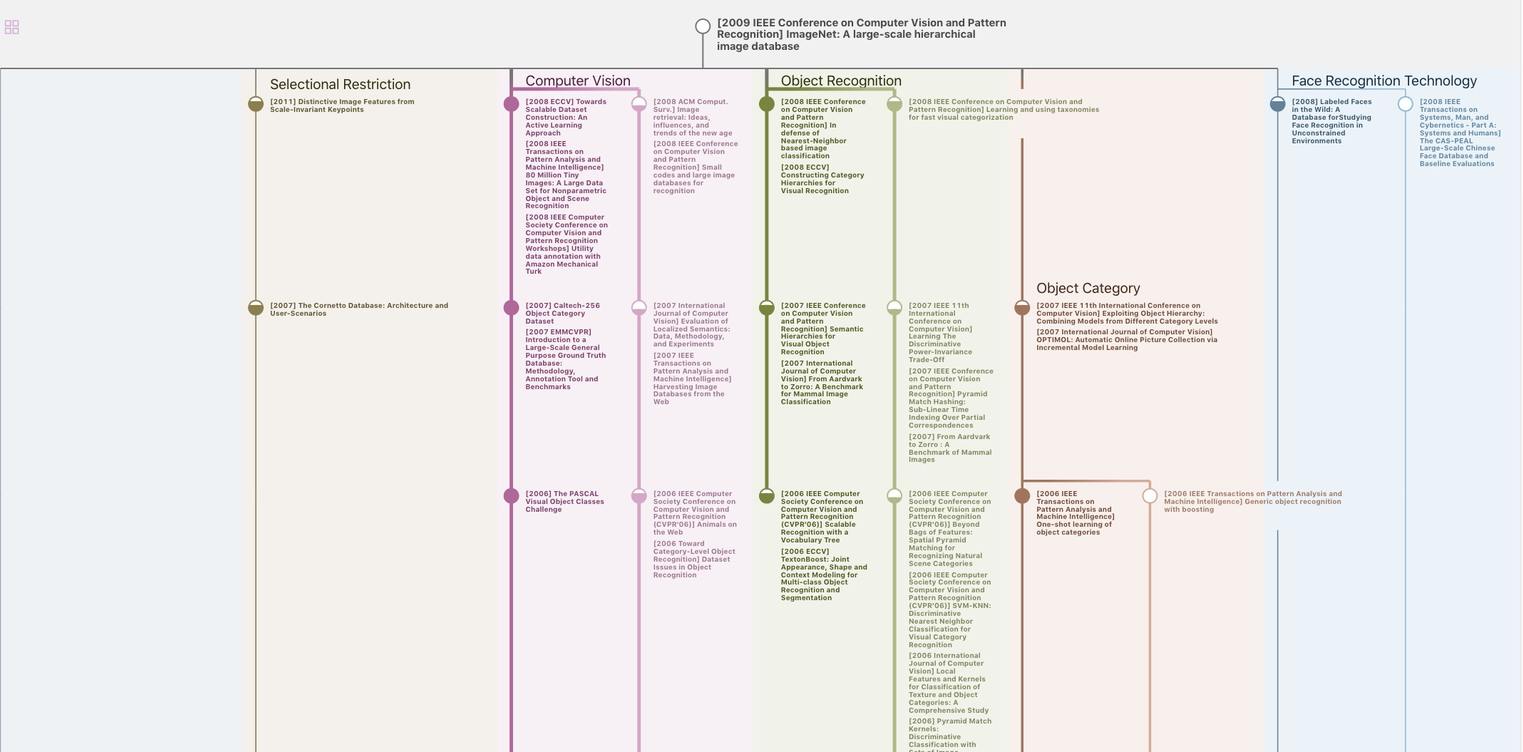
生成溯源树,研究论文发展脉络
Chat Paper
正在生成论文摘要