Delving into Global Dialogue Structures: Structure Planning Augmented Response Selection for Multi-turn Conversations.
KDD(2023)
摘要
Retrieval-based dialogue systems are a crucial component of natural language processing, employing information retrieval techniques to select responses from a predefined pool of candidates. The advent of pre-trained language models (PLMs) has significantly advanced the field, with a prevailing paradigm that involves post-training PLMs on specific dialogue corpora, followed by fine-tuning for the response selection (RS) task. This post-training process aims to capture dialogue-specific features, as most PLMs are originally trained on plain text. However, prior approaches predominantly rely on self-supervised tasks or session-level graph neural networks during post-training, focusing on capturing underlying patterns of coherent dialogues without explicitly refining the global pattern across the entire dialogue corpus. Consequently, the learned knowledge for organizing coherent dialogues remains isolated, heavily reliant on specific contexts. Additionally, interpreting or visualizing the implicit knowledge acquired through self-supervised tasks proves challenging. In this study, we address these limitations by explicitly refining the knowledge required for response selection and structuring it into a coherent global flow, known as "dialogue structure." This structure captures the inter-dependency of utterances and topic shifts, thereby enhancing the response selection task. To achieve this, we propose a novel structure model comprising a state recognizer and a structure planner. This model effectively captures the flow within the utterance history and plans the trajectory of future utterances. Importantly, the structure model operates orthogonally to the retrieval model, enabling seamless integration with existing retrieval models and facilitating collaborative training. Extensive experiments conducted on three benchmark datasets demonstrate the superior performance of our method over a wide range of competitive baselines, establishing a new state-of-the-art in the field.
更多查看译文
关键词
dialogue retrieval,dialogue structure,text mining
AI 理解论文
溯源树
样例
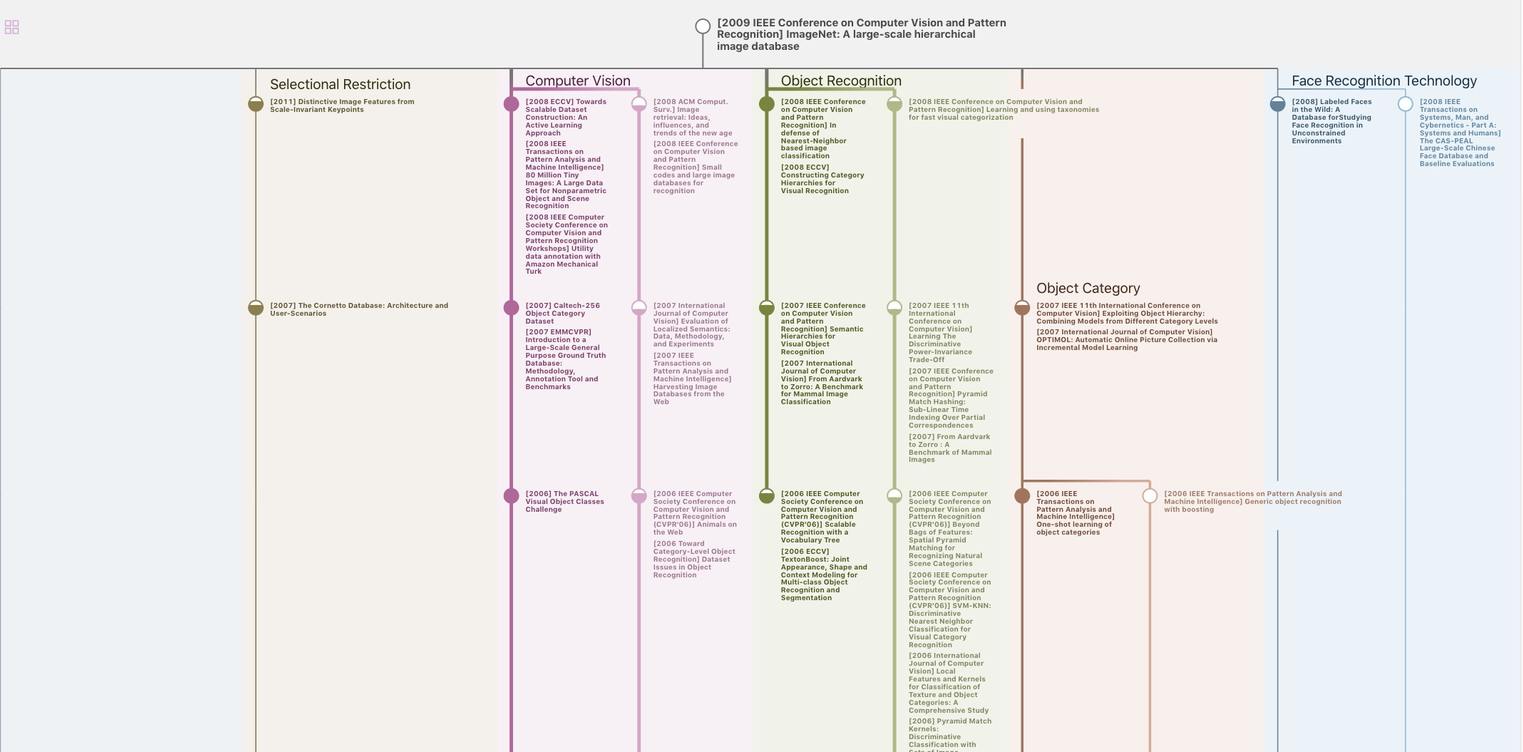
生成溯源树,研究论文发展脉络
Chat Paper
正在生成论文摘要