DisasterNet: Causal Bayesian Networks with Normalizing Flows for Cascading Hazards Estimation from Satellite Imagery.
KDD(2023)
摘要
Sudden-onset hazards like earthquakes often induce cascading secondary hazards (e.g., landslides, liquefaction, debris flows, etc.) and subsequent impacts (e.g., building and infrastructure damage) that cause catastrophic human and economic losses. Rapid and accurate estimates of these hazards and impacts are critical for timely and effective post-disaster responses. Emerging remote sensing techniques provide pre- and post-event satellite images for rapid hazard estimation. However, hazards and damage often co-occur or co-locate with underlying complex cascading geophysical processes, making it challenging to directly differentiate multiple hazards and impacts from satellite imagery using existing single-hazard models. We introduce DisasterNet, a novel family of causal Bayesian networks to model processes that a major hazard triggers cascading hazards and impacts and further jointly induces signal changes in remotely sensed observations. We integrate normalizing flows to effectively model the highly complex causal dependencies in this cascading process. A triplet loss is further designed to leverage prior geophysical knowledge to enhance the identifiability of our highly expressive Bayesian networks. Moreover, a novel stochastic variational inference with normalizing flows is derived to jointly approximate posteriors of multiple unobserved hazards and impacts from noisy remote sensing observations. Integrating with the U.S. Geological Survey (USGS) Prompt Assessment of Global Earthquakes for Response (PAGER) system, our framework is evaluated in recent global earthquake events. Evaluation results show that DisasterNet significantly improves multiple hazard and impact estimation compared to existing USGS products.
更多查看译文
关键词
Bayesian network,Stochastic variational inference,Normalizing flows,Disaster Responses,Satellite Imagery
AI 理解论文
溯源树
样例
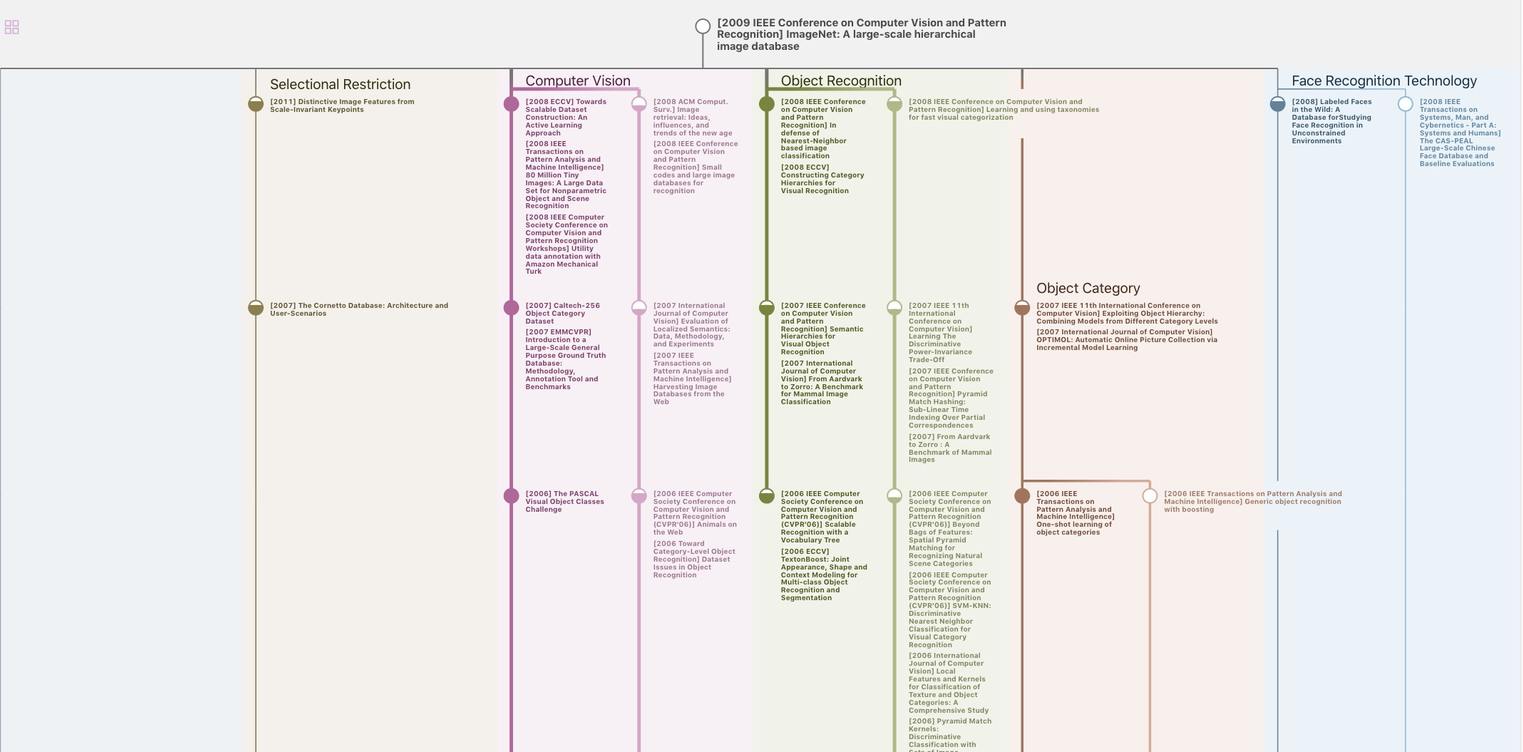
生成溯源树,研究论文发展脉络
Chat Paper
正在生成论文摘要