Counterfactual Video Recommendation for Duration Debiasing.
KDD(2023)
摘要
Duration bias widely exists in video recommendations, where models tend to recommend short videos for the higher ratio of finish playing and thus possibly fail to capture users' true interests. In this paper, we eliminate the duration bias from both data and model. First, based on the extensive data analysis, we observe that play completion rate of videos with the same duration presents a bimodal distribution. Hence, we propose to perform threshold division to construct binary labels as training labels for alleviating the drawback of finish playing labels overly biased towards short videos. Algorithmically, we resort to causal inference, which enables us to inspect causal relationships of video recommendations with a causal graph. We identify that duration has two kinds of effect on prediction: direct and indirect. Duration bias lies in the direct effect, while the indirect effect benefits prediction. To this end, we design a model-agnostic Counterfactual Video Recommendation for Duration Debiasing (CVRDD) framework, which incorporates multi-task learning to estimate different causal effect during training. In the inference phase, we perform counterfactual inference to remove the direct effect of duration for unbiased prediction. We conduct experiments on two industrial datasets, and in addition to achieving highly promising results on traditional top-k recommendation metrics, CVRDD also improves the user watch time.
更多查看译文
关键词
Recommender System,Counterfactual Inference,Debias
AI 理解论文
溯源树
样例
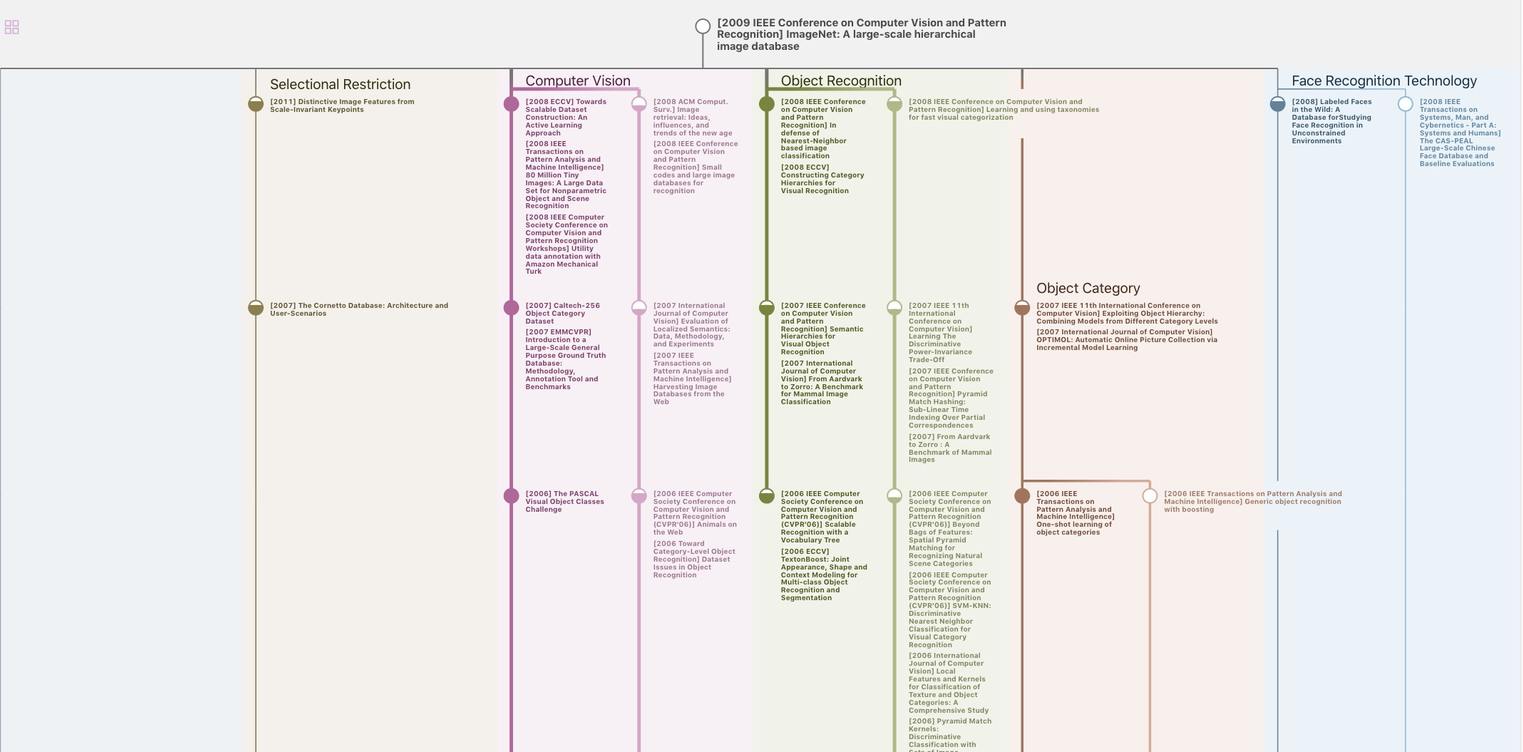
生成溯源树,研究论文发展脉络
Chat Paper
正在生成论文摘要